Estimating Local-Scale Domestic Electricity Energy Consumption Using Demographic, Nighttime Light Imagery And Twitter Data
ENERGY(2021)
摘要
To implement a new mixed approach for electricity energy consumption estimates, this study aimed to estimate country-wide local-scale electricity consumption by combining demographic, remote sensing, and social sensing data. Specifically, England-wide local-scale electricity energy consumption, including domestic and non-domestic ones, was estimated based on population in combination with nighttime light intensity or/and tweet volume. Moreover, to improve the explanatory power of statistical regression models, this study applied a newly developed spatial regression model (i.e., the 'random effects eigenvector spatial filtering' model) to the estimation of electricity energy consumption in comparison with conventional spatial regression models used in relevant studies. The spatial regression model used was further compared with machine learning and deep learning models (i.e., random forest and long shortterm memory models). The empirical results uncover that: 1) the electricity energy consumption can be best explained by population in combination with both the nighttime light intensity and tweet volume; 2) the domestic electricity energy consumption can be better explained than its non-domestic counterpart; 3) the 'random effects eigenvector spatial filtering' models appear to outperform the conventional spatial regression models; and 4) the performance of the 'random effects eigenvector spatial filtering' models is similar to that of the random forest models and is lower than that of the long shortterm memory models.(c) 2021 Elsevier Ltd. All rights reserved.
更多查看译文
关键词
Electricity energy consumption, Twitter data, Nighttime light imagery, SNPP-VIIRS, Random effects eigenvector spatial filtering
AI 理解论文
溯源树
样例
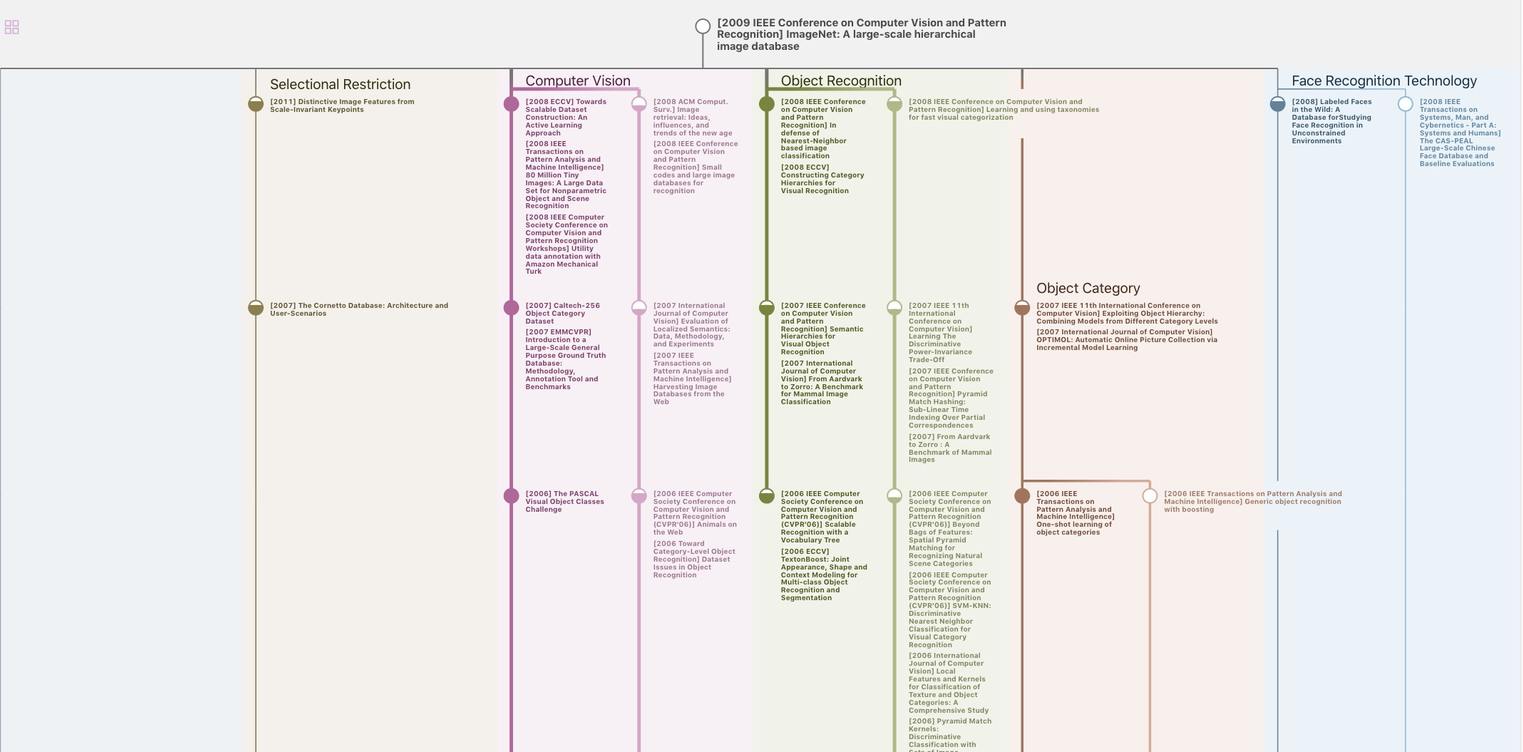
生成溯源树,研究论文发展脉络
Chat Paper
正在生成论文摘要