Representation Learning Based On Autoencoder And Deep Adaptive Clustering For Image Clustering
MATHEMATICAL PROBLEMS IN ENGINEERING(2021)
摘要
Image clustering is a complex procedure, which is significantly affected by the choice of image representation. Most of the existing image clustering methods treat representation learning and clustering separately, which usually bring two problems. On the one hand, image representations are difficult to select and the learned representations are not suitable for clustering. On the other hand, they inevitably involve some clustering step, which may bring some error and hurt the clustering results. To tackle these problems, we present a new clustering method that efficiently builds an image representation and precisely discovers cluster assignments. For this purpose, the image clustering task is regarded as a binary pairwise classification problem with local structure preservation. Specifically, we propose here such an approach for image clustering based on a fully convolutional autoencoder and deep adaptive clustering (DAC). To extract the essential representation and maintain the local structure, a fully convolutional autoencoder is applied. To manipulate feature to clustering space and obtain a suitable image representation, the DAC algorithm participates in the training of autoencoder. Our method can learn an image representation that is suitable for clustering and discover the precise clustering label for each image. A series of real-world image clustering experiments verify the effectiveness of the proposed algorithm.
更多查看译文
AI 理解论文
溯源树
样例
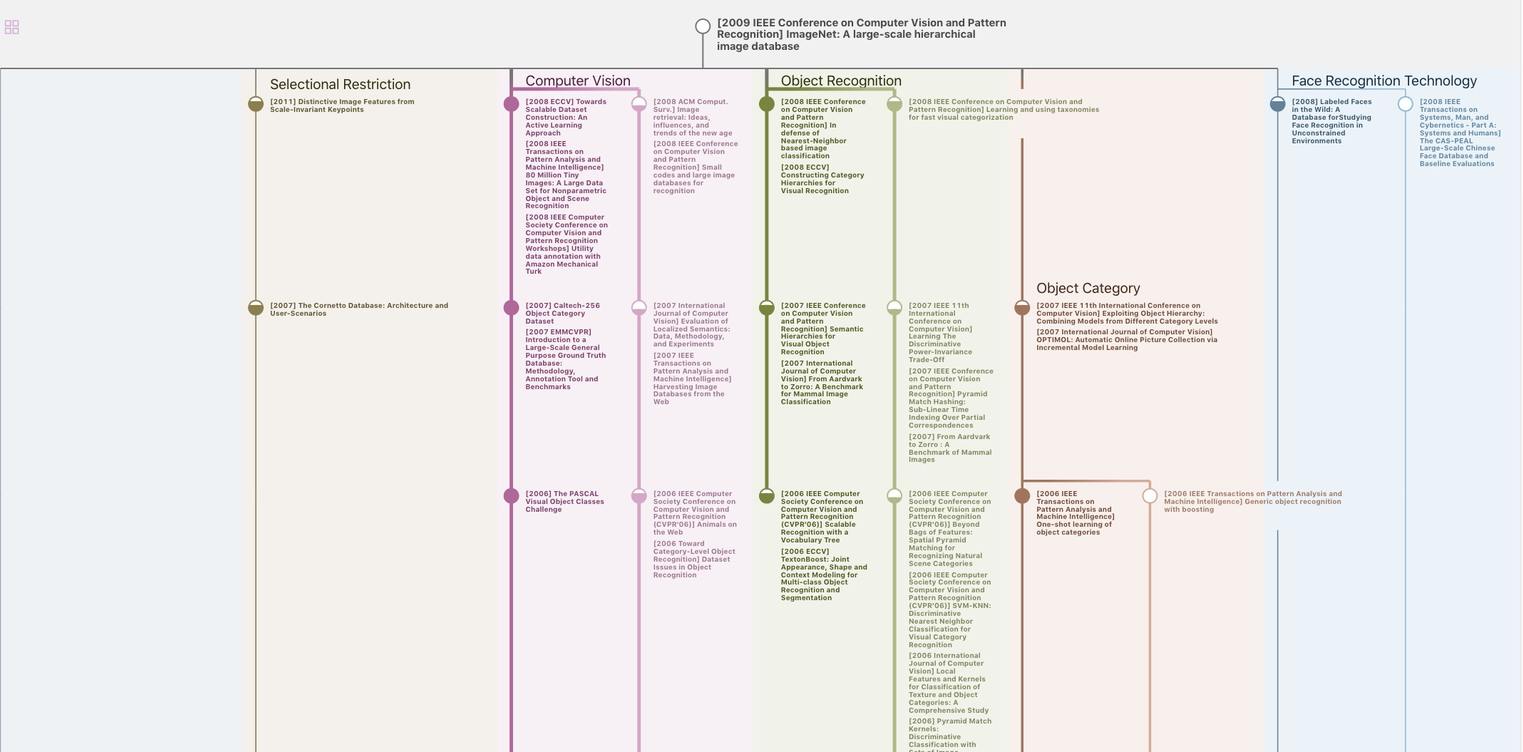
生成溯源树,研究论文发展脉络
Chat Paper
正在生成论文摘要