Point Transformer for Shape Classification and Retrieval of Urban Roof Point Clouds
IEEE GEOSCIENCE AND REMOTE SENSING LETTERS(2022)
摘要
The success of deep learning methods led to significant breakthroughs in 3-D point cloud processing tasks with applications in remote sensing. Existing methods utilize convolutions that have some limitations, as they assume a uniform input distribution and cannot learn long-range dependences. Recent works have shown that adding attention in conjunction with these methods improves performance. This raises a question: can attention layers completely replace convolutions? This letter proposes a fully attentional model--Point Transformer (PT) for deriving a rich point cloud representation. The model's shape classification and retrieval performance are evaluated on a large-scale urban data set--RoofN3D and a standard benchmark data set ModelNet40. Extensive experiments are conducted to test the model's robustness to unseen point corruptions for analyzing its effectiveness on real data sets. The proposed method outperforms other state-of-the-art models in the RoofN3D data set, gives competitive results in the ModelNet40 benchmark, and shows high robustness to various unseen point corruptions. Furthermore, the model is highly memory and space-efficient when compared to other methods.
更多查看译文
关键词
Three-dimensional displays, Shape, Data models, Routing, Robustness, Feature extraction, Benchmark testing, Mean average precision (MAP), Point Transformer (PT), self-attention, shape classification, shape retrieval
AI 理解论文
溯源树
样例
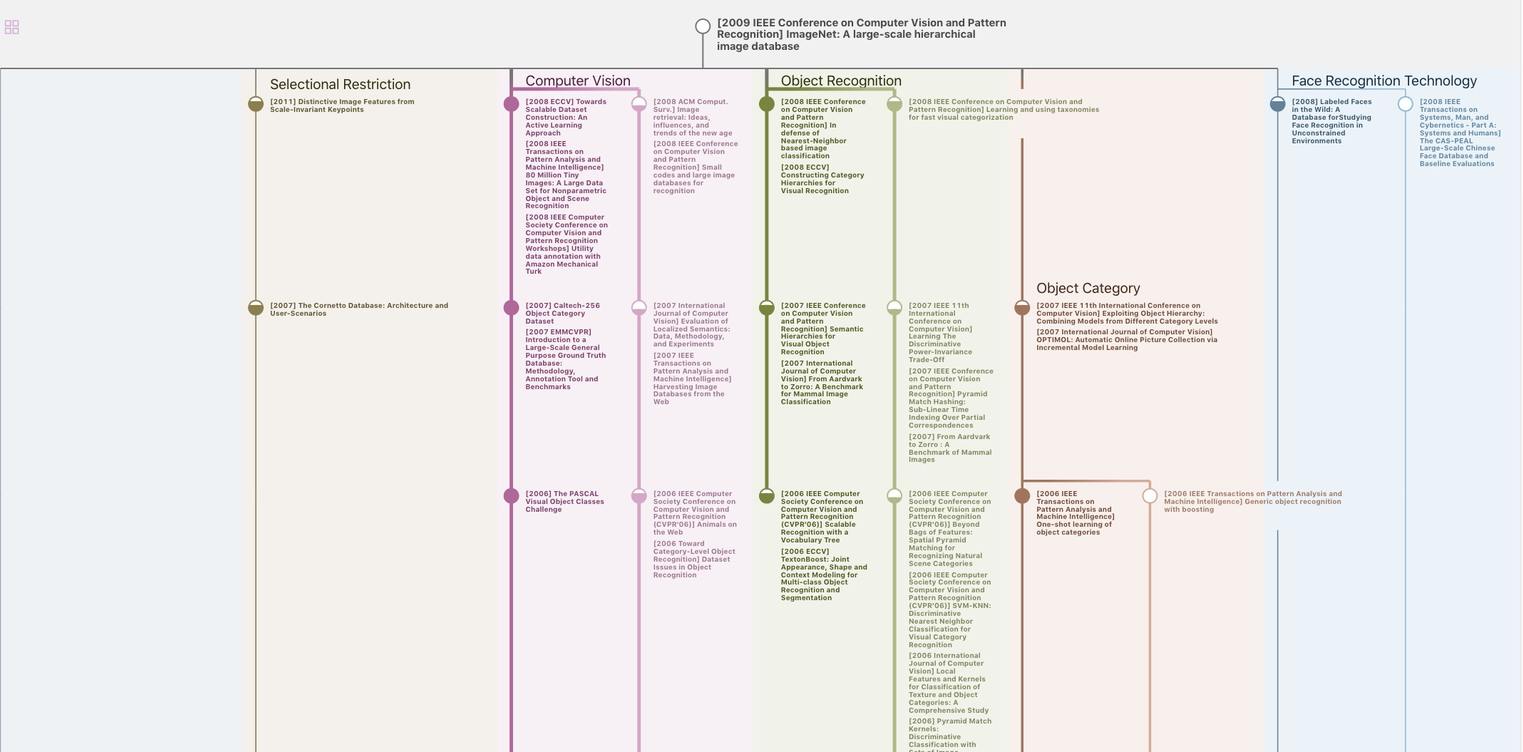
生成溯源树,研究论文发展脉络
Chat Paper
正在生成论文摘要