MEAN: Multi - Element Attention Network for Scene Text Recognition
2020 25TH INTERNATIONAL CONFERENCE ON PATTERN RECOGNITION (ICPR)(2021)
摘要
Scene text recognition is a challenging problem due to the wide variances in contents, styles, orientations, and image quality of text instances in natural scene images. To learn the intrinsic representation of scene texts, a novel multi-element attention (MEA) mechanism is proposed to exploit geometric structures from local to global levels in feature maps extracted from a scene text image. The MEA mechanism is a generalized form of self-attention technique. The elements in feature maps are taken as the nodes of an undirected graph, and three kinds of adjacency matrices are designed to aggregate information at local, neighborhood and global levels before calculating the attention weights. A multi-element attention network (MEAN) is implemented, which includes a CNN for feature extraction, an encoder with MEA mechanism and a decoder for predicting text codes. Orientational positional encoding is added to feature maps output by the CNN, and a feature vector sequence transformed from the feature maps is used as the input of the encoder. Experimental results show that MEAN has achieved state-of-the-art or competitive performance on seven public English scene text datasets (IIITSk, SVT, IC03, IC13, IC15, SVTP, and CUTE). Further experiments have been conducted on a selected subset of the RCTW Chinese scene text dataset, demonstrating that MEAN can handle horizontal, vertical, and irregular scene text samples.
更多查看译文
关键词
attention weights,multielement attention network,feature extraction,MEA mechanism,text codes,orientational positional encoding,feature maps output,feature vector sequence,seven public English scene text datasets,RCTW Chinese scene text dataset,irregular scene text samples,multi- element attention network,scene text recognition,image quality,text instances,natural scene images,scene texts,novel multielement attention mechanism,global levels,scene text image,self-attention technique
AI 理解论文
溯源树
样例
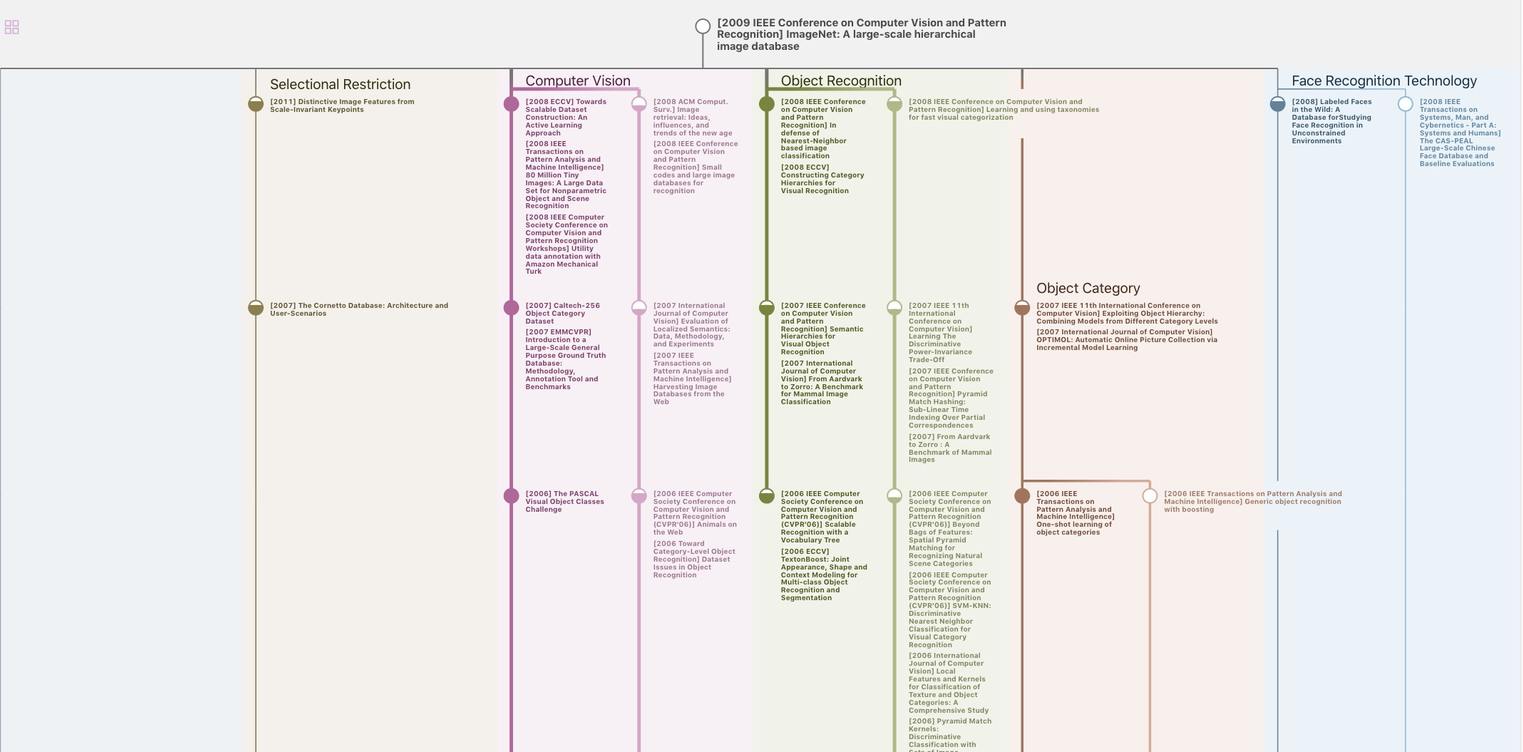
生成溯源树,研究论文发展脉络
Chat Paper
正在生成论文摘要