Comparison of machine learning classifiers: A case study of temperature alarms in a pharmaceutical supply chain
Information Systems(2021)
摘要
Temperature deviations are critical in a pharmaceutical supply chain (SC) due to quality deterioration concerns and resulting health risks. The current solutions ensuring temperature maintenance are either labor-intensive or prone to triggering alarms that require no corrective measures, which, in turn, increase the alarm investigation costs. Machine learning (ML) methods have fared well both in the areas characterized by the execution of repetitive tasks and in the identification of false alarms; however, they have not been applied in the context of temperature monitoring in a pharmaceutical SC. In this paper, we used the real-world data of a large international logistics service provider for the period of 2013–2018 and compared the optimized performance of 10 ML classification methods in the task of false temperature alarm identification. Such additional features as temperature in the location of possible physical handling and average temperature deviation were either externally collected or estimated to enrich the models. In general, gradient boosting achieved the best performance in our evaluations, with an accuracy of 95.9% in comparison with the value of 16.6% demonstrated by the current legacy rule-based system. The feature ranking and sensitivity tests pointed to the strength of the features indicating an absolute temperature deviation and the location of cargo along the SC. The tests simulating model applications on new dissimilar observations showed various performance losses across classifiers, with the best stability retained for a new customer scenario and largest performance decreases for a new temperature range scenario.
更多查看译文
关键词
Cold supply chain,Pharmaceutical,Temperature alarm,Rule-based monitoring,Machine learning,Prediction
AI 理解论文
溯源树
样例
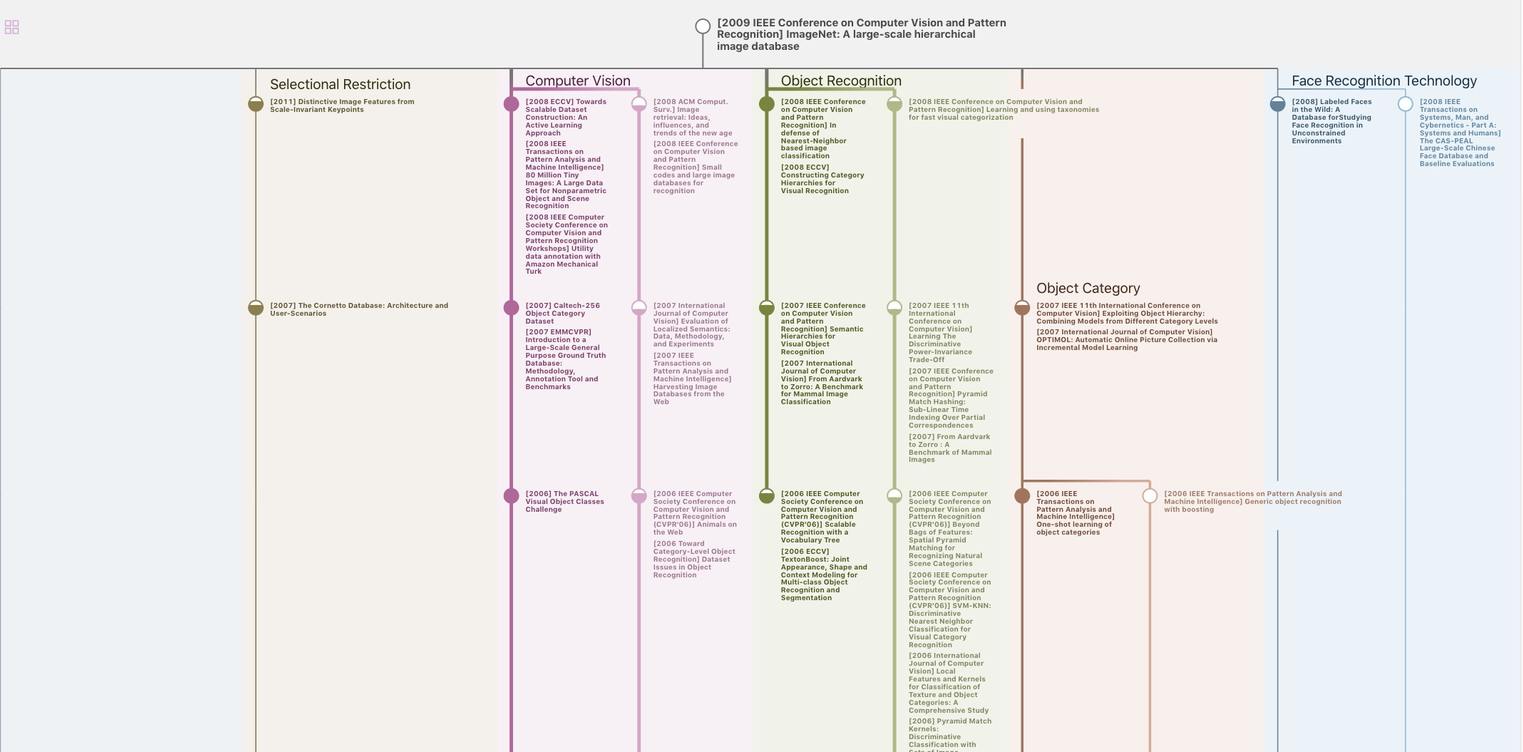
生成溯源树,研究论文发展脉络
Chat Paper
正在生成论文摘要