Fast Optimal Subsampling Probability Approximation for Generalized Linear Models
ECONOMETRICS AND STATISTICS(2024)
摘要
For massive data, subsampling techniques are popular to mitigate computational burden by reducing the data size. In a subsampling approach, subsampling probabilities for each data point are specified to obtain an informative sub-data, and then estimates based on the sub-data are obtained to approximate estimates from the full data. Assigning subsampling probabilities based on minimization of the asymptotic mean squared error of the estimator from a general subsample (A-optimality criterion) is a popular approach, however, it is still computationally demanding to calculate the probabilities under this setting. To efficiently approximate the A-optimal subsampling probabilities for generalized linear models, randomized algorithms are proposed. To develop the algorithms, the Johnson-Lindenstrauss Transform and Subsampled Randomized Hadamard Transform are used. Additionally, optimal subsampling probabilities are derived for the Gaussian linear model in the case where both the regression coefficients and dispersion parameter are of interest, and algorithms are developed to approximate the optimal subsampling probabilities. Simulation studies indicate that the estimators based on the developed algorithms have excellent performance for statistical inference and have substantial savings in computing time compared to the direct calculation of the A-optimal subsampling probabilities.(c) 2021 EcoSta Econometrics and Statistics. Published by Elsevier B.V. All rights reserved.
更多查看译文
关键词
Generalized linear models,Massive data,Optimal subsampling,Randomized algorithm
AI 理解论文
溯源树
样例
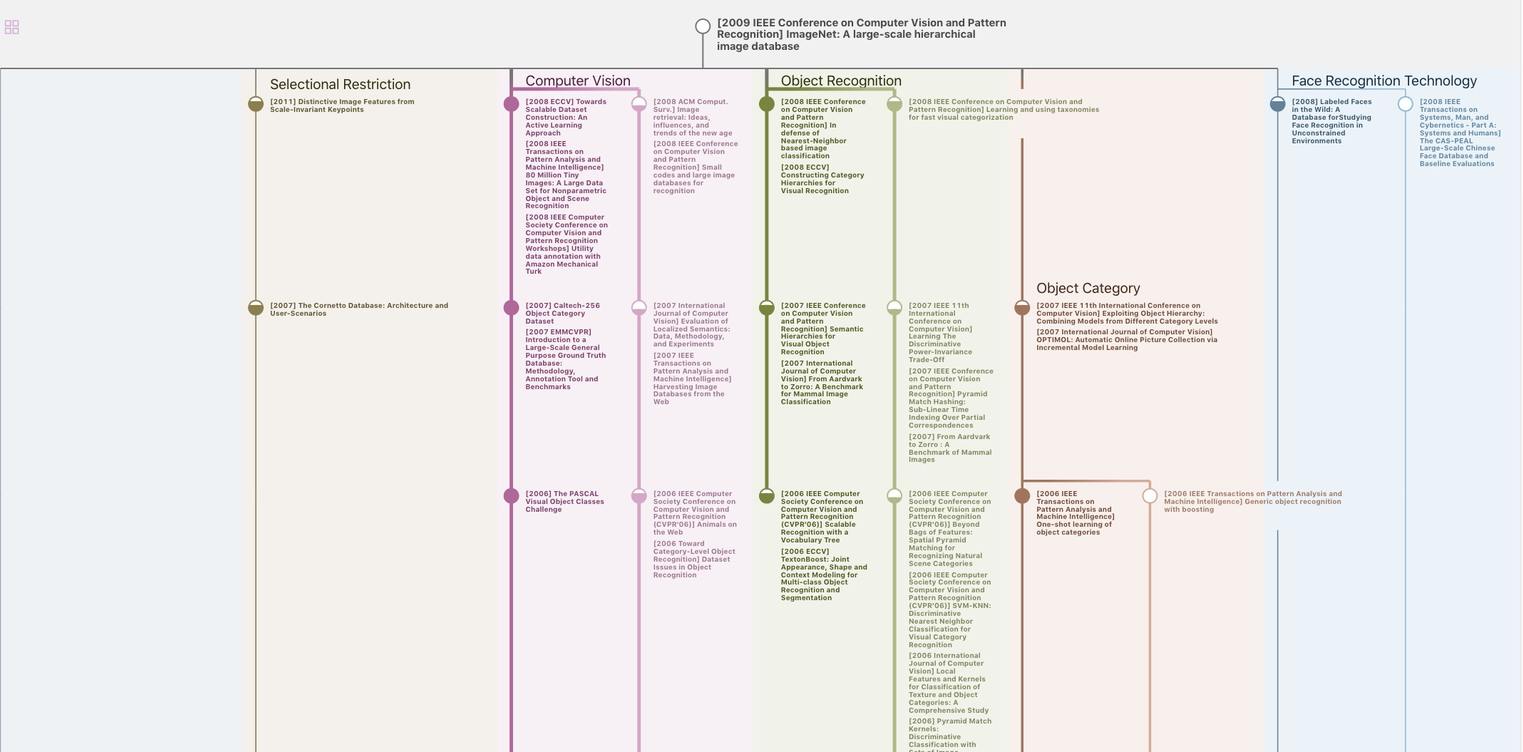
生成溯源树,研究论文发展脉络
Chat Paper
正在生成论文摘要