What And How? Jointly Forecasting Human Action And Pose
2020 25TH INTERNATIONAL CONFERENCE ON PATTERN RECOGNITION (ICPR)(2020)
摘要
Forecasting human actions and motion trajectories address the problem of predicting what a person is going to do next and how they will perform it. This is crucial in a wide range of applications, such as assisted living and future co-robotic settings. We propose to simultaneously learn actions and action-related human motion dynamics while existing works perform them independently. This paper presents a method to jointly forecast categories of human action and skeletal joint pose, allowing the two tasks to reinforce each other. As a result, our system can predict future actions and the motion trajectories that will result. To achieve this, we define a task of joint action classification and pose regression. We employ a sequence to sequence encoder-decoder model combined with multi-task learning to forecast future actions and poses progressively before the action happens. Experimental results on two public datasets, IkeaDB and OAD, demonstrate the effectiveness of the proposed method.
更多查看译文
关键词
pose regression,multitask learning,human motion dynamics,skeletal joint pose,joint action classification,human action forecasting,motion trajectories forecasting,sequence to sequence encoder-decoder model
AI 理解论文
溯源树
样例
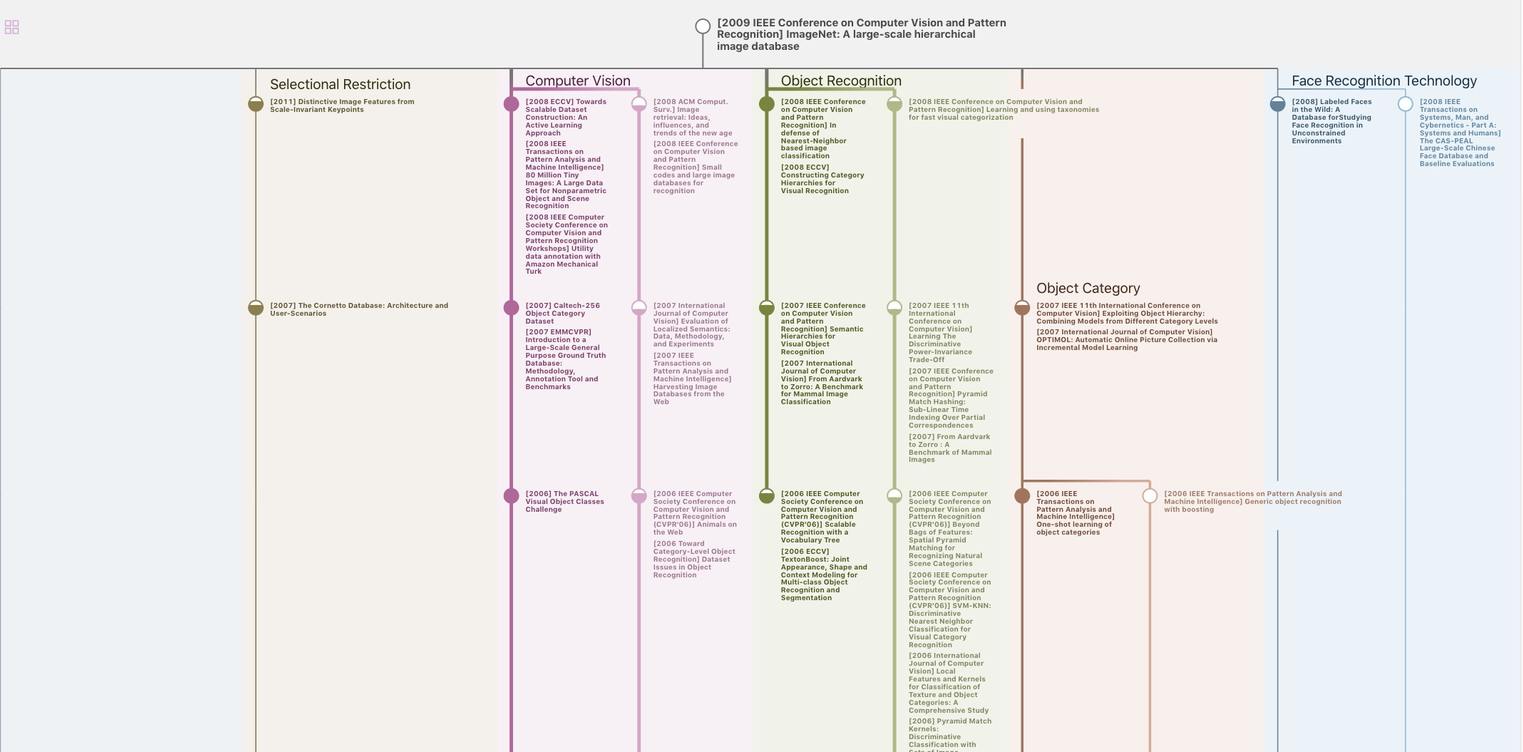
生成溯源树,研究论文发展脉络
Chat Paper
正在生成论文摘要