Optimality Assessments Of Classifiers On Single And Multi-Labelled Obstetrics Outcome Classification Problems
INTERNATIONAL JOURNAL OF ADVANCED COMPUTER SCIENCE AND APPLICATIONS(2021)
摘要
It is indisputable that clinicians cannot exactly state the outcome of pregnancies through conventional knowledge and methods even as the surge in human knowledge continues. Hence, several computational techniques have been adapted for precise pregnancy outcome (PO) prediction. Obstetric datasets for PO determination exist as single label learning (SLL), multi-label learning (MLL) and multi-target (MTP) problems. There is however no single classifier recommended to optimally satisfy the needs of all the classification types. This work therefore identifies six widely used PO classifiers and investigates their performances in all three classification categories; to find the best performing classifier. Obstetric dataset exposed to input rank analysis via Principal component Analysis, produced thirteen (13) significant features for the experiment. Accuracy, F1-measure and build/test time were used as evaluation metrics. Decision tree (DT) had an average accuracy and F1 score of 89.23% and 88.23% respectively, with 1.0 average rank. Under MLL configuration, average accuracy (91.71%) and F1 score (94.28%) were highest in the random forest (RF) which had a 1.0 average test time rank. Using MTP, DT had an average accuracy of 88.80% and average F1 score of 71.13%, the multi-layered perceptron (MLP) had the best time cost with an average rank value of 2.0. From the results, RF is most optimal in terms of accuracy and average rank value, while DT is the most efficient in terms of time cost. The comparative analysis of global averages of the six base classifiers shows that RF is the most optimal algorithm with an average accuracy of 87.3% given all three data setups in the study. MLP on the other hand had an unexpectedly high time cost, making it unsuitable for similar data classifications if time is the main criterion. It is recommended that the choice of the classifier should either be RF or DT depending on the application domain and whether or not time cost is a major consideration.
更多查看译文
关键词
Pregnancy outcome, random forest, multi-label learning, comparative analytics, machine learning algorithms, single label learning, maternal outcome prediction, decision tree
AI 理解论文
溯源树
样例
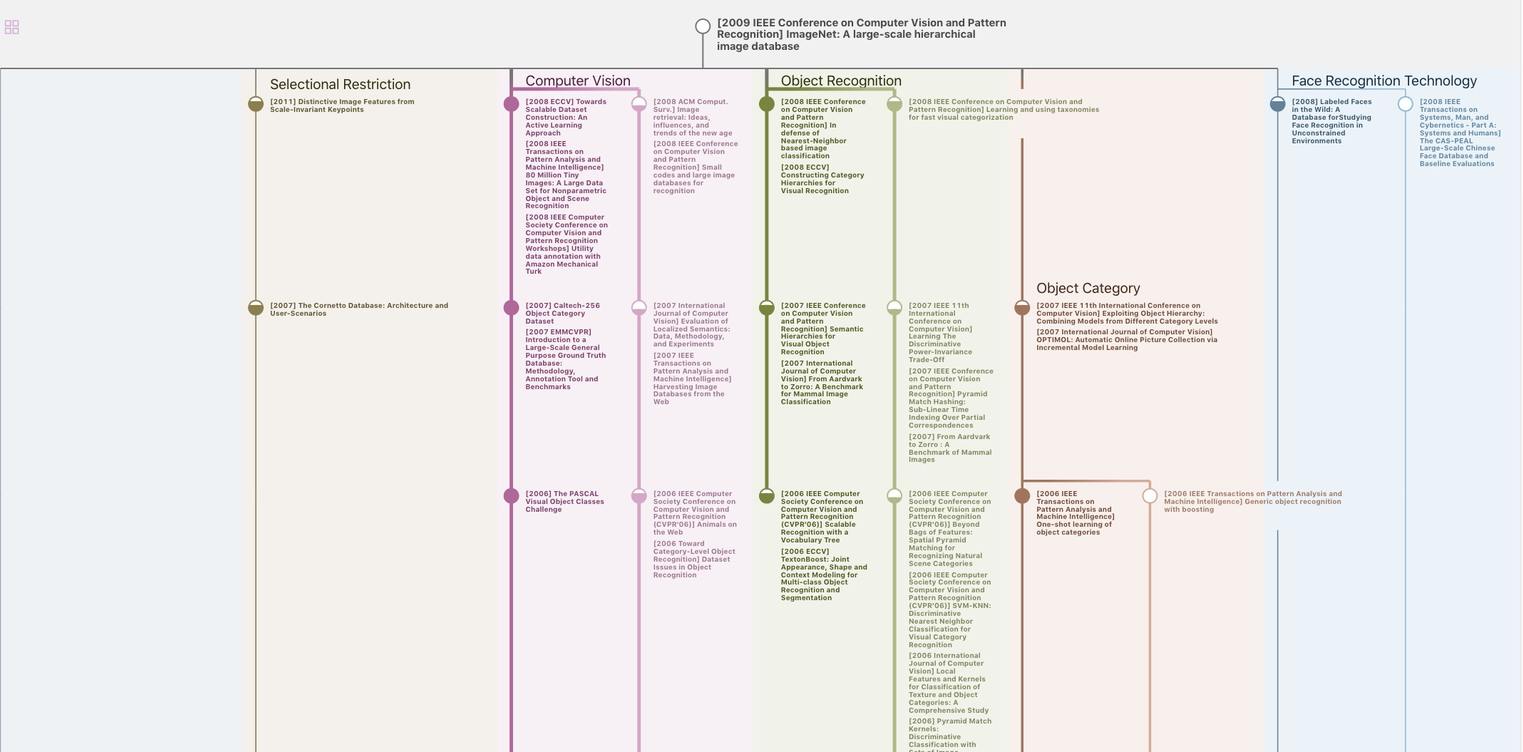
生成溯源树,研究论文发展脉络
Chat Paper
正在生成论文摘要