Perspective—Combining Physics and Machine Learning to Predict Battery Lifetime
Journal of The Electrochemical Society(2021)
摘要
Forecasting the health of a battery is a modeling effort that is critical to driving improvements in and adoption of electric vehicles. Purely physics-based models and purely data-driven models have advantages and limitations of their own. Considering the nature of battery data and end-user applications, we outline several architectures for integrating physics-based and machine learning models that can improve our ability to forecast battery lifetime. We discuss the ease of implementation, advantages, limitations, and viability of each architecture, given the state of the art in the battery and machine learning fields.
更多查看译文
关键词
Batteries, Lithium, Energy Storage, Electrochemical Engineering
AI 理解论文
溯源树
样例
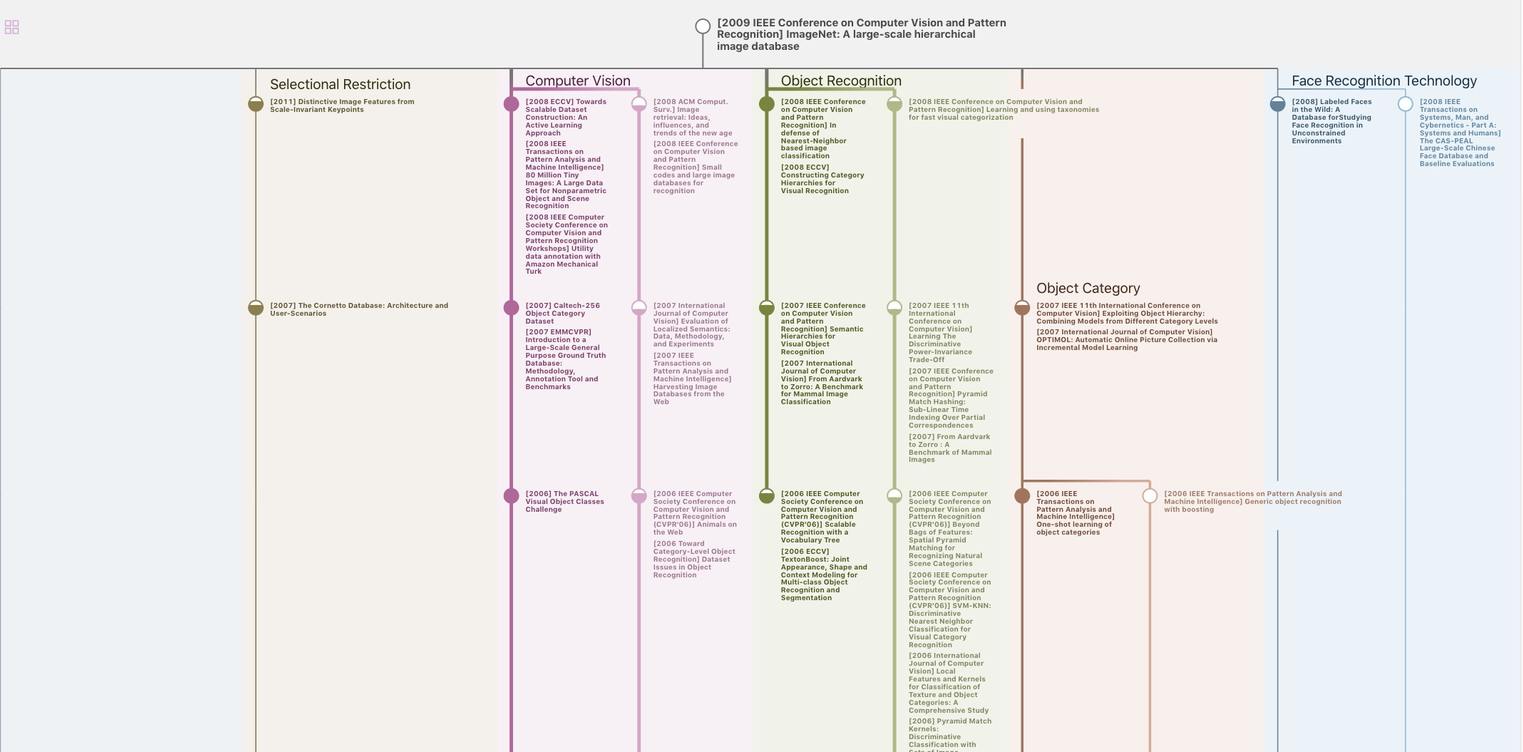
生成溯源树,研究论文发展脉络
Chat Paper
正在生成论文摘要