Taming The Challenges Of Activity And Selectivity In The Electrochemical Nitrogen Reduction Reaction Using Graphdiyne-Supported Double-Atom Catalysts
JOURNAL OF MATERIALS CHEMISTRY A(2021)
摘要
Developing highly efficient electrocatalysts for the electrochemical nitrogen reduction reaction (eNRR) is becoming increasingly important but remains a great challenge due to the lack of strategies to solve the problems of activity and selectivity. By means of systematic density functional theory (DFT) calculations, this work focuses on understanding and addressing this challenge by building a full profile of the stability, activity, and selectivity of the eNRR on a model electrocatalyst system, i.e., graphdiyne (GDY)-supported Fe-based transition metal (M) double-atom catalysts (FeM-GDYs). Based on the studies on 26 FeM-GDYs, the activity trends of the catalysts are constructed using the adsorption energy of the *NH2 intermediate (E-ads(*NH2)) as a descriptor; this displays a well-defined volcano-shaped relationship, which enables us to identify 11 FeM-GDYs that show improved activities in comparison with that of the Ru(0001) stepped surface, which is used as a benchmark. Employing the difference in the free energies of the H and N-2 adsorption processes, i.e., Delta G(*N-2) - Delta G(*H), as a selectivity descriptor, 3 FeM-GDYs (M = Ni, Mo, and Cr) are finally demonstrated to exhibit a strong ability to suppress the competitive hydrogen evolution reaction (HER). Their improved activity and selectivity have been discussed and explained based on the alteration of the electronic structures and the coordination environments of the metal atoms upon FeM-GDY formation. More importantly, these FeM-GDY catalysts have a high potential for experimental synthesis. These results not only contribute to more efficient electrocatalysts toward the eNRR but also provide an effective way to find new substrates for catalyst synthesis and a guideline to improve the activity and selectivity of the resulting catalysts.
更多查看译文
AI 理解论文
溯源树
样例
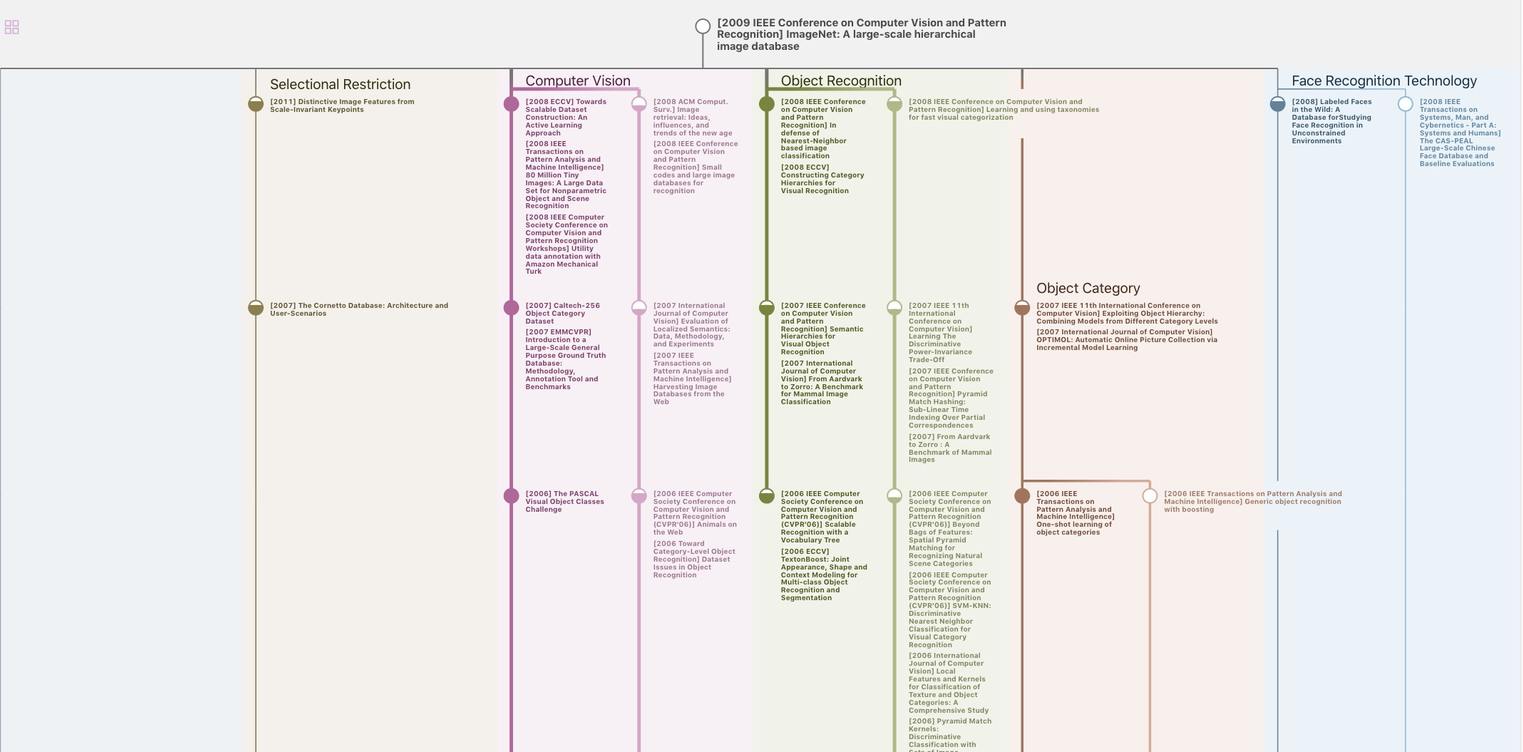
生成溯源树,研究论文发展脉络
Chat Paper
正在生成论文摘要