Improving Mix-And-Separate Training In Audio-Visual Sound Source Separation With An Object Prior
2020 25TH INTERNATIONAL CONFERENCE ON PATTERN RECOGNITION (ICPR)(2020)
摘要
The performance of an audio-visual sound source separation system is determined by its ability to separate audio sources given the images of the sources and the audio mixture. The goal of this study is to investigate the ability to learn the mapping between the sounds and the images of instruments in the self-supervisied mix-and-seperate training paradigm used by state-of-the-art audio-visual sound source separation methods. Theoretical and empirical analyses illustrate that the self-supervised mix-and-separate training does not automatically learn the 1-to-1 correspondence between visual and audio signals, leading to low audio-visual object classification accuracy. Based on this analysis, a weakly-supervised method called Object-Prior is proposed and evaluated on two audio-visual datasets. The experimental results show that the Object-Prior method outperforms state-of-the-art baselines in the audio-visual sound source separation task. It is also more robust against asynchronized data, where the frame and the audio do not come from the same video, and recognizes musical instruments based on their sound with higher accuracy. This indicates that learning the 1-to-1 correspondence between visual and audio features of an instrument improves the effectiveness of audio-visual sound source separation.
更多查看译文
关键词
audio-visual sound source separation system,audio sources,audio mixture,state-of-the-art audio-visual sound source separation methods,audio-visual object classification accuracy,audio-visual datasets,Object-Prior method,audio-visual sound source separation task,self-supervisied mix-and-seperate training paradigm
AI 理解论文
溯源树
样例
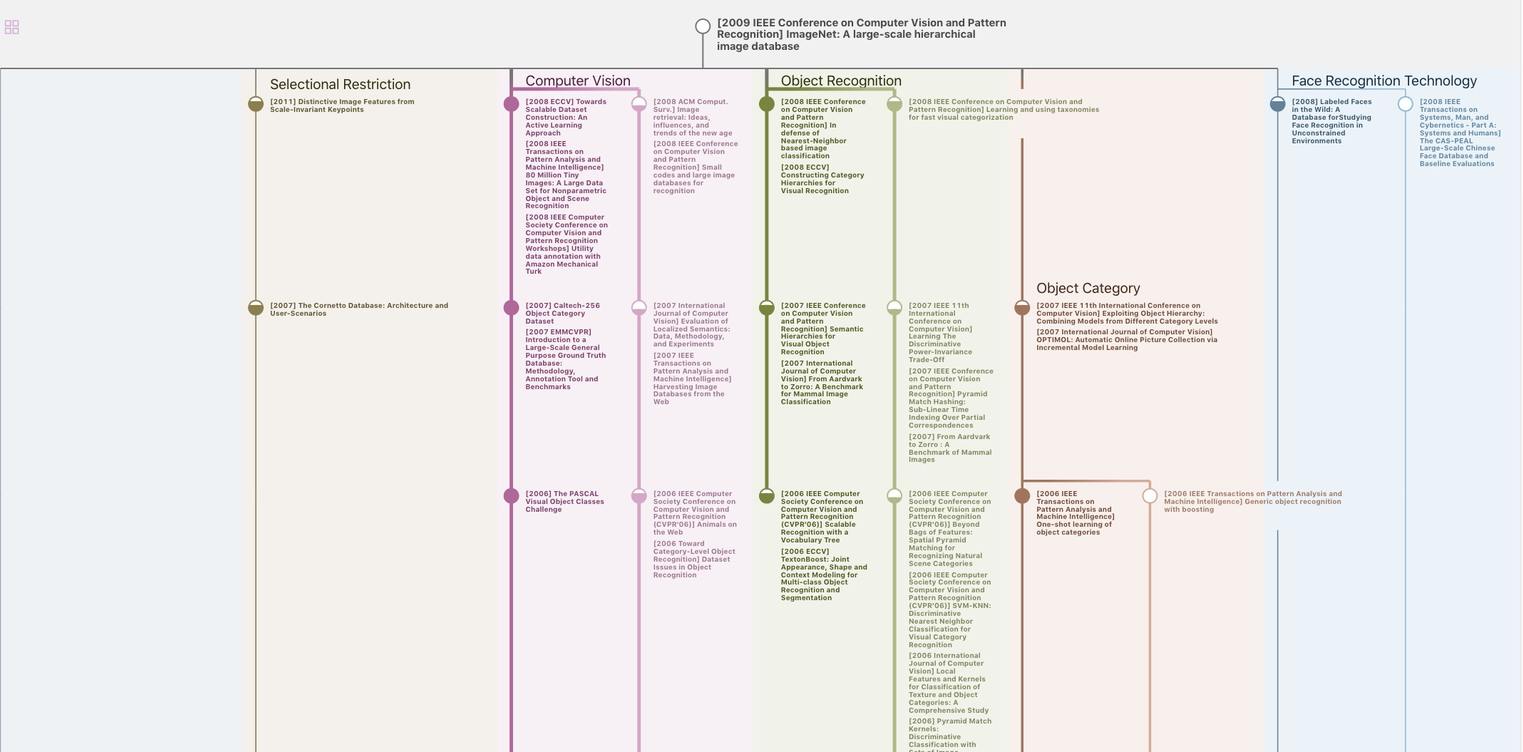
生成溯源树,研究论文发展脉络
Chat Paper
正在生成论文摘要