A Bayesian Deep Cnn Framework For Reconstructing K-T-Undersampled Resting-Fmri
2020 25TH INTERNATIONAL CONFERENCE ON PATTERN RECOGNITION (ICPR)(2020)
摘要
Undersampled reconstruction in resting functional magnetic resonance imaging (R-fMRI) holds the potential to enable higher spatial resolution in brain R-fMRI without increasing scan duration. We propose a novel approach to reconstruct k-t undersampled R-fMRI relying on a deep convolutional neural network (CNN) framework. The architecture of our CNN framework comprises a novel scheme for R-fMRI reconstruction that jointly learns two multilayer CNN components for (i) explicitly filling in missing k-space data, using acquired data in frequency-temporal neighborhoods, and (ii) image quality enhancement in the spatiotemporal domain. The architecture sandwiches the Fourier transformation from the frequency domain to the spatial domain between the two aforementioned CNN components, during, both, CNN learning and inference. We propose four methods within our framework, including a Bayesian CNN that produces uncertainty maps indicating the per-voxel (and per-timepoint) confidence in the blood oxygenation level dependent (BOLD) time-series reconstruction. Results on brain R-fMRI show that our CNN framework improves over the state of the art, quantitatively and qualitatively, in terms of the connectivity maps for three cerebral functional networks.
更多查看译文
关键词
R-fMRI reconstruction, k-t undersampling, deep convolutional neural network, k-space filling, image quality enhancement, Bayesian modeling, uncertainty
AI 理解论文
溯源树
样例
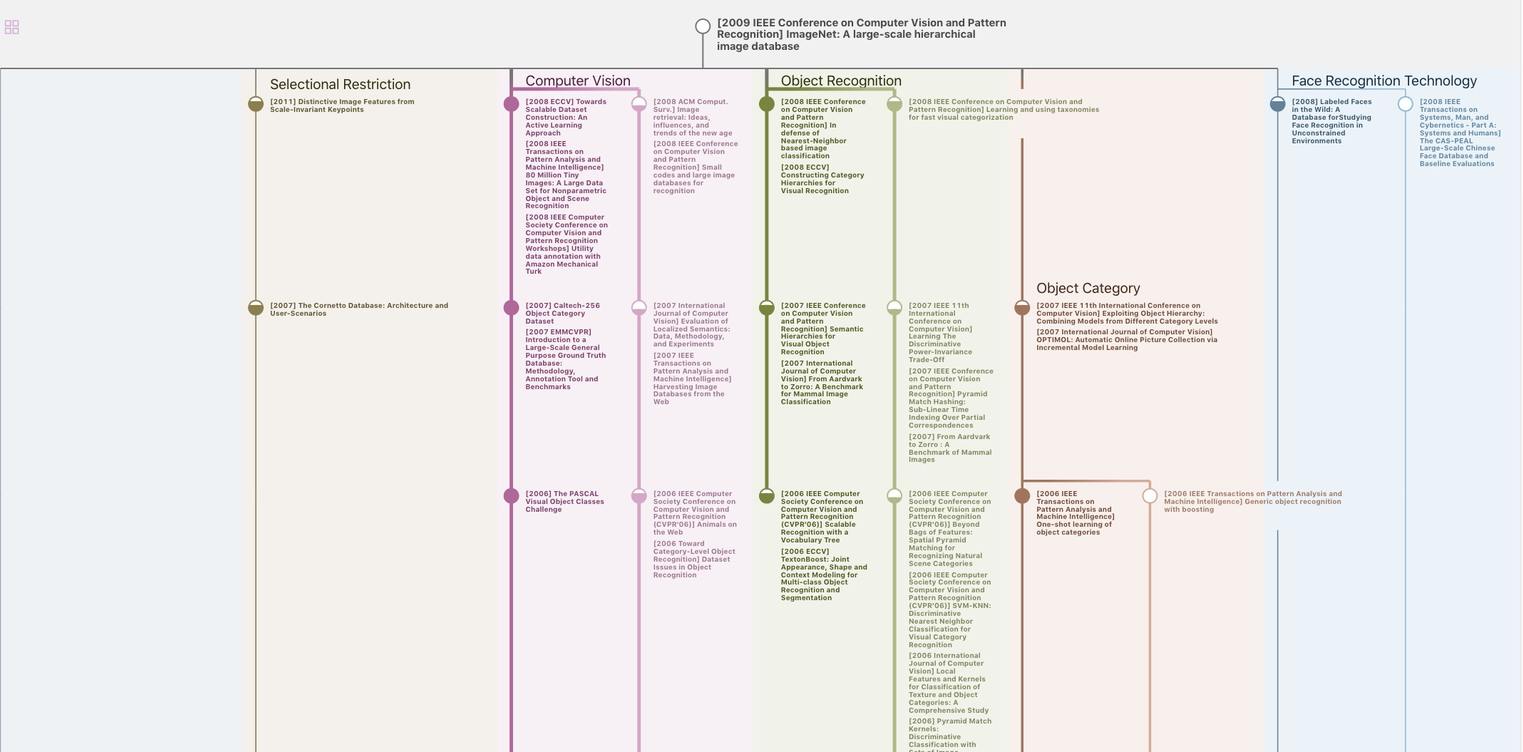
生成溯源树,研究论文发展脉络
Chat Paper
正在生成论文摘要