Mean Subtraction and Mode Selection in Dynamic Mode Decomposition
arxiv(2023)
摘要
Koopman mode analysis has provided a framework for analysis of nonlinear phenomena across a plethora of fields. Its numerical implementation via Dynamic Mode Decomposition (DMD) has been extensively deployed and improved upon over the last decade. We address the problems of mean subtraction and DMD mode selection in the context of finite dimensional Koopman invariant subspaces. Preprocessing of data by subtraction of the temporal mean of a time series has been a point of contention in companion matrix-based DMD. This stems from the potential of said preprocessing to render DMD equivalent to temporal DFT. We prove that this equivalence is impossible in linearly consistent data, when the order of the DMD-based representation of the dynamics exceeds the dimension of the system. Therefore, this parity of DMD and DFT can be indicative of an inadequacy of data, in the sense that the number of snapshots taken is not enough to represent the true dynamics of the system. We then rigorously justify the practice of pruning DMD eigen-values based on the norm of the respective modes. Once a minimum number of time delays has been taken, DMD eigen-values corresponding to DMD modes with low norm are shown to be spurious, and hence must be discarded. When dealing with mean-subtracted data, the above criterion for detecting synthetic eigen-values can be applied if DMD is preceded by time delay embedding.
更多查看译文
关键词
dynamic mode decomposition,mode selection
AI 理解论文
溯源树
样例
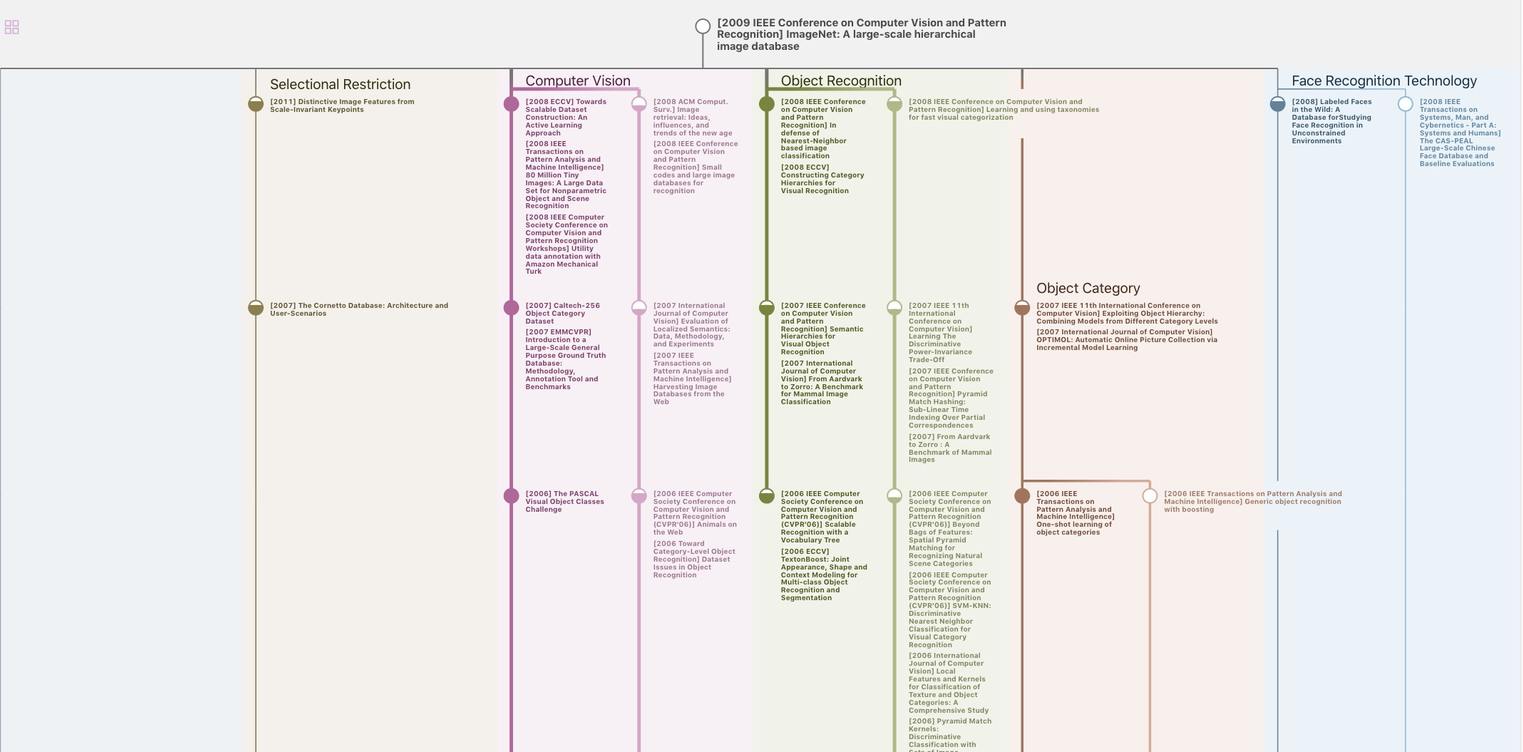
生成溯源树,研究论文发展脉络
Chat Paper
正在生成论文摘要