The synergy of complex event processing and tiny machine learning in industrial IoT
DEBS(2021)
摘要
ABSTRACTFocusing on comprehensive networking, the Industrial Internet-of-Things (IIoT) facilitates efficiency and robustness in factory operations. Various intelligent sensors play a central role, as they generate a vast amount of real-time data that can provide insights into manufacturing. Complex event processing (CEP) and machine learning (ML) have been developed actively in the last years in IIoT to identify patterns in heterogeneous data streams and fuse raw data into tangible facts. In a traditional compute-centric paradigm, the raw field data are continuously sent to the cloud and processed centrally. As IIoT devices become increasingly pervasive, concerns are raised since transmitting such an amount of data is energy-intensive, vulnerable to be intercepted, and subjected to high latency. Decentralized on-device ML and CEP provide a solution where data is processed primarily on edge devices. Thus communications can be minimized. However, this is no mean feat because most IIoT edge devices are resource-constrained with low power consumption. This paper proposes a framework that exploits ML and CEP's synergy at the edge in distributed sensor networks. By leveraging tiny ML and μCEP, we now shift the computation from the cloud to the resource-constrained IIoT devices and allow users to adapt on-device ML models and CEP reasoning rules flexibly on the fly. Lastly, we demonstrate the proposed solution and show its effectiveness and feasibility using an industrial use case of machine safety monitoring.
更多查看译文
AI 理解论文
溯源树
样例
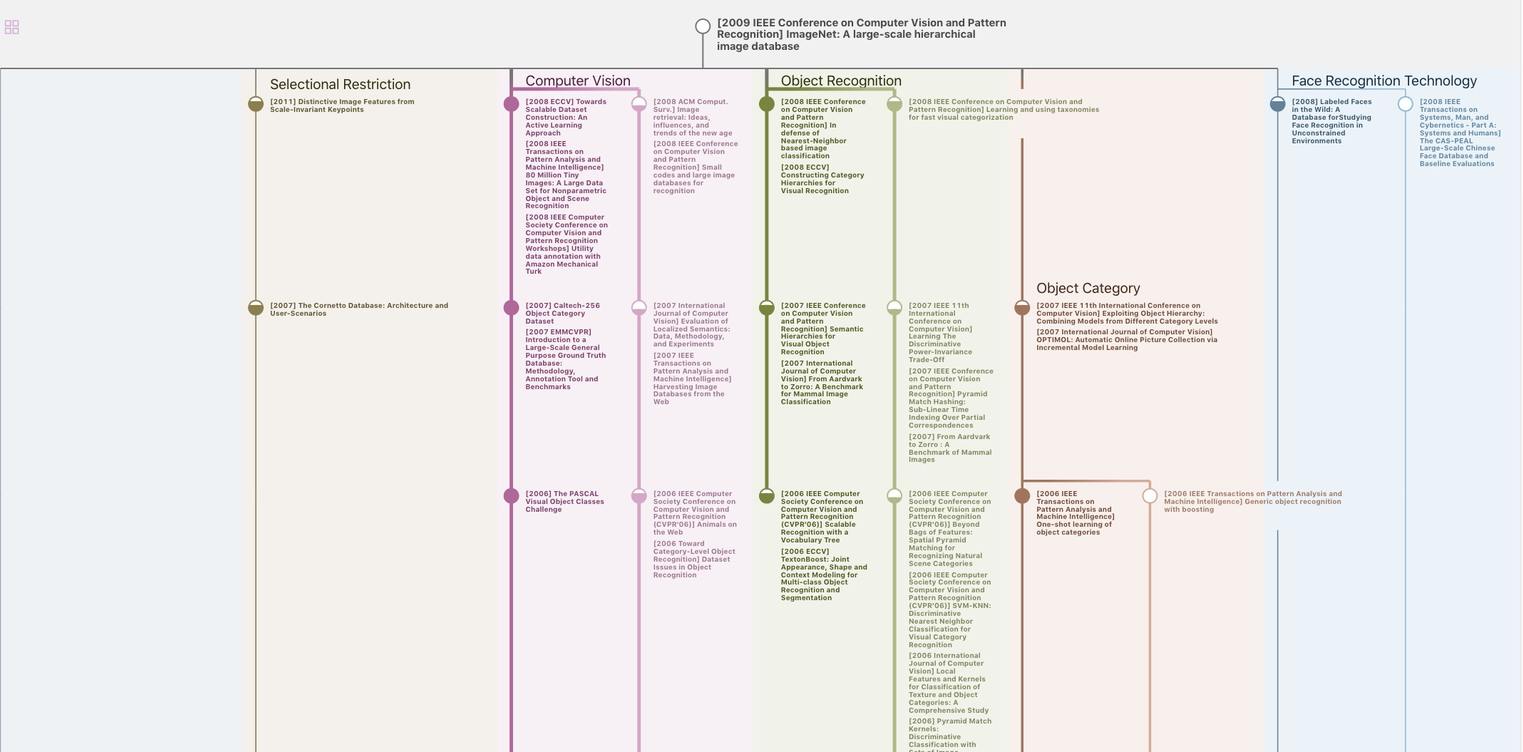
生成溯源树,研究论文发展脉络
Chat Paper
正在生成论文摘要