MuseMorphose: Full-Song and Fine-Grained Piano Music Style Transfer with One Transformer VAE
arxiv(2022)
摘要
Transformers and variational autoencoders (VAE) have been extensively employed for symbolic (e.g., MIDI) domain music generation. While the former boast an impressive capability in modeling long sequences, the latter allow users to willingly exert control over different parts (e.g., bars) of the music to be generated. In this paper, we are interested in bringing the two together to construct a single model that exhibits both strengths. The task is split into two steps. First, we equip Transformer decoders with the ability to accept segment-level, time-varying conditions during sequence generation. Subsequently, we combine the developed and tested in-attention decoder with a Transformer encoder, and train the resulting MuseMorphose model with the VAE objective to achieve style transfer of long pop piano pieces, in which users can specify musical attributes including rhythmic intensity and polyphony (i.e., harmonic fullness) they desire, down to the bar level. Experiments show that MuseMorphose outperforms recurrent neural network (RNN) based baselines on numerous widely-used metrics for style transfer tasks.
更多查看译文
关键词
Measurement, Recurrent neural networks, Music, Transformers, Harmonic analysis, Decoding, Task analysis, Transformer, variational autoencoder (VAE), deep learning, controllable music generation, music style transfer
AI 理解论文
溯源树
样例
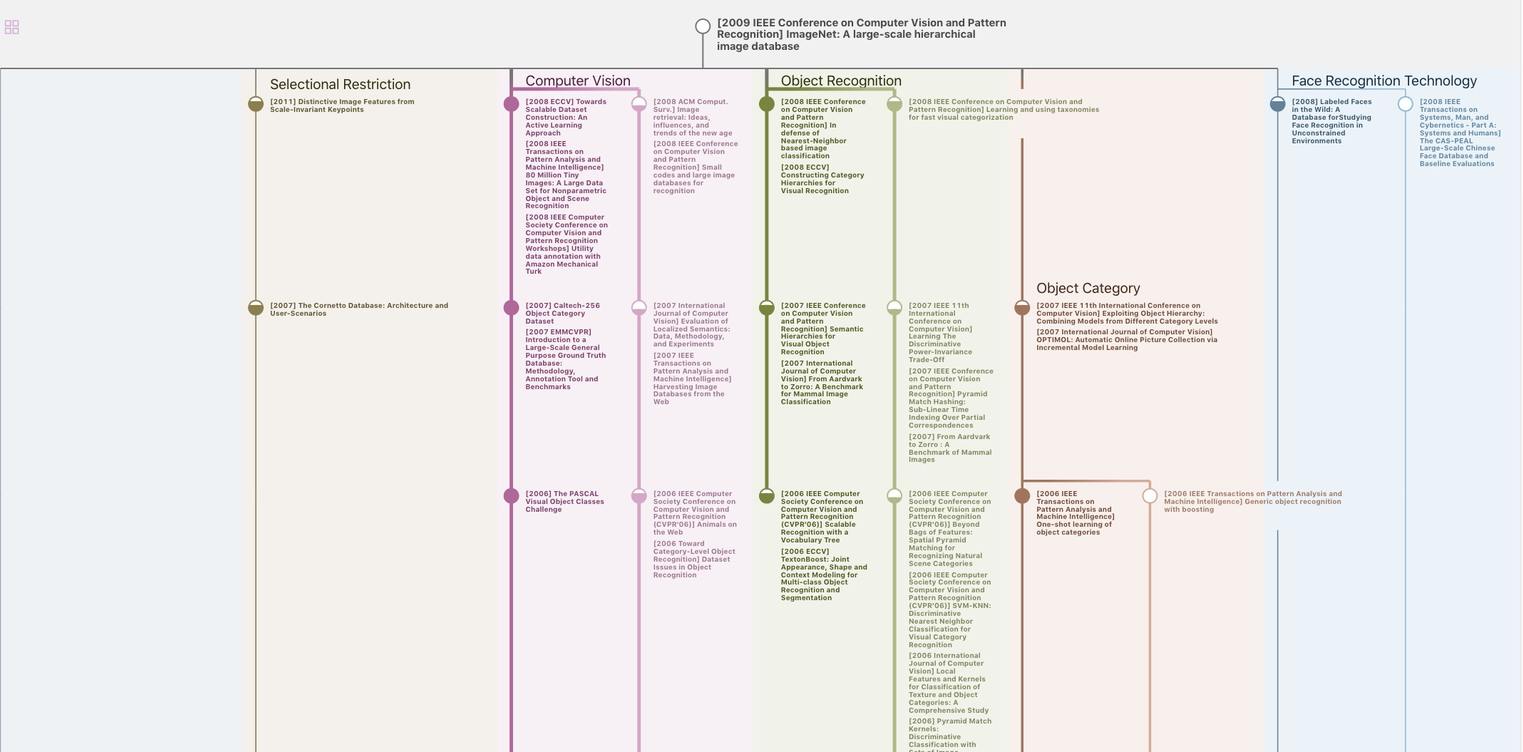
生成溯源树,研究论文发展脉络
Chat Paper
正在生成论文摘要