Learning To Detect Incongruence In News Headline And Body Text Via A Graph Neural Network
IEEE ACCESS(2021)
摘要
This paper tackles the problem of detecting incongruities between headlines and body text, where a news headline is irrelevant or even in opposition to the information in its body. Our model, called the graph-based hierarchical dual encoder (GHDE), utilizes a graph neural network to efficiently learn the content similarity between news headlines and long body paragraphs. This paper also releases a million-item-scale dataset of incongruity labels that can be used for training. The experimental results show that the proposed graph-based neural network model outperforms previous state-of-the-art models by a substantial margin (5.3%) on the area under the receiver operating characteristic (AUROC) curve. Real-world experiments on recent news articles confirm that the trained model successfully detects headline incongruities. We discuss the implications of these findings for combating infodemics and news fatigue.
更多查看译文
关键词
Graph neural networks, Media, Training, Task analysis, Licenses, Deep learning, Recurrent neural networks, Graph neural network, headline incongruity, online misinformation
AI 理解论文
溯源树
样例
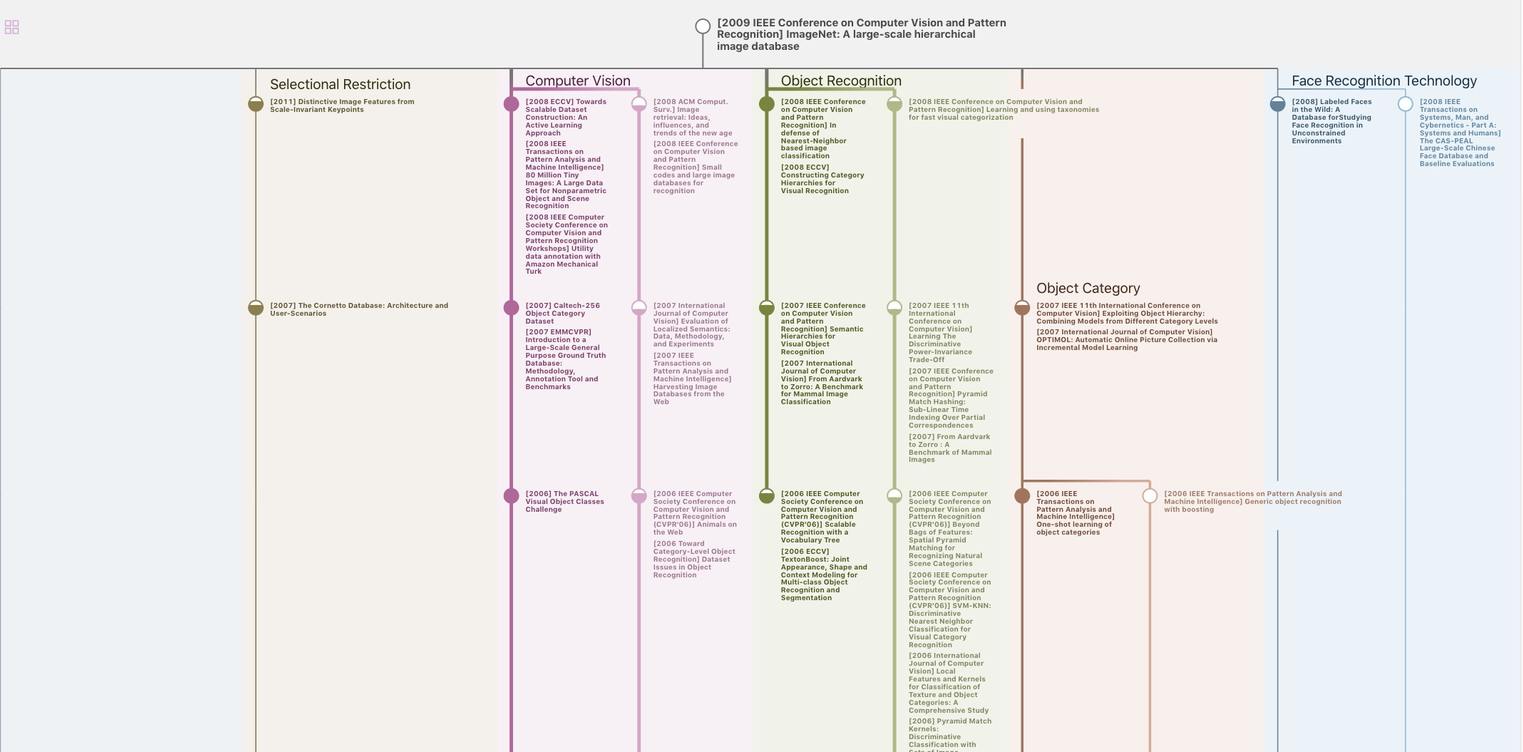
生成溯源树,研究论文发展脉络
Chat Paper
正在生成论文摘要