CASA: Bridging the Gap between Policy Improvement and Policy Evaluation with Conflict Averse Policy Iteration
arxiv(2023)
摘要
We study the problem of model-free reinforcement learning, which is often solved following the principle of Generalized Policy Iteration (GPI). While GPI is typically an interplay between policy evaluation and policy improvement, most conventional model-free methods assume the independence of the granularity and other details of the GPI steps, despite of the inherent connections between them. In this paper, we present a method that regularizes the inconsistency between policy evaluation and policy improvement, leading to a conflict averse GPI solution with reduced functional approximation error. To this end, we formulate a novel learning paradigm where taking the policy evaluation step is equivalent to some compensation of performing policy improvement, and thus effectively alleviates the gradient conflict between the two GPI steps. We also show that the form of our proposed solution is equivalent to performing entropy-regularized policy improvement and therefore prevents the policy from being trapped into suboptimal solutions. We conduct extensive experiments to evaluate our method on the Arcade Learning Environment (ALE). Empirical results show that our method outperforms several strong baselines in major evaluation domains.
更多查看译文
关键词
reinforcement learning,policy iteration
AI 理解论文
溯源树
样例
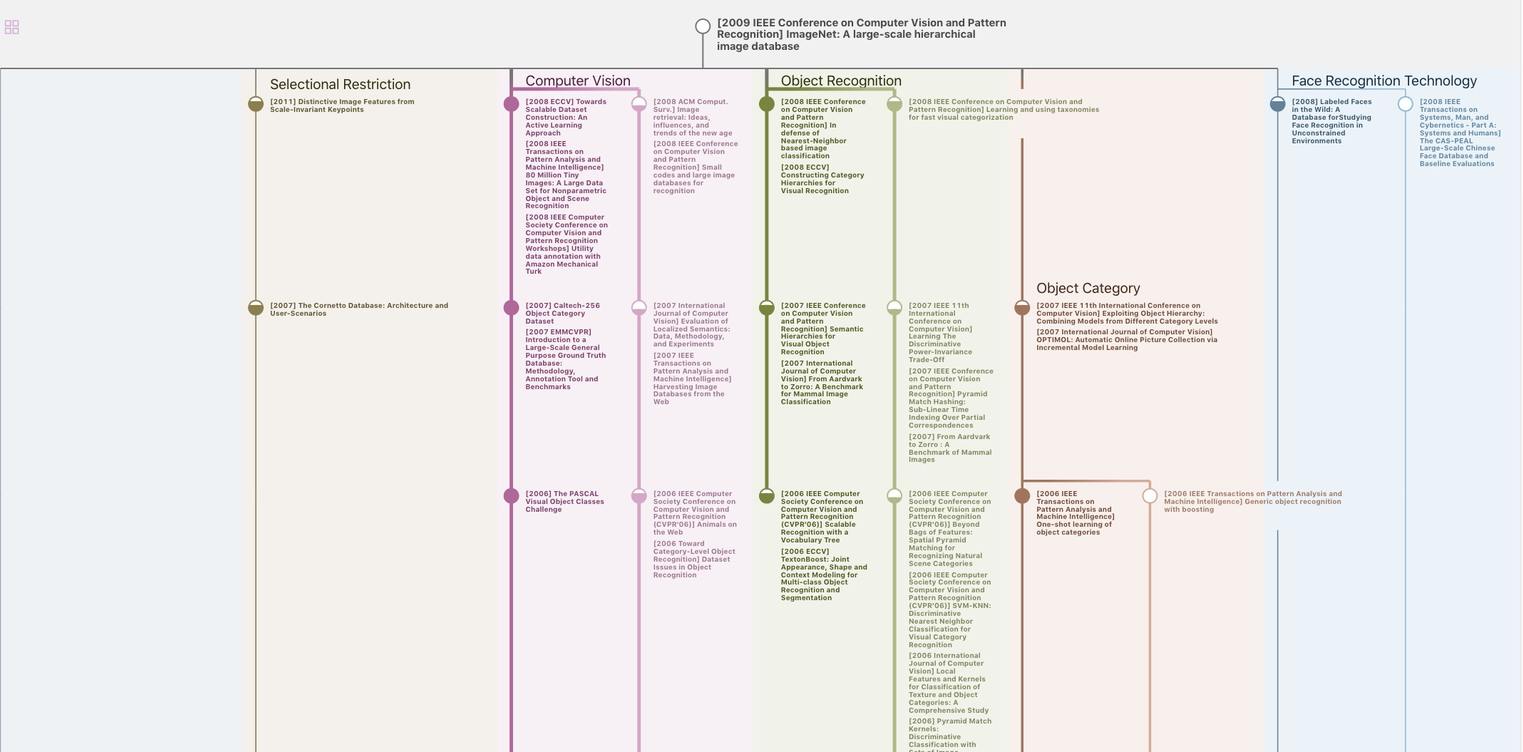
生成溯源树,研究论文发展脉络
Chat Paper
正在生成论文摘要