Learning Continuous Temporal Emb E Dding Of Videos Using Pattern Theory
PATTERN RECOGNITION LETTERS(2021)
摘要
Visual Question Answering (VQA) is a challenging task in artificial intelligence and has received increasing attention from both the computer vision and the natural language processing communities. Joint embedding learning for it suffers from the background noise of images and text. Learning continuous temporal embedding is a potential solution to extract both visual elements and textual elements with a proper temporal structure. In this paper, we address a continuous temporal embedding model based on pattern theory (CTE-PT) and fully express the atomism and combinatory based on Grenander's pattern theory. First, we generate atomic actions from videos and note them as generators, which reflects the atomism in pattern theory. Second, we design a CTE-PT model to discover the discriminative configuration of videos, which reflects the combinatory of atomic actions in pattern theory. In the CTE-PT model, we design the configuration proposal module to remove some background information initially, and the configuration interpretation module to minimize the interpretive energy of continuous temporal embedding. We estimate the energy by each pair in this embedding sequence and optimize it based on the Viterbi algorithm. The experimental results show that our CTE-PT model outperforms the baseline C3D + LSTM model on Olympic Sports, UCF 101, and HMDB51 datasets, which proves the effectiveness of mining the common continuous temporal embedding as the class-specific configuration for activity discriminator.(c) 2021 Published by Elsevier B.V.
更多查看译文
关键词
Action Recognition, Continuous Temporal Embedding, Pattern Theory, CNN, LSTM
AI 理解论文
溯源树
样例
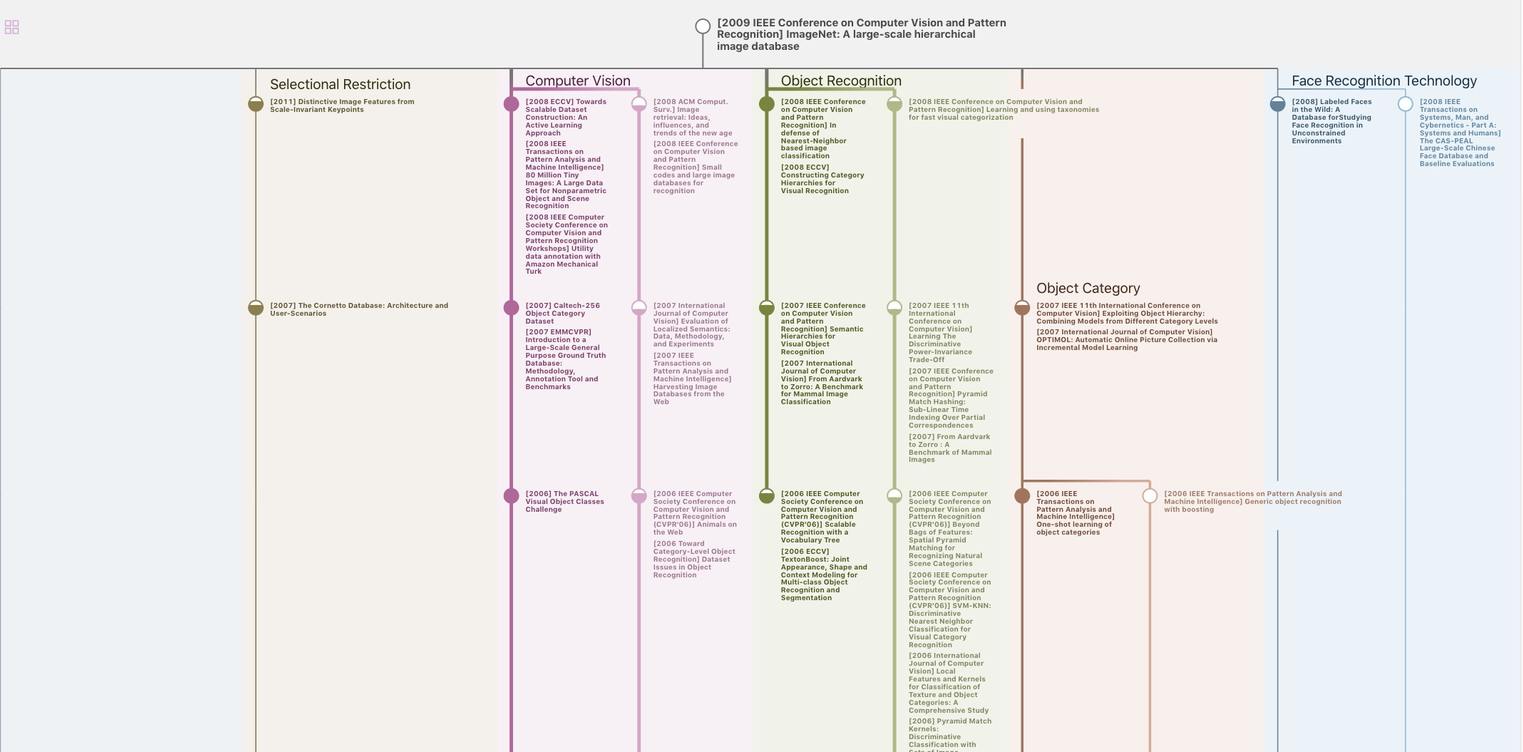
生成溯源树,研究论文发展脉络
Chat Paper
正在生成论文摘要