Unifying inference for semiparametric regression
ECONOMETRICS JOURNAL(2021)
摘要
In the literature, a discrepancy in the limiting distributions of least square estimators between the stationary and nonstationary cases exists in various regression models with different persistence level regressors. This hinders further statistical inference since one has to decide which distribution should be used next. In this paper, we develop a semiparametric partially linear regression model with stationary and nonstationary regressors to attenuate this difficulty, and propose a unifying inference procedure for the coefficients. To be specific, we propose a profile weighted estimation equation method that facilitates the unifying inference. The proposed method is applied to the predictive regressions of stock returns, and an empirical likelihood procedure is developed to test the predictability. It is shown that the Wilks theorem holds for the empirical likelihood ratio regardless of predictors being stationary or not, which provides a unifying method for constructing confidence regions of the coefficients of state variables. Simulations show that the proposed method works well and has favourable finite sample performance over some existing approaches. An empirical application examining the predictability of equity returns highlights the value of our methodology.
更多查看译文
关键词
Empirical likelihood, semiparametric regression, integrated process, profile weighted estimation, uniform inference
AI 理解论文
溯源树
样例
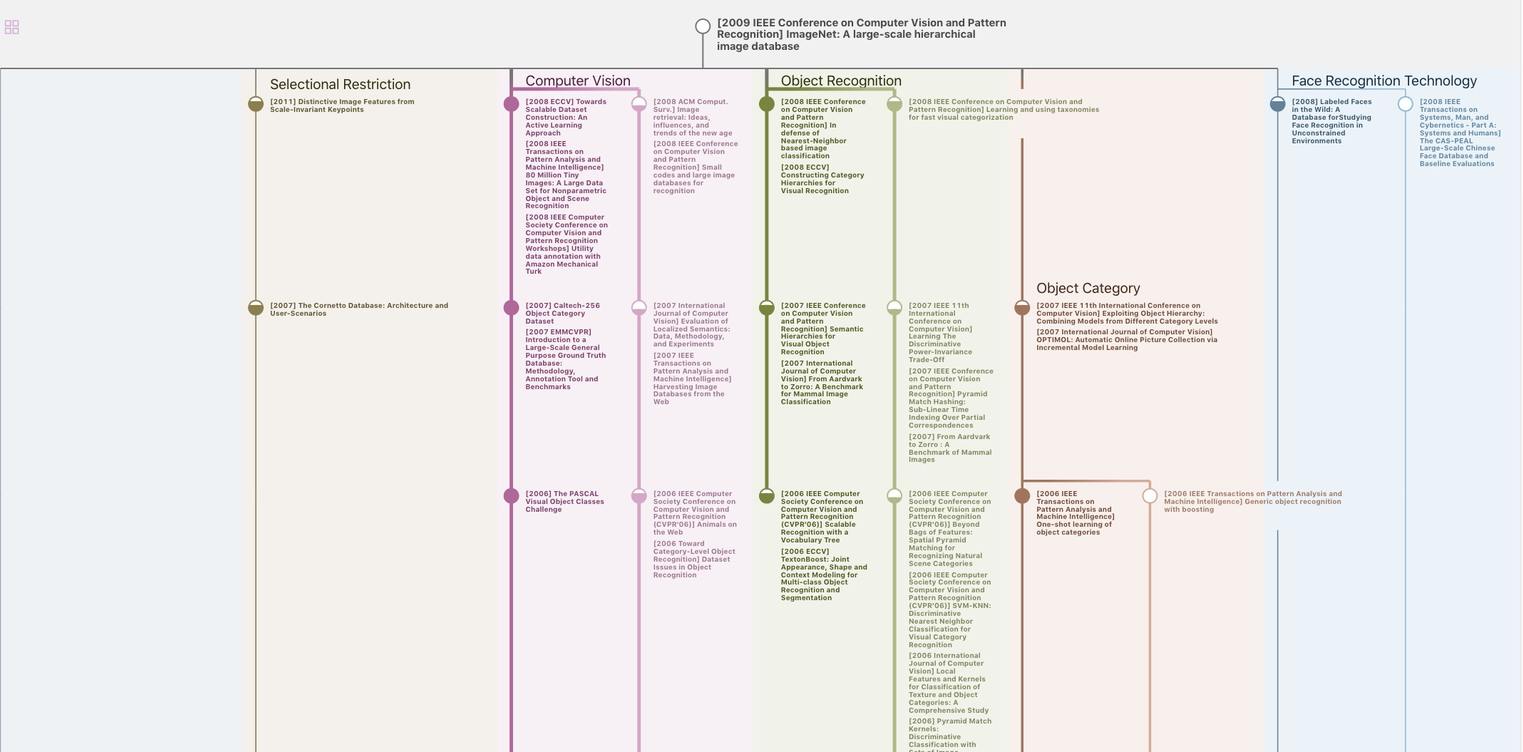
生成溯源树,研究论文发展脉络
Chat Paper
正在生成论文摘要