KCRR: a nonlinear machine learning with a modified genomic similarity matrix improved the genomic prediction efficiency.
BRIEFINGS IN BIOINFORMATICS(2021)
摘要
Nowadays, advances in high-throughput sequencing benefit the increasing application of genomic prediction (GP) in breeding programs. In this research, we designed a Cosine kernel-based KRR named KCRR to perform GP. This paper assessed the prediction accuracies of 12 traits with various heritability and genetic architectures from four populations using the genomic best linear unbiased prediction (GBLUP), BayesB, support vector regression (SVR), and KCRR. On the whole, KCRR performed stably for all traits of multiple species, indicating that the hypothesis of KCRR had the potential to be adapted to a wide range of genetic architectures. Moreover, we defined a modified genomic similarity matrix named Cosine similarity matrix (CS matrix). The results indicated that the accuracies between GBLUP_kinship and GBLUP_CS almost unanimously for all traits, but the computing efficiency has increased by an average of 20 times. Our research will be a significant promising strategy in future GP.
更多查看译文
关键词
kernel ridge regression, Cosine kernel, genomic prediction, support vector regression
AI 理解论文
溯源树
样例
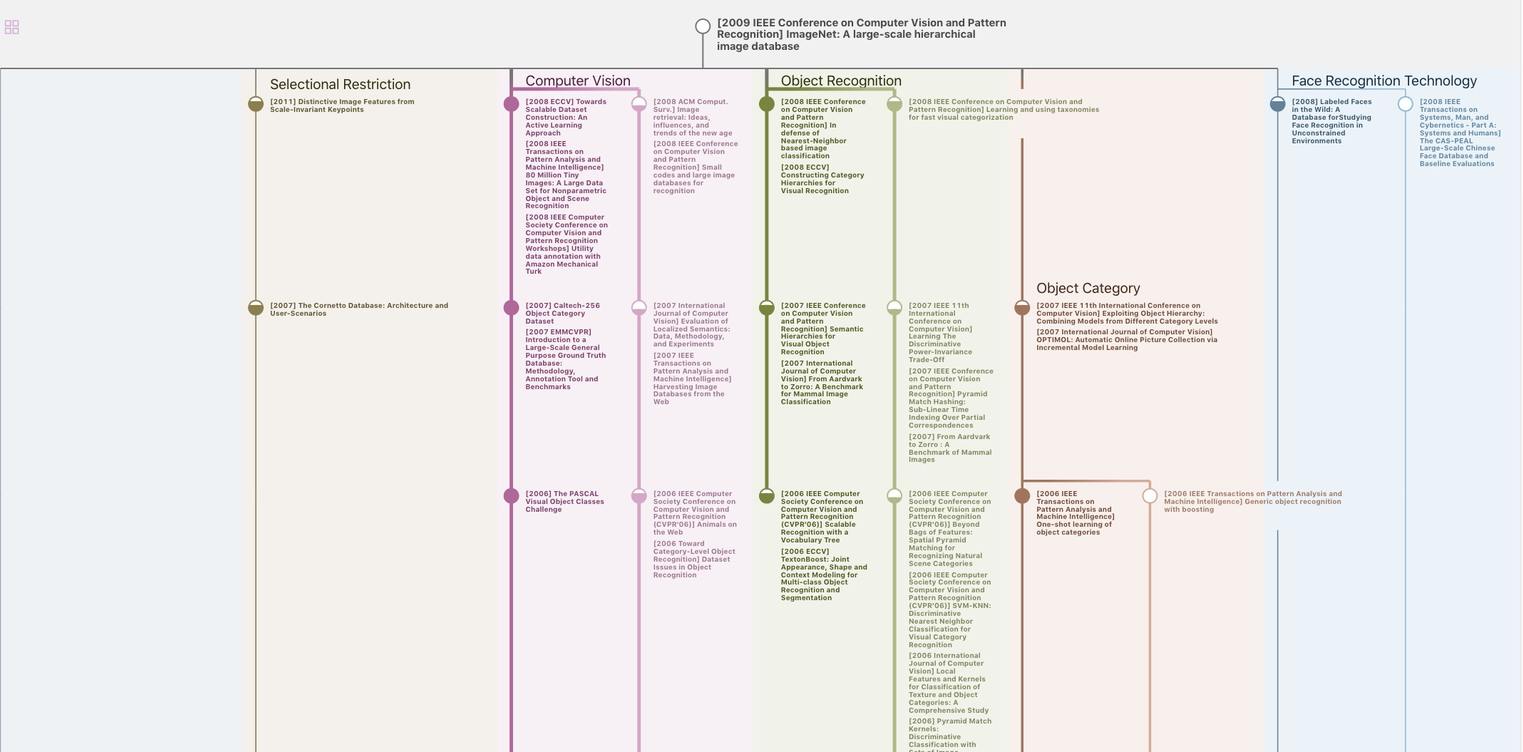
生成溯源树,研究论文发展脉络
Chat Paper
正在生成论文摘要