Forage yield and quality estimation by means of UAV and hyperspectral imaging
PRECISION AGRICULTURE(2021)
摘要
This study investigated the potential of in-season airborne hyperspectral imaging for the calibration of robust forage yield and quality estimation models. An unmanned aerial vehicle (UAV) and a hyperspectral imager were used to capture canopy reflections of a grass-legume mixture in the range of 450 nm to 800 nm. Measurements were performed over two years at two locations in Southeast and Central Norway. All images were subject to radiometric and geometric corrections before being processed to ortho-images, carrying canopy reflectance information. The data (n = 707) was split in two, using half the data for model calibration and the remaining half for validation. Several powered partial least squares regression (PPLSR) models were fitted to the reflectance data to estimate fresh (FM) and dry matter (DM) yields, as well as crude protein (CP), dry matter digestibility (DMD), neutral detergent fibre (NDF), and indigestible neutral detergent fibre (iNDF) content. Prediction performance of these models was compared with the prediction performance of simple linear regression (SLR) models, which were based on selected vegetation indices and plant height. The highest prediction accuracies for general models, based on the pooled data, were achieved by means of PPLSR, with relative root-mean-square errors of validation of 14.2% (2550 kg FM ha −1 ), 15.2% (555 kg DM ha −1 ), 11.7% (1.32 g CP 100 g −1 DM), 2.4% (1.71 g DMD 100 g −1 DM), 4.8% (2.72 g NDF 100 g −1 DM), and 12.8% (1.32 g iNDF 100 g −1 DM) for the prediction of FM, DM, CP, DMD, NDF, and iNDF content, respectively. None of the tested SLR models achieved acceptable prediction accuracies.
更多查看译文
关键词
UAV, Hyperspectral imaging, Multivariate statistics, Forage, Yield, Quality
AI 理解论文
溯源树
样例
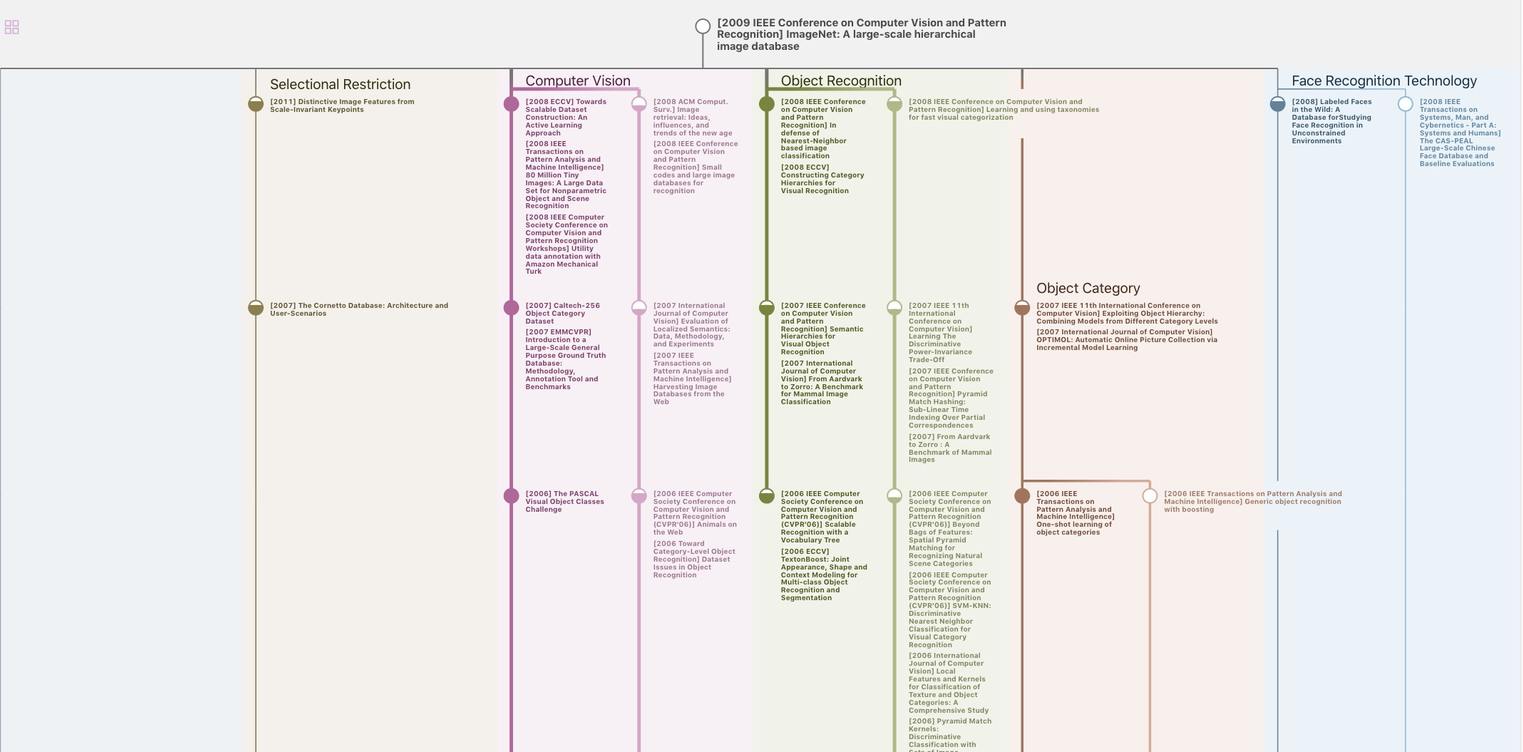
生成溯源树,研究论文发展脉络
Chat Paper
正在生成论文摘要