Quantitative pure-phase object reconstruction under single-shot Fourier measurement via deep learning
Optics and Lasers in Engineering(2021)
摘要
•A deep convolutional neural network framework is developed to establish an accurate propagation model of the Fourier imaging system.•Quantitative phase reconstruction is accomplished from a single-shot far-field intensity-only measurement.•Compared with the well-known Gerchberg-Saxton-Fienup (GSF) algorithm, the phase reconstruction is achieved in a more convenient and faster manner, without the requirement of prior knowledge like support constraints and nonnegativity.•The deep convolutional neural network is considerably robust to the Poisson noise and Speckle noise, and can be trained to additionally function as a denoiser in the phase retrieval process.•Some experimental conditions can be implicitly revealed in the built propagation model, and the spatial resolution is proved to be significantly enhanced and improved via deep learning.
更多查看译文
关键词
Phase retrieval,Fourier measurement,Deep learning,Convolutional neural network
AI 理解论文
溯源树
样例
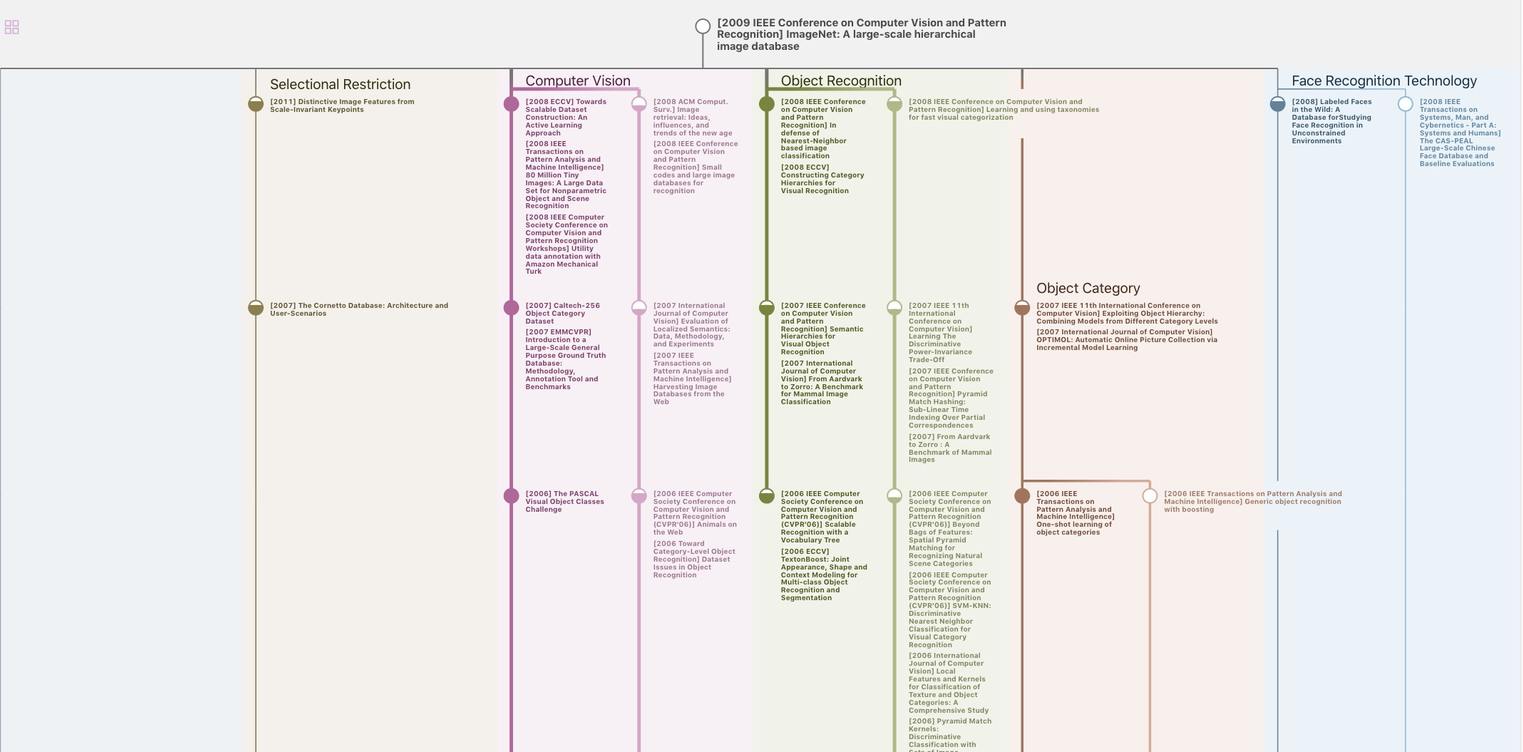
生成溯源树,研究论文发展脉络
Chat Paper
正在生成论文摘要