Zero-shot policy generation in lifelong reinforcement learning.
Neurocomputing(2021)
摘要
Lifelong reinforcement learning (LRL) is an important approach to achieve continual lifelong learning of multiple reinforcement learning tasks. The two major methods used in LRL are task decomposition and policy knowledge extraction. Policy knowledge extraction method in LRL can share knowledge for tasks in different task domains and for tasks in the same task domain with different system environmental coefficients. However, the generalization ability of policy knowledge extraction method is limited on learned tasks rather than learned task domains. In this paper, we propose a cross-domain lifelong reinforcement learning algorithm with zero-shot policy generation ability (CDLRL-ZPG) to improve generalization ability of policy knowledge extraction method from learned tasks to learned task domains. In experiments, we evaluated CDLRL-ZPG performance on four task domains. And our results show that the proposed algorithm can directly generate satisfactory results without needing a trial and error learning process to achieve zero-shot learning in general.
更多查看译文
关键词
Lifelong reinforcement learning,Generalization policy,Task domain
AI 理解论文
溯源树
样例
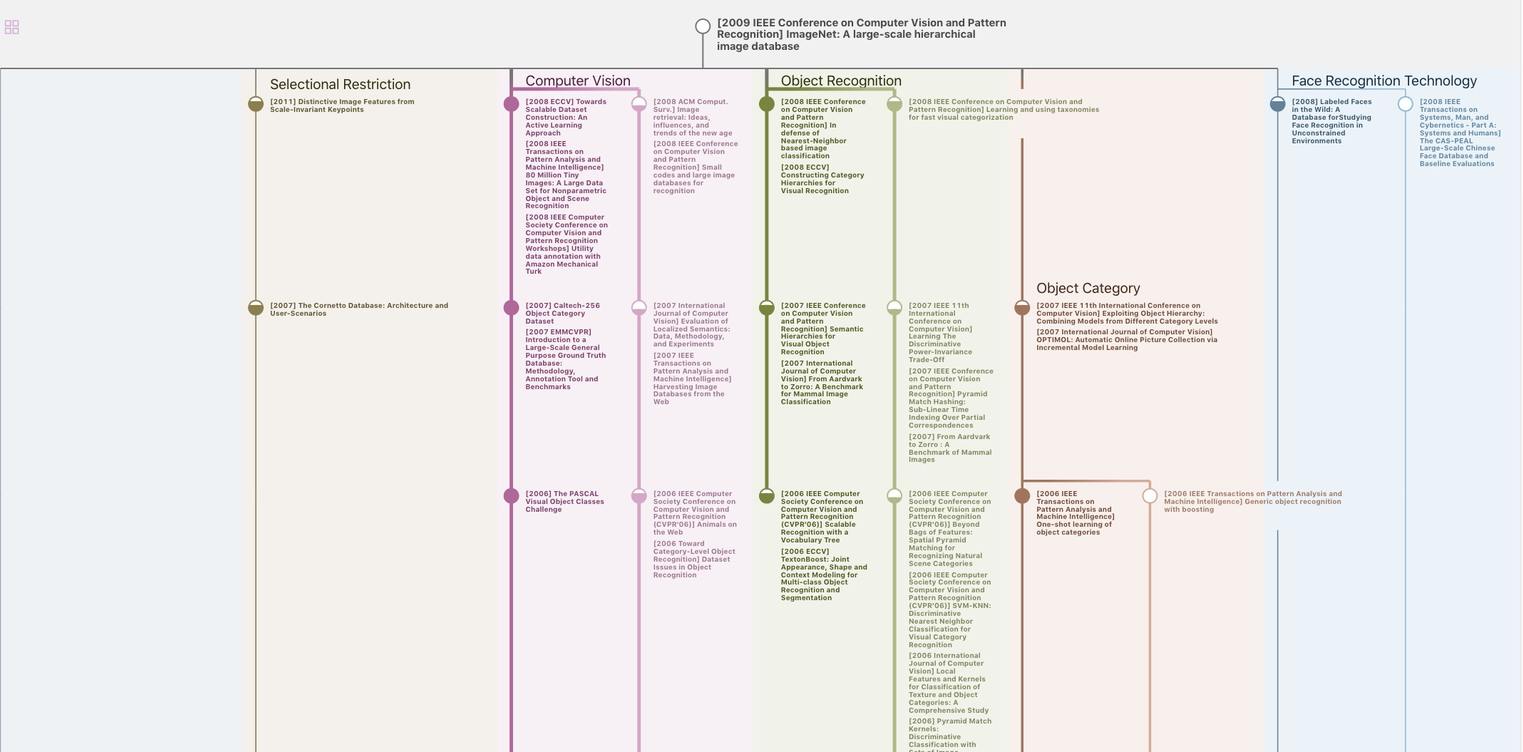
生成溯源树,研究论文发展脉络
Chat Paper
正在生成论文摘要