The effect of data aggregation on dispersion estimates in count data models
INTERNATIONAL JOURNAL OF BIOSTATISTICS(2022)
摘要
For the modelling of count data, aggregation of the raw data over certain subgroups or predictor configurations is common practice. This is, for instance, the case for count data biomarkers of radiation exposure. Under the Poisson law, count data can be aggregated without loss of information on the Poisson parameter, which remains true if the Poisson assumption is relaxed towards quasi-Poisson. However, in biodosimetry in particular, but also beyond, the question of how the dispersion estimates for quasi-Poisson models behave under data aggregation have received little attention. Indeed, for real data sets featuring unexplained heterogeneities, dispersion estimates can increase strongly after aggregation, an effect which we will demonstrate and quantify explicitly for some scenarios. The increase in dispersion estimates implies an inflation of the parameter standard errors, which, however, by comparison with random effect models, can be shown to serve a corrective purpose. The phenomena are illustrated by gamma-H2AX foci data as used for instance in radiation biodosimetry for the calibration of dose-response curves.
更多查看译文
关键词
heterogeneity, overdispersion, quasi-Poisson, radiation biomarker, random effect
AI 理解论文
溯源树
样例
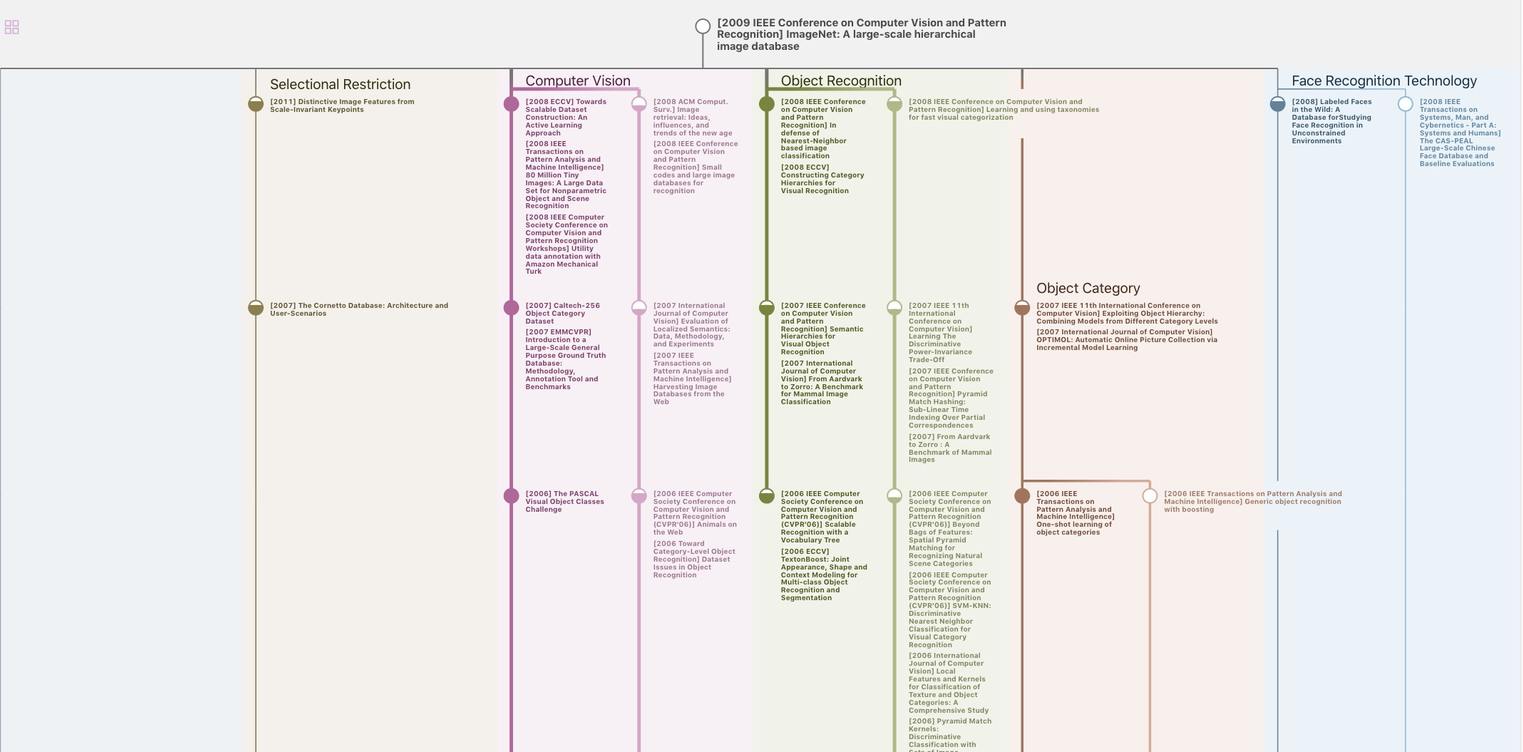
生成溯源树,研究论文发展脉络
Chat Paper
正在生成论文摘要