Micro-Metastream: Algorithm Selection For Time-Changing Data
INFORMATION SCIENCES(2021)
摘要
Data stream mining needs to deal with scenarios where data distribution can change over time. As a result, different learning algorithms can be more suitable in different time periods. This paper proposes micro-MetaStream, a meta-learning based method to recommend the most suitable learning algorithm for each new example arriving in a data stream. It is an evolution of MetaStream, which recommends learning algorithms for batches of examples. By using a unitary granularity, micro-MetaStream is able to respond more efficiently to changes in data distribution than its predecessor. The meta-data combines meta-features, characteristics describing recent data, with base-level features, the original variables of the new example. In experiments on real-world regression data streams, micro-metaStream outperformed MetaStream and a baseline method at the meta-level and frequently improved the predictive performance at the base-level.(c) 2021 Elsevier Inc. All rights reserved.
更多查看译文
关键词
Algorithm selection, Meta-learning, Time-changing data
AI 理解论文
溯源树
样例
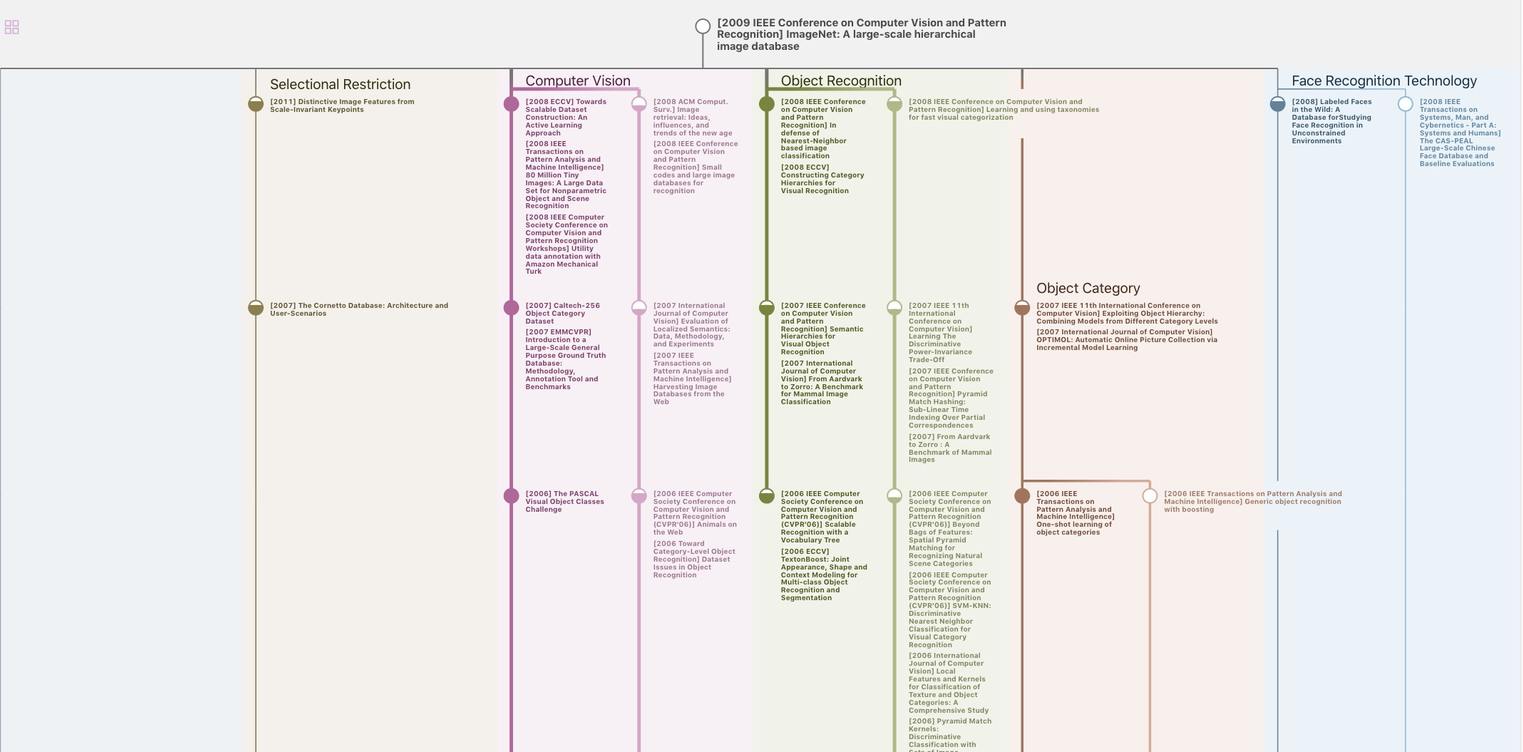
生成溯源树,研究论文发展脉络
Chat Paper
正在生成论文摘要