Versatile Auxiliary Classification And Regression With Generative Adversarial Networks
IEEE ACCESS(2021)
摘要
One of the most interesting challenges in Artificial Intelligence is to train conditional generators which are able to provide labeled adversarial samples drawn from a specific distribution. For a successful implementation of conditional generators, the created samples are constrained to a specific class. In this work, a new framework is presented to train a deep conditional generator by placing a classifier or regression model in parallel with the discriminator and back propagate the classification or regression error through the generator network. Special cases for binary classification, multi-class classification, and regression are studied. Experimental results on several data-sets are provided and the results are compared with similar state-of-the-art techniques. The main advantage of the method is that it is versatile and applicable to any variation of Generative Adversarial Network (GAN) implementation but also it is shown to obtain superior results compared to other methods. The mathematical proofs for the proposed scheme for both classification and regression are presented.
更多查看译文
关键词
Generators, Gallium nitride, Generative adversarial networks, Task analysis, Databases, Training, Data models, Conditional generators, deep neural networks, generative adversarial networks
AI 理解论文
溯源树
样例
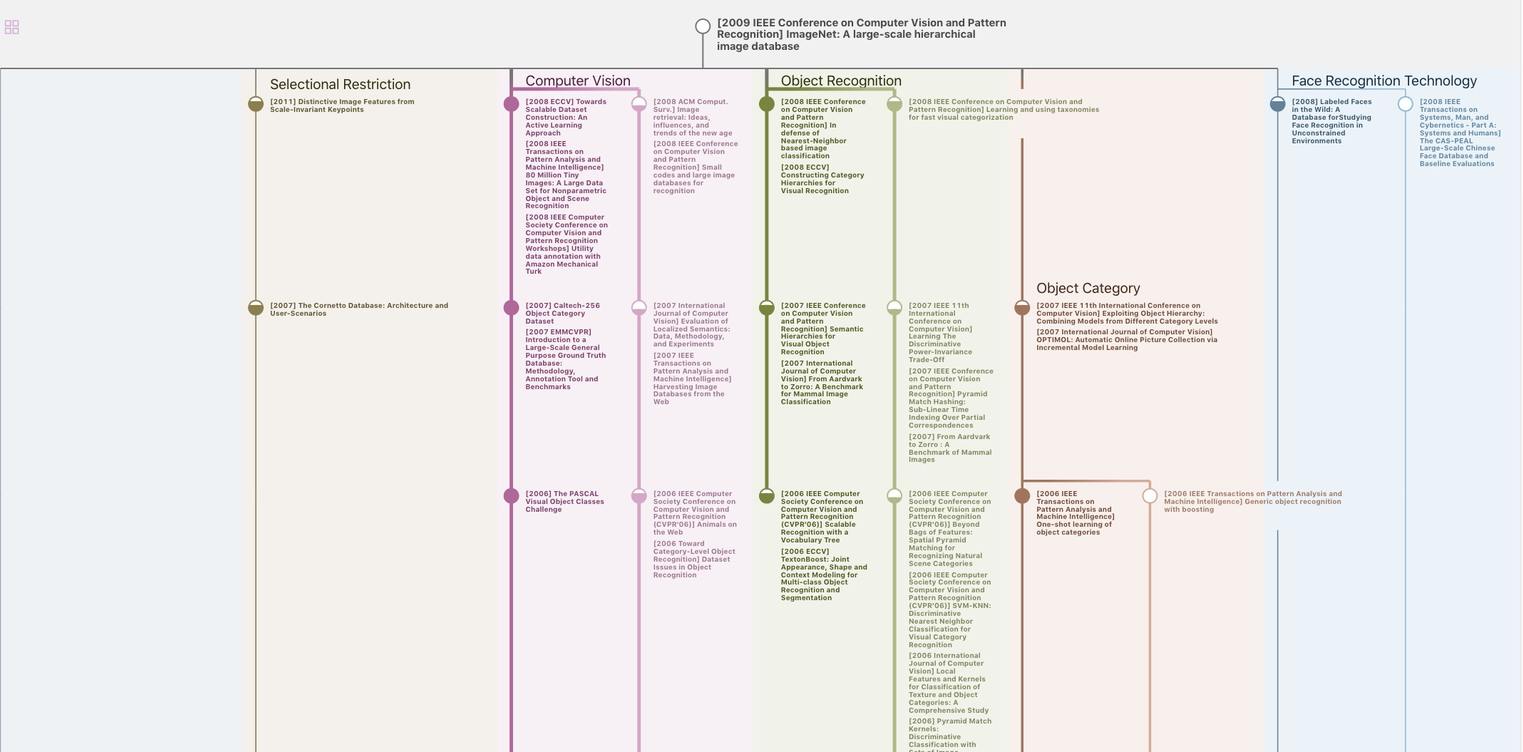
生成溯源树,研究论文发展脉络
Chat Paper
正在生成论文摘要