A Detailed Catalogue Of Multi-Omics Methodologies For Identification Of Putative Biomarkers And Causal Molecular Networks In Translational Cancer Research
INTERNATIONAL JOURNAL OF MOLECULAR SCIENCES(2021)
摘要
Recent advances in sequencing and biotechnological methodologies have led to the generation of large volumes of molecular data of different omics layers, such as genomics, transcriptomics, proteomics and metabolomics. Integration of these data with clinical information provides new opportunities to discover how perturbations in biological processes lead to disease. Using data-driven approaches for the integration and interpretation of multi-omics data could stably identify links between structural and functional information and propose causal molecular networks with potential impact on cancer pathophysiology. This knowledge can then be used to improve disease diagnosis, prognosis, prevention, and therapy. This review will summarize and categorize the most current computational methodologies and tools for integration of distinct molecular layers in the context of translational cancer research and personalized therapy. Additionally, the bioinformatics tools Multi-Omics Factor Analysis (MOFA) and netDX will be tested using omics data from public cancer resources, to assess their overall robustness, provide reproducible workflows for gaining biological knowledge from multi-omics data, and to comprehensively understand the significantly perturbed biological entities in distinct cancer types. We show that the performed supervised and unsupervised analyses result in meaningful and novel findings.
更多查看译文
关键词
translational cancer research, oncology, multi-omics data integration, supervised data integration, unsupervised data integration, integrative methods, analysis tools, literature review, personalized medicine
AI 理解论文
溯源树
样例
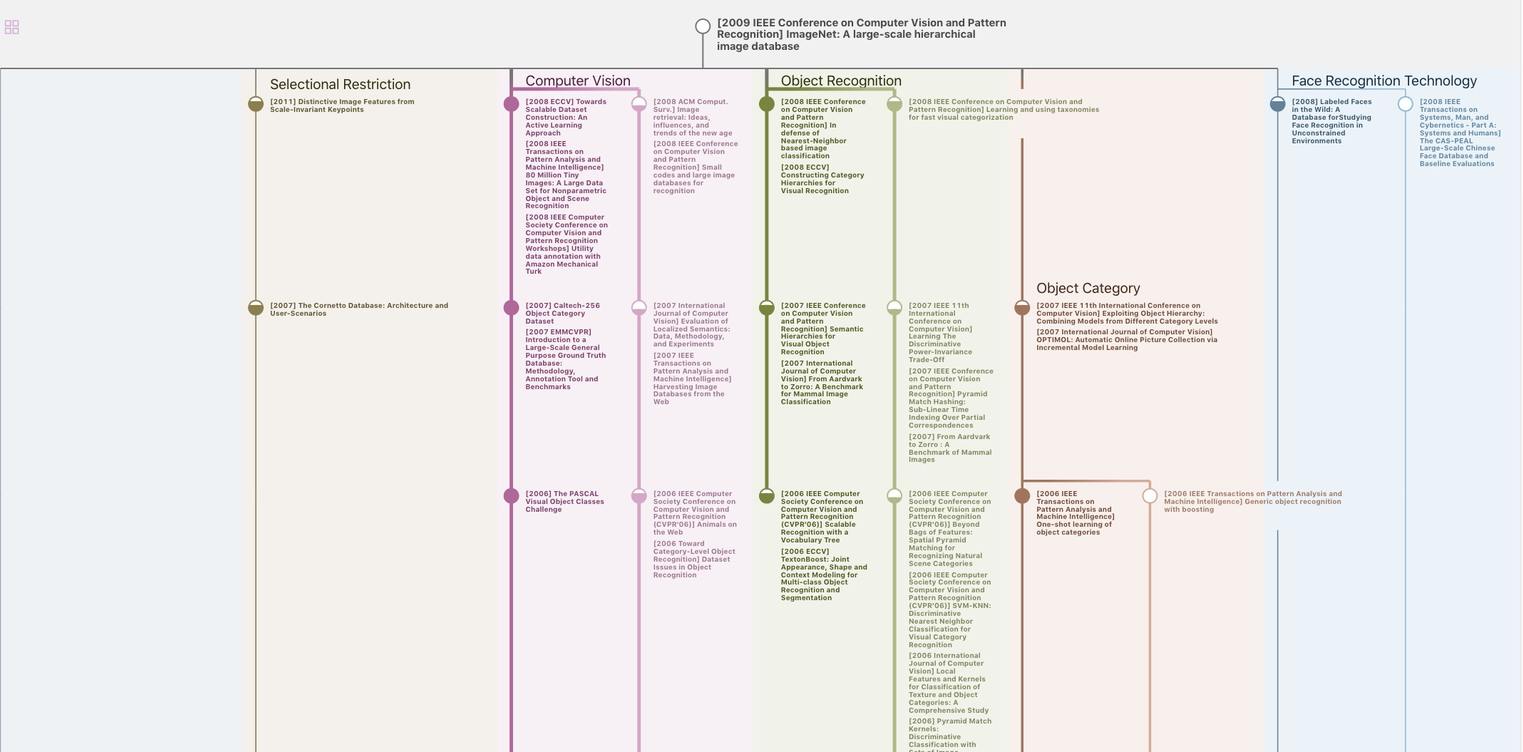
生成溯源树,研究论文发展脉络
Chat Paper
正在生成论文摘要