Spatial-temporal prediction of ambient nitrogen dioxide and ozone levels over Italy using a Random Forest model for population exposure assessment
AIR QUALITY ATMOSPHERE AND HEALTH(2021)
摘要
We developed an integrated approach coupling a chemical transport model (CTM) with machine learning (ML) techniques to produce high spatial resolution NO 2 and O 3 daily concentration fields over Italy. Three years (2013–2015) simulations, at a spatial resolution of 5 km, performed by the Flexible Air quality Regional Model (FARM) were used as predictors, together with other spatial-temporal data, such as population, land-use, surface greenness and road networks, by a ML Random Forest (ML-RF) algorithm to produce daily concentrations at higher resolution (1 km) over the national territory. The evaluation of the adopted integrated approach was based on NO 2 and O 3 observations available from 530 and 293 monitoring stations across Italy, respectively. A good performance for NO 2 and excellent results for O 3 were obtained from the application of the CTM; as for NO 2 , the levels at urban traffic stations were not captured by the simulations due to the adopted horizontal resolution and related emissions uncertainties. Performance improvements were achieved with ML-RF predictions, reducing NO 2 underestimation (near zero fractional bias results) and better capturing spatial contrasts. The results obtained in this work were used to support the national exposure assessment and environmental epidemiology studies planned in the BEEP (Big data in Environmental and occupational Epidemiology) project and confirm the potential of machine learning methods to adequately predict air pollutant levels at high spatial and temporal resolutions. Graphical abstract
更多查看译文
关键词
Chemical transport model, FARM, WRF, Machine learning, Random Forest, Nitrogen dioxide, Ozone
AI 理解论文
溯源树
样例
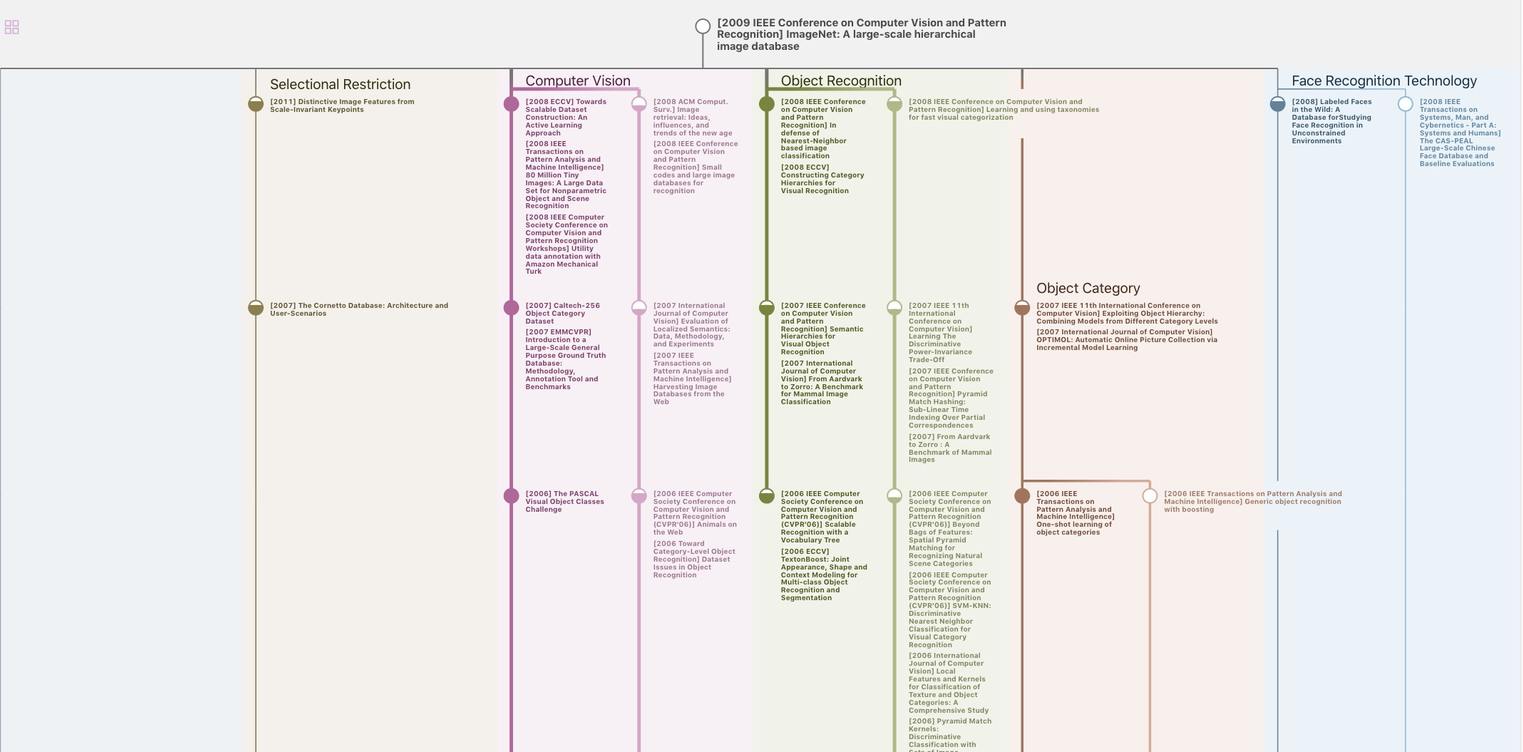
生成溯源树,研究论文发展脉络
Chat Paper
正在生成论文摘要