Classification Of Wines Using Principal Component Analysis
JOURNAL OF WINE ECONOMICS(2021)
摘要
Classification of wines with a large number of correlated covariates may lead to classification results that are difficult to interpret. In this study, we use a publicly available dataset on wines from three known cultivars, where there are 13 highly correlated variables measuring chemical compounds of wines. The goal is to produce an efficient classifier with straightforward interpretation to shed light on the important features of wines in the classification. To achieve the goal, we incorporate principal component analysis (PCA) in the k-nearest neighbor (kNN) classification to deal with the serious multicollinearity among the explanatory variables. PCA can identify the underlying dominant features and provide a more succinct and straightforward summary over the correlated covariates. The study shows that kNN combined with PCA yields a much simpler and interpretable classifier that has comparable performance with kNN based on all the 13 variables. The appropriate number of principal components is chosen to strike a balance between predictive accuracy and simplicity of interpretation. Our final classifier is based on only two principal components, which can be interpreted as the strength of taste and level of alcohol and fermentation in wines, respectively.
更多查看译文
关键词
cross-validation, k-nearest neighbor classification, principal component analysis
AI 理解论文
溯源树
样例
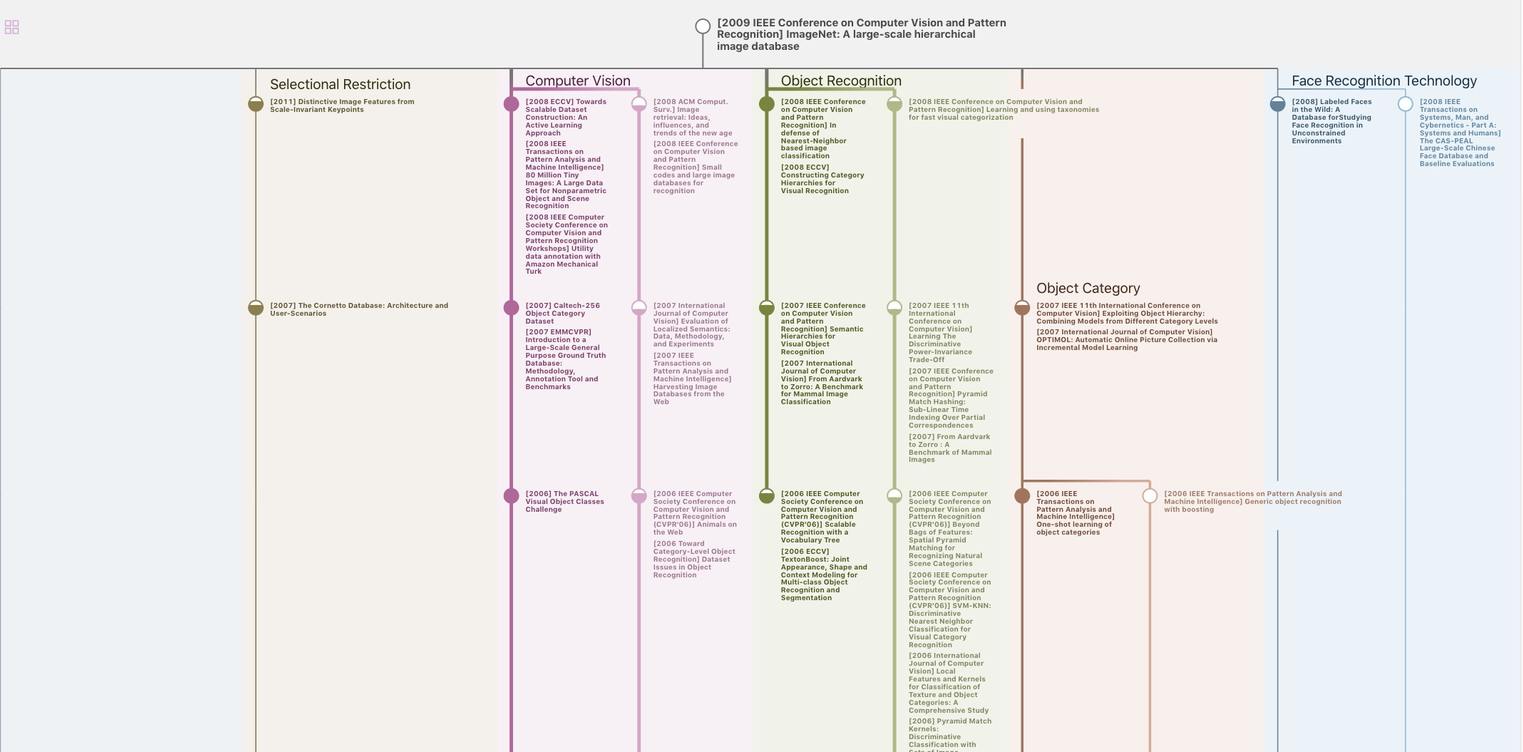
生成溯源树,研究论文发展脉络
Chat Paper
正在生成论文摘要