On The Influence Maximization Problem And The Percolation Phase Transition
PHYSICA A-STATISTICAL MECHANICS AND ITS APPLICATIONS(2021)
摘要
We analyze the problem of network influence maximization in the uniform independent cascade model: Given a network with N nodes and a probability p for a node to contaminate a neighbor, find a set of k initially contaminated nodes that maximizes the expected number of eventually contaminated nodes. This problem is of interest theoretically and for many applications in social networks. Unfortunately, it is a NP-hard problem. Using Percolation Theory, we show that in practice the problem is hard only in a vanishing neighborhood of a critical value p = p(c). For p > p(c) there exists a "Giant Cluster'' of order N, that is easily found in finite time. For p < p(c) the clusters are finite, and one can find one of them in finite time (independent of N). Thus, for most social networks in the real world the solution time does not scale with the size of the network. (C) 2021 Elsevier B.V. All rights reserved.
更多查看译文
关键词
Networks, Influence maximization, Percolation, Empirical hardness
AI 理解论文
溯源树
样例
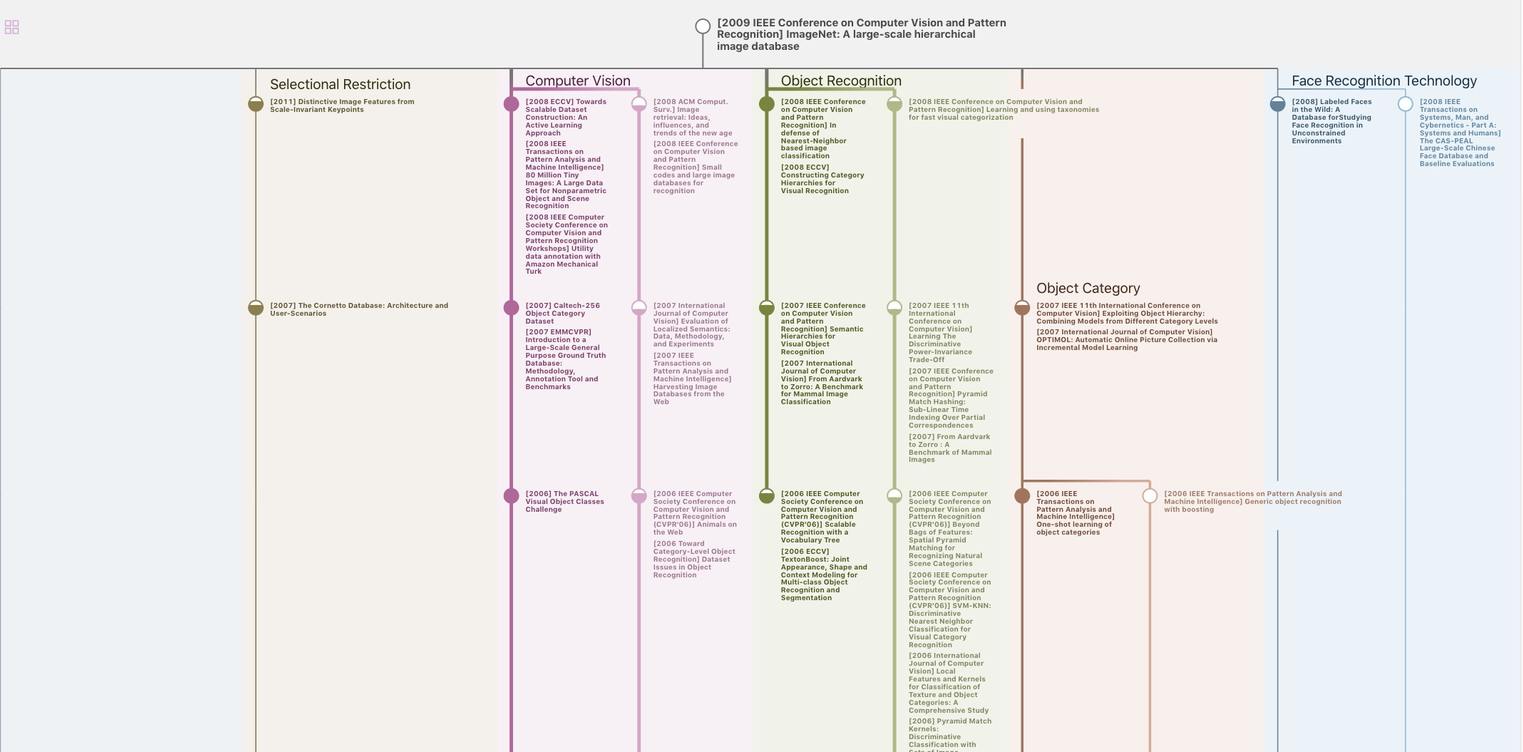
生成溯源树,研究论文发展脉络
Chat Paper
正在生成论文摘要