Advance Machine Learning Methods For Dyslexia Biomarker Detection: A Review Of Implementation Details And Challenges
IEEE ACCESS(2021)
摘要
Dyslexia is a neurological disorder that is characterized by imprecise comprehension of words and generally poor reading performance. It affects a significant population of school-age children, with more occurrences in males, thus, putting them at risk of poor academic performance and low self-esteem for a lifetime. The long-term hope is to have a dyslexia diagnostic method that is informed by neural-biomarkers. In this regard, large numbers of machine learning methods and, more recently, deep learning methods have been implemented across various types of dataset with the above-chance classification accuracy. However, attainment of clinical acceptability of these state-of-the-art methods is bedeviled by certain challenges including lack of biologically-interpretable biomarkers, privacy of dataset and classifiers, hyper-parameter selection/optimization, and overfitting problem among others. This review paper critically analyzes recent machine learning methods for detecting dyslexia and its biomarkers and discusses challenges that require proper attentions from the users of deep learning methods in order to enable them to attain clinically relevance and acceptable level. The review is conducted within the premise of implementation and experimental outcomes for each of the 22 selected articles using the Preferred Reporting Items for Systematic review and Meta-Analyses (PRISMA) protocol, with a view to outlining some critical challenges for achieving high accuracy and reliability of the state-of-the-art machine learning methods. As an evidence-based protocol for reporting in systematic reviews and meta-analyses, PRISMA helps to ensure clarity and transparency of this paper by showing a four-phase flow diagram of the selection process for articles used in this review. It is therefore, envisaged that higher classification performance of clinical relevance can be achieved using deep learning models for dyslexia and its biomarkers by addressing identified potential challenges.
更多查看译文
关键词
Support vector machines, Machine learning, Feature extraction, Deep learning, Visualization, Machine learning algorithms, Radio frequency, Dyslexia detection, biomarkers, feature extraction, classification algorithms, machine learning, deep learning
AI 理解论文
溯源树
样例
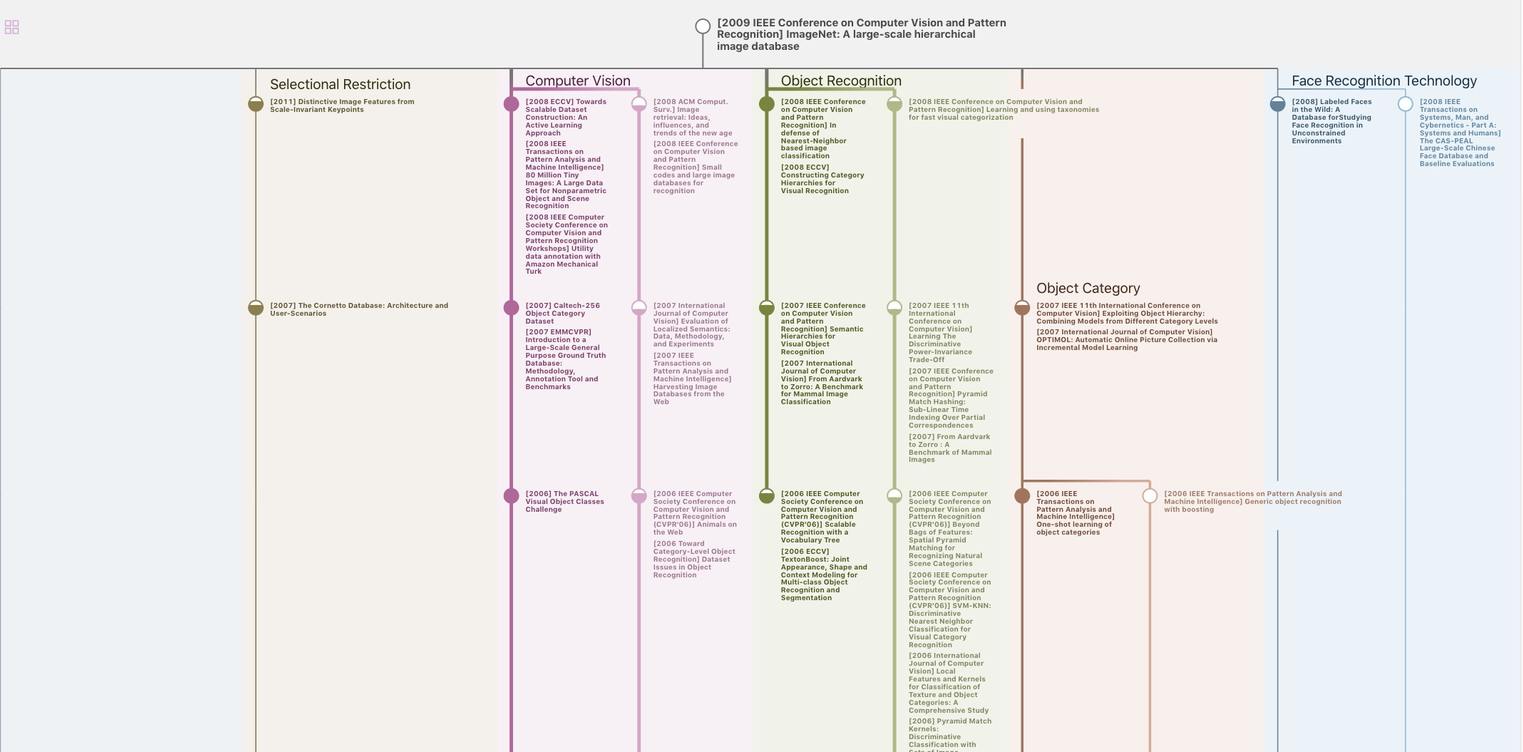
生成溯源树,研究论文发展脉络
Chat Paper
正在生成论文摘要