UGRec: Modeling Directed and Undirected Relations for Recommendation
Research and Development in Information Retrieval(2021)
摘要
ABSTRACTThe recommender systems, which merely leverage user-item interactions for user preference prediction (such as the collaborative filtering-based ones), often face dramatic performance degradation when the interactions of users or items are insufficient. In recent years, various types of side information have been explored to alleviate this problem. Among them, knowledge graph (KG) has attracted extensive research interests as it can encode users/items and their associated attributes in the graph structure to preserve the relation information. In contrast, less attention has been paid to the item-item co-occurrence information (i.e., co-view), which contains rich item-item similarity information. It provides information from a perspective different from the user/item-attribute graph and is also valuable for the CF recommendation models. In this work, we make an effort to study the potential of integrating both types of side information (i.e., KG and item-item co-occurrence data) for recommendation. To achieve the goal, we propose a unified graph-based recommendation model (UGRec), which integrates the traditional directed relations in KG and the undirected item-item co-occurrence relations simultaneously. In particular, for a directed relation, we transform the head and tail entities into the corresponding relation space to model their relation; and for an undirected co-occurrence relation, we project head and tail entities into a unique hyperplane in the entity space to minimize their distance. In addition, a head-tail relation-aware attentive mechanism is designed for fine-grained relation modeling.
更多查看译文
关键词
Graph-based, Metric Learning, Recommendation, Attention Mechanism
AI 理解论文
溯源树
样例
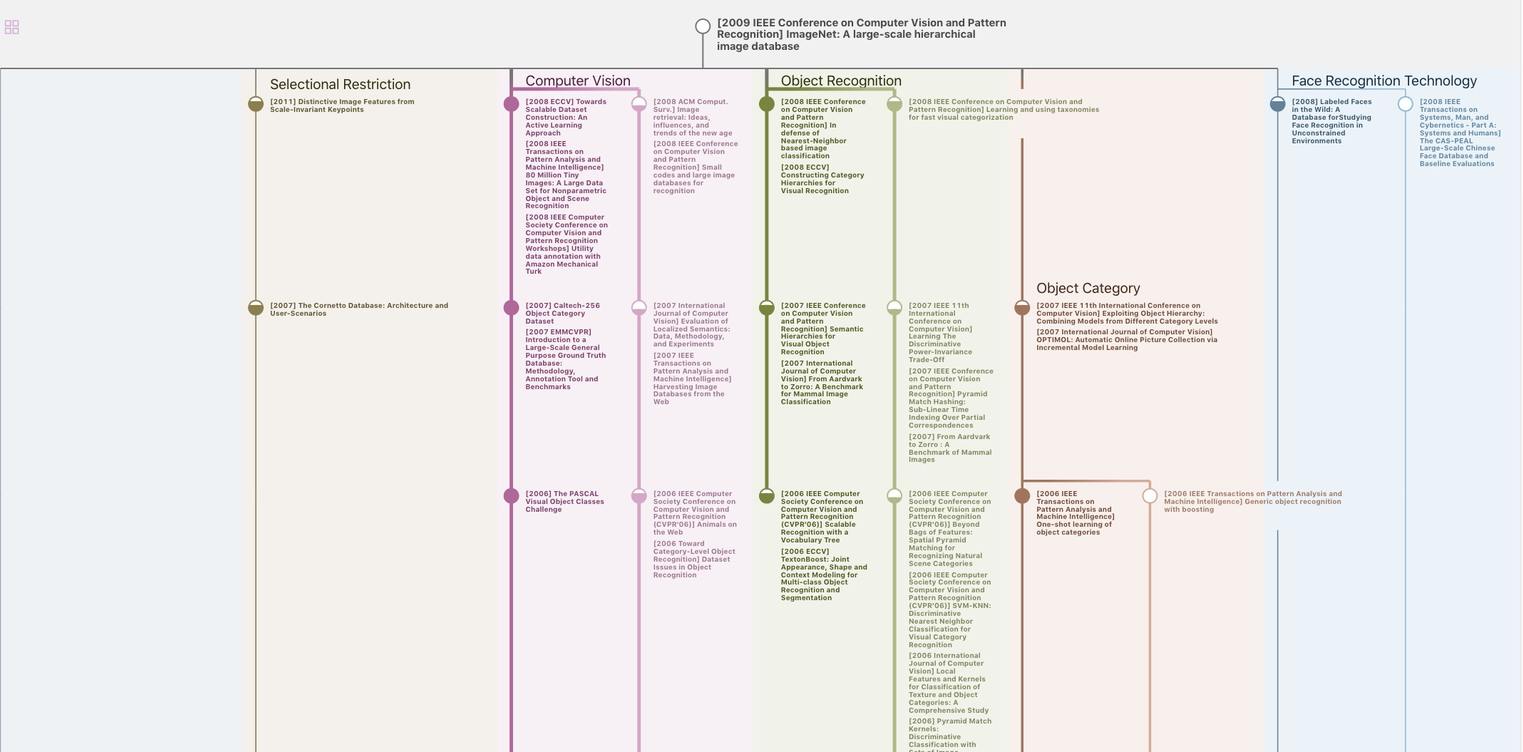
生成溯源树,研究论文发展脉络
Chat Paper
正在生成论文摘要