TAAN: Task-Aware Attention Network for Few-shot Classification
2020 25TH INTERNATIONAL CONFERENCE ON PATTERN RECOGNITION (ICPR)(2021)
摘要
Few-shot classification aims to recognize unlabeled samples from unseen classes given only a few labeled samples. Current approaches of few-shot learning usually employ a metric-learning framework to learn a feature similarity comparison between a query (test) example and the few support (training) examples. However, these approaches all extract features from samples independently without looking at the entire task as a whole, and so fail to provide an enough discrimination to features. Moreover, the existing approaches lack the ability to select the most relevant features for the task at hand. In this work, we propose a novel algorithm called
Task-Aware
Attention
Network
(TAAN) to address the above problems in few-shot classification. By inserting a
Task-Relevant
Channel
Attention
Module
into metric-based few-shot learners, TAAN generates channel attentions for each sample by aggregating the context of the entire support set and identifies the most relevant features for similarity comparison. The experiment demonstrates that TAAN is competitive in overall performance comparing to the recent state-of-the-art systems and improves the performance considerably over baseline systems on both mini-ImageNet and tiered - ImageNet benchmarks.
更多查看译文
关键词
few-shot classification, metric learning, task-aware, task-relevant channel attention
AI 理解论文
溯源树
样例
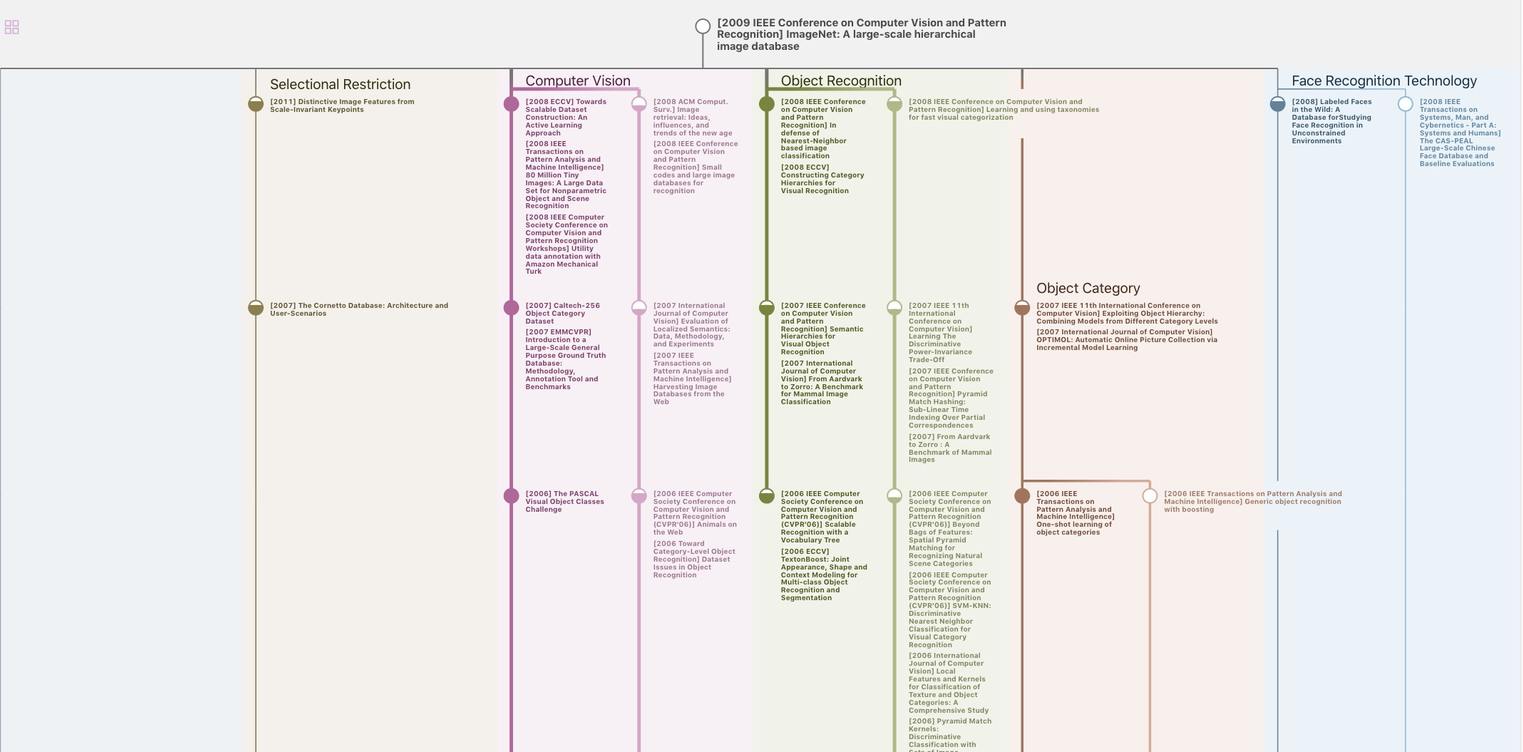
生成溯源树,研究论文发展脉络
Chat Paper
正在生成论文摘要