Efficient unsupervised monocular depth estimation using attention guided generative adversarial network
JOURNAL OF REAL-TIME IMAGE PROCESSING(2021)
摘要
Deep-learning-based approaches to depth estimation are rapidly advancing, offering better performance over traditional computer vision approaches across many domains. However, for many critical applications, cutting-edge deep-learning based approaches require too much computational overhead to be operationally feasible. This is especially true for depth-estimation methods that leverage adversarial learning, such as Generative Adversarial Networks (GANs). In this paper, we propose a computationally efficient GAN for unsupervised monocular depth estimation using factorized convolutions and an attention mechanism. Specifically, we leverage the Extremely Efficient Spatial Pyramid of Depth-wise Dilated Separable Convolutions (EESP) module of ESPNetv2 inside the network, leading to a total reduction of 22.8% , 35.37% , and 31.5% in the number of model parameters, FLOPs, and inference time respectively, as compared to the previous unsupervised GAN approach. Finally, we propose a context-aware attention architecture to generate detail-oriented depth images. We demonstrate superior performance of our proposed model on two benchmark datasets KITTI and Cityscapes. We have also provided more qualitative examples (Fig. 8 ) at the end of this paper.
更多查看译文
关键词
Attention,Efficient GAN,Unsupervised depth estimation,Convolution factorization
AI 理解论文
溯源树
样例
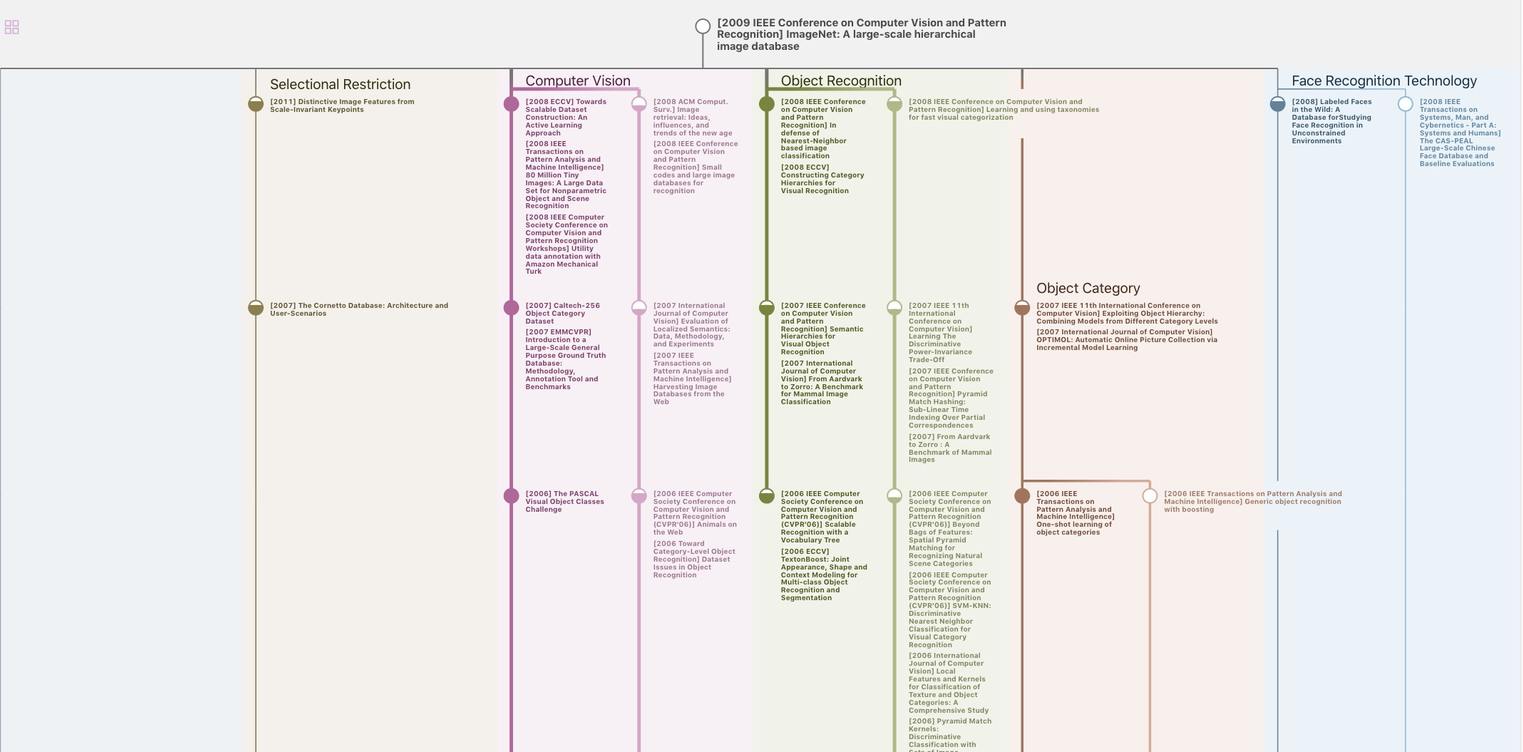
生成溯源树,研究论文发展脉络
Chat Paper
正在生成论文摘要