Srgat: Single Image Super-Resolution With Graph Attention Network
IEEE TRANSACTIONS ON IMAGE PROCESSING(2021)
摘要
Deep neural networks have demonstrated remarkable reconstruction for single-image super-resolution (SISR). However, most existing CNN-based SISR methods directly learn the relation between low-resolution (LR) and high-resolution (HR) images, neglecting to explore the recurrence of internal patches, hence hindering the representational power of CNNs. In this paper, we propose a novel single image Super-Resolution network based on Graph ATtention network (SRGAT) to make full use of the internal patch-recurrence in a natural image. The proposed model employs a feature mapping block with a recurrent structure to refine low-level representations with high-level information. Especifically, the feature mapping block contains a parallel graph similarity branch and a content branch, where the graph similarity branch aims at exploiting the similarity and symmetry across different image patches in low-resolution feature space and provides additional priors for the content branch to enhance texture details. Specifically, we consider the internal patch-recurrence of an image by constructing a graph network on image feature patches. In this way, the information from neighboring patches can be interacted using graph attention network (GAT) to help it recover additional textures, which complements the textures learned from the content branch. Extensive quantitative and qualitative evaluations on five benchmark datasets demonstrate that the proposed algorithm performs favorably against the state-of-the-art super-resolution methods.
更多查看译文
关键词
Superresolution, Image reconstruction, Feature extraction, Task analysis, Image edge detection, Correlation, Computational modeling, Single image super-resolution, graph attention network, patch-recurrence
AI 理解论文
溯源树
样例
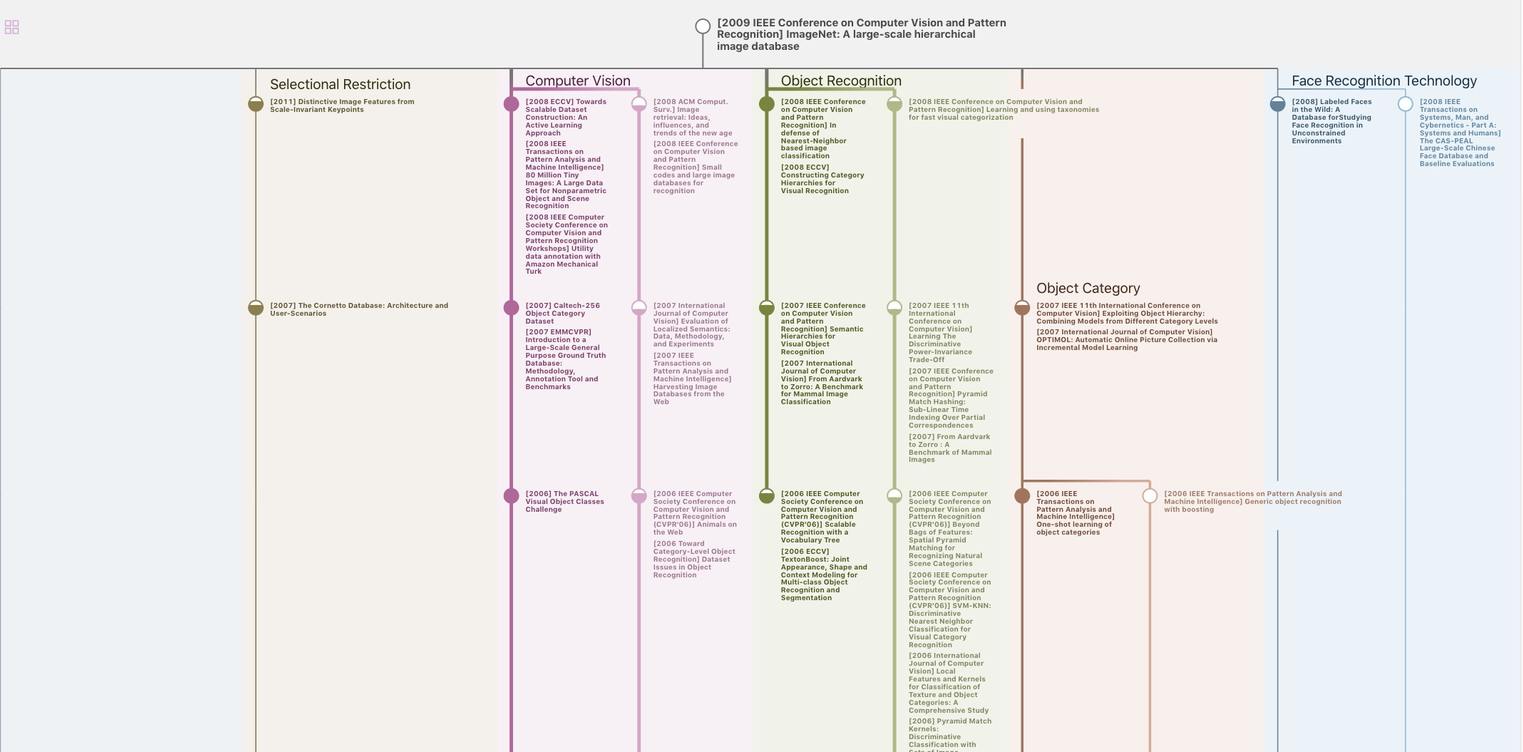
生成溯源树,研究论文发展脉络
Chat Paper
正在生成论文摘要