A power and thermal-aware virtual machine management framework based on machine learning
CLUSTER COMPUTING-THE JOURNAL OF NETWORKS SOFTWARE TOOLS AND APPLICATIONS(2021)
摘要
Energy consumption in data centers grows rapidly in recent years. As a widely-applied energy-efficient method, workload consolidation also has its own limitations that may bring some negative effects, such as performance degradation, QoS violation, localized hotspots and so on, which is especially true when optimal objectives are inherently conflict. In this paper, we present a power and thermal-aware VM management framework called PTM-ML, which relies on machine learning technique to find optimal host configuration based on workload characteristics and cooling system’s working state. Based on such an optimal host configuration, it then makes VM migration and consolidation decisions by enforcing an efficient load-balancing policy, with aiming at achieving a better trade-off between energy efficiency and performance. The prototype of PTM-ML framework is deployed and evaluated in a real-world cloud data center. Extensive experiments are conducted by using different workload traces with distinctive characteristics, and the results are compared with four similar approaches in terms of total energy consumption, real-time power consumption, average latency and etc. Experimental results show that the proposed PTM-ML outperforms the existing approaches in terms of multiple metrics, and it also exhibits better robustness and adaptability in presence of dynamic workloads.
更多查看译文
关键词
Cloud computing, Virtual machine, Data center, Energy efficiency, Thermal management
AI 理解论文
溯源树
样例
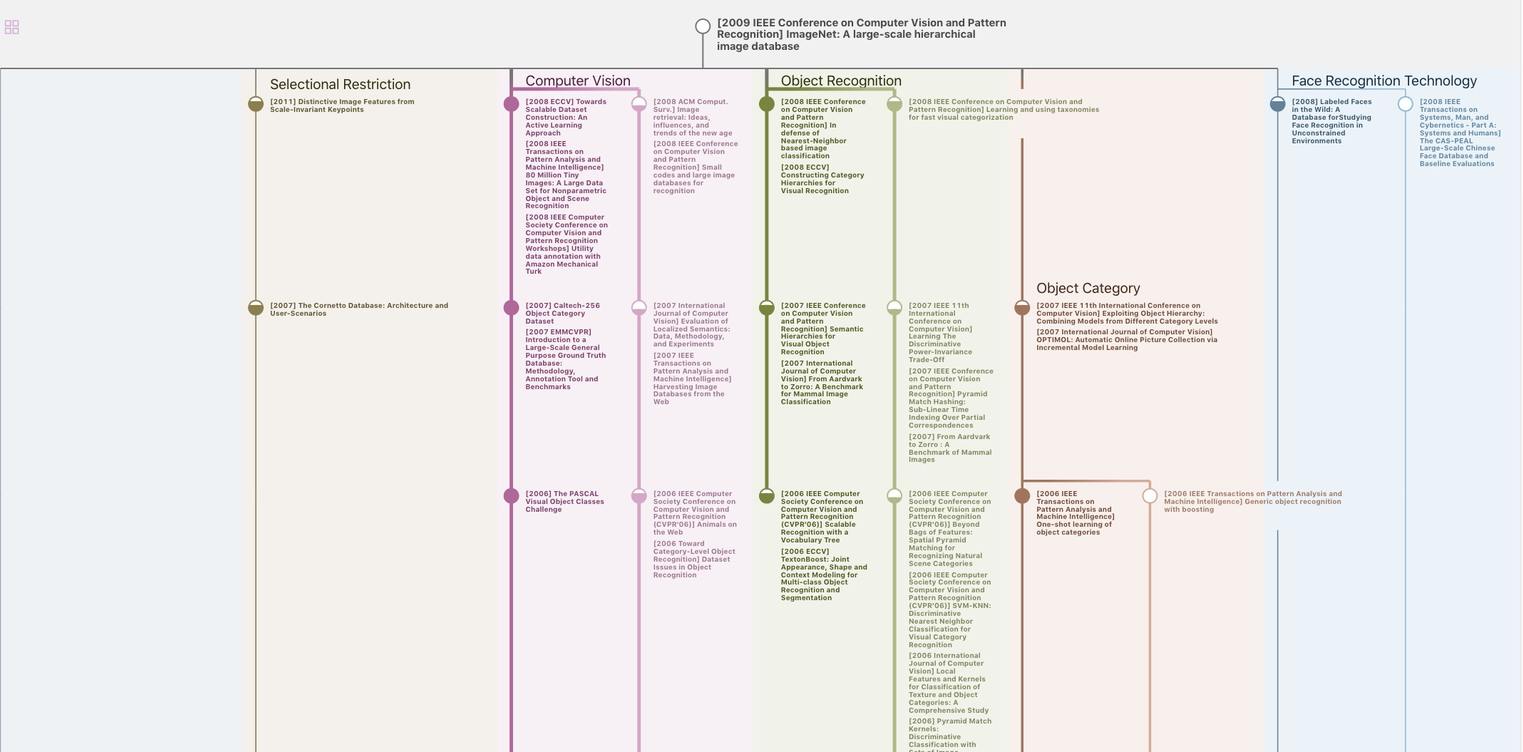
生成溯源树,研究论文发展脉络
Chat Paper
正在生成论文摘要