Reinforcement learning in optimizing forest management
CANADIAN JOURNAL OF FOREST RESEARCH(2021)
摘要
We solve a stochastic high-dimensional optimal harvesting problem by using reinforcement learning algorithms developed for agents who learn an optimal policy in a sequential decision process through repeated experience. This approach produces optimal solutions without discretization of state and control variables. Our stand-level model includes mixed species, tree size structure, optimal harvest timing, choice between rotation and continuous cover forestry, stochasticity in stand growth, and stochasticity in the occurrence of natural disasters. The optimal solution or policy maps the system state to the set of actions, i.e., clear-cutting, thinning, or no harvest decisions as well as the intensity of thinning over tree species and size classes. The algorithm repeats the solutions for deterministic problems computed earlier with time-consuming methods. Optimal policy describes harvesting choices from any initial state and reveals how the initial thinning versus clear-cutting choice depends on the economic and ecological factors. Stochasticity in stand growth increases the diversity of species composition. Despite the high variability in natural regeneration, the optimal policy closely satisfies the certainty equivalence principle. The effect of natural disasters is similar to an increase in the interest rate, but in contrast to earlier results, this tends to change the management regime from rotation forestry to continuous cover management.
更多查看译文
关键词
reinforcement learning, forestry, economics, stochasticity, continuous cover forestry, uneven-aged forestry, optimal rotation, mixed species, optimal harvesting
AI 理解论文
溯源树
样例
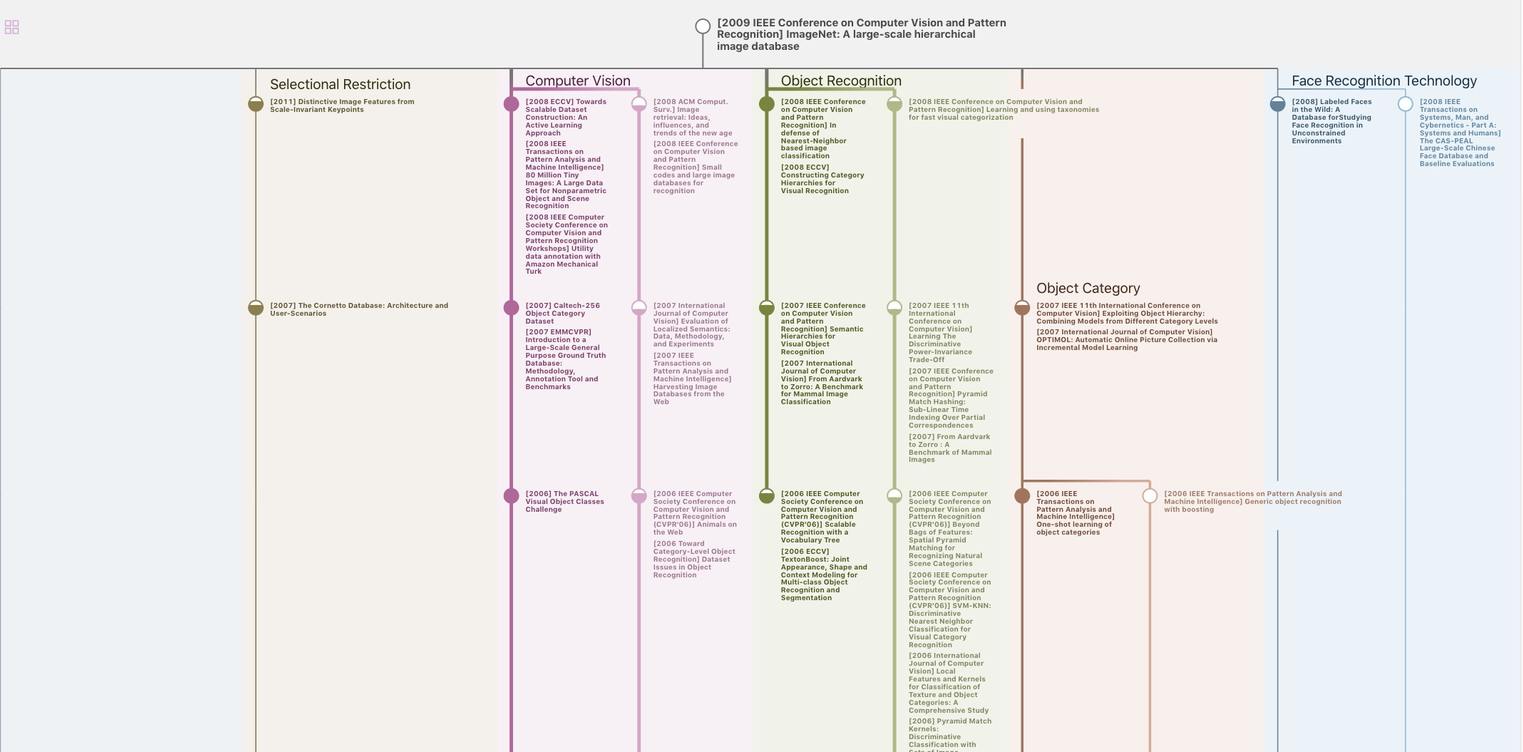
生成溯源树,研究论文发展脉络
Chat Paper
正在生成论文摘要