A Review Of Automated Techniques For Assisting The Early Detection Of Alzheimer'S Disease With A Focus On Eeg
JOURNAL OF ALZHEIMERS DISEASE(2021)
摘要
In this paper, we review state-of-the-art approaches that apply signal processing (SP) and machine learning (ML) to automate the detection of Alzheimer's disease (AD) and its prodromal stages. In the first part of the document, we describe the economic and social implications of the disease, traditional diagnosis techniques, and the fundaments of automated AD detection. Then, we present electroencephalography (EEG) as an appropriate alternative for the early detection of AD, owing to its reduced cost, portability, and non-invasiveness. We also describe the main time and frequency domain EEG features that are employed in AD detection. Subsequently, we examine some of the main studies of the last decade that aim to provide an automatic detection of AD and its previous stages by means of SP and ML. In these studies, brain data was acquired using multiple medical techniques such as magnetic resonance imaging, positron emission tomography, and EEG. The main aspects of each approach, namely feature extraction, classification model, validation approach, and performance metrics, are compiled and discussed. Lastly, a set of conclusions and recommendations for future research on AD automatic detection are drawn in the final section of the paper.
更多查看译文
关键词
Alzheimer's disease, early diagnosis, electroencephalography, machine learning
AI 理解论文
溯源树
样例
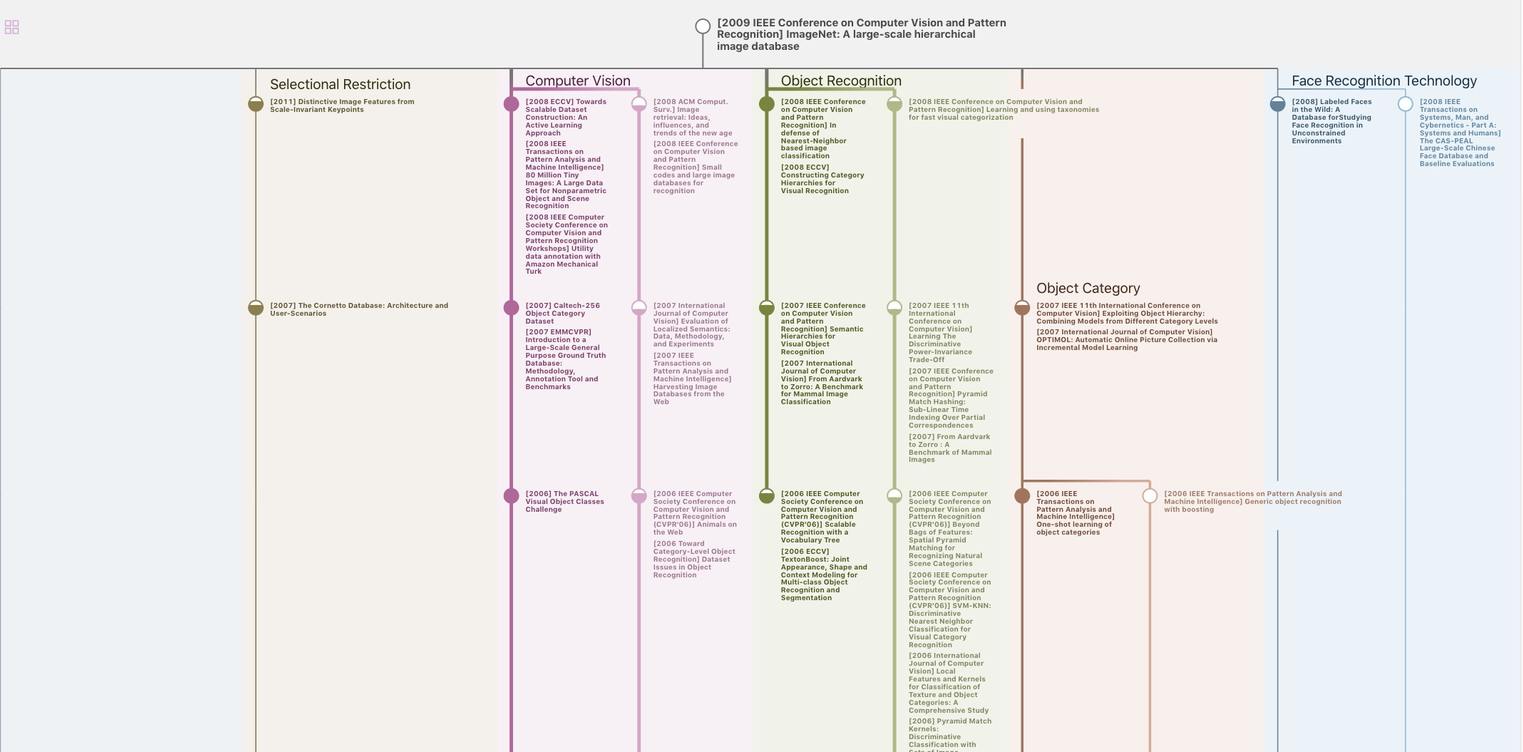
生成溯源树,研究论文发展脉络
Chat Paper
正在生成论文摘要