Longitudinal Feature Selection and Feature Learning for Parkinson's Disease Diagnosis and Prediction
2020 25TH INTERNATIONAL CONFERENCE ON PATTERN RECOGNITION (ICPR)(2021)
摘要
Parkinson's disease (PD) is an irreversible neurodegenerative disease that seriously affects patients' lives. To provide patients with accurate treatment in time and to reduce deterioration of the disease, it is critical to have an early diagnosis of PD and accurate clinical score predictions. Different from previous studies on PD, most of which only focus on feature selection methods, we propose a network combining joint learning from multiple modalities and relations (JLMMR) with sparse nonnegative autoencoder (SNAE) to further enhance the ability of feature expression. We first preprocess and extract features of the modal neuroimaging data with multiple time points. To extract discriminative and informative features from longitudinal data, we apply JLMMR method for feature selection to avoid over-fitting issues. We further exploit SNAE to learn longitudinal discriminative features for joint disease diagnosis and obtain clinical score predictions. Extensive experiments on the publicly available Parkinson's Progression Markers Initiative (PPMI) dataset show the proposed method produces promising classification and prediction performance, which outperforms state-of-the-art methods as well.
更多查看译文
关键词
Parkinson's disease, Longitudinal multi-modal data, sparse nonnegative autoencoder, multiple modalities and relation Classification and Regression
AI 理解论文
溯源树
样例
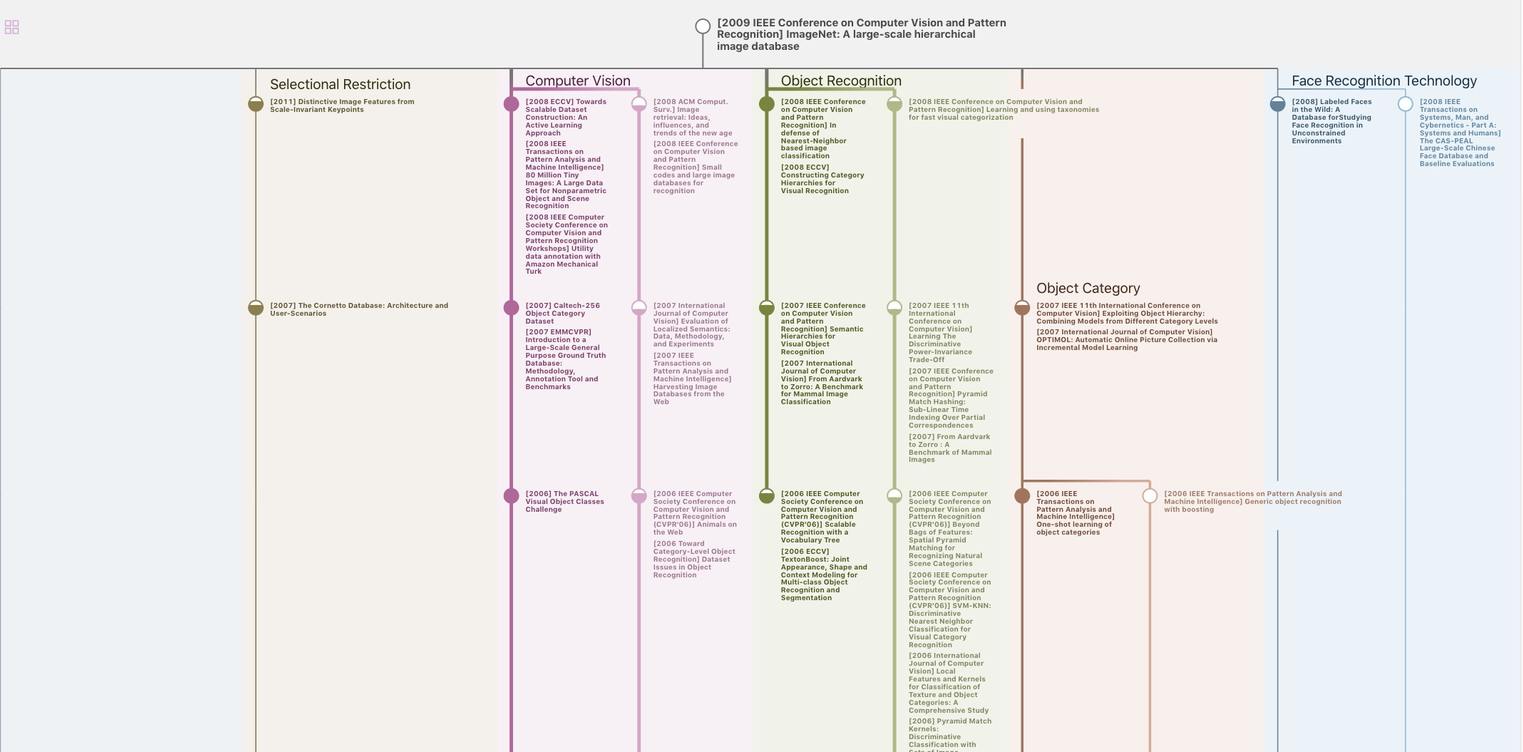
生成溯源树,研究论文发展脉络
Chat Paper
正在生成论文摘要