Study On Dimensionality Reduction For Sea-Level Variations By Using Altimetry Data Around The East Asia Coasts
KOREAN JOURNAL OF REMOTE SENSING(2021)
摘要
Recently, as data mining and artificial neural network techniques are developed, analyzing large amounts of data is proposed to reduce the dimension of the data. In general, empirical orthogonal function (EOF) used to reduce the dimension in the ocean data and recently, Self-organizing maps (SOM) algorithm have been investigated to apply to the ocean field. In this study, both algorithms used the monthly Sea level anomaly (SLA) data from 1993 to 2018 around the East Asia Coasts. There was dominated by the influence of the Kuroshio Extension and eddy kinetic energy. It was able to find the maximum amount of variance of EOF modes. SOM algorithm summarized the characteristic of spatial distributions and periods in EOF mode 1 and 2. It was useful to find the change of SLA variable through the movement of nodes. Node 1 and 5 appeared in the early 2000s and the early 2010s when the sea level was high. On the other hand, node 2 and 6 appeared in the late 1990s and the late 2000s, when the sea level was relatively low. Therefore, it is considered that the application of the SOM algorithm around the East Asia Coasts is well distinguished. In addition, SOM results processed by SLA data, it is able to apply the other climate data to explain more clearly SLA variation mechanisms.
更多查看译文
关键词
Altimetry, Sea level change, Empirical Orthogonal Function (EOF), Self-organizing Maps (SOM)
AI 理解论文
溯源树
样例
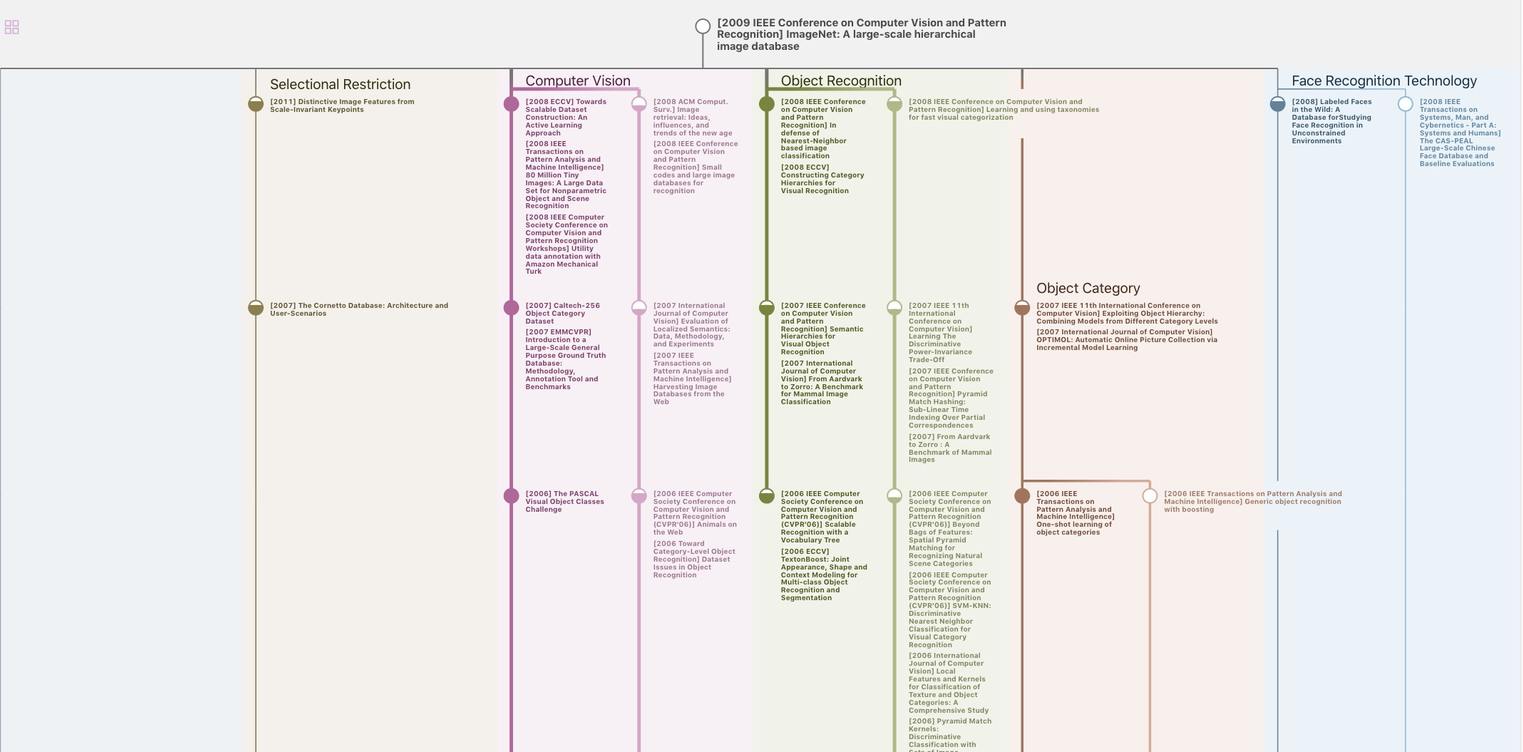
生成溯源树,研究论文发展脉络
Chat Paper
正在生成论文摘要