Hierarchical Classification With Confidence Using Generalized Logits
2020 25TH INTERNATIONAL CONFERENCE ON PATTERN RECOGNITION (ICPR)(2020)
摘要
We present a bottom-up approach to hierarchical classification based on posteriors conditioned with logits. Beginning with the output logits for a set of terminal labels from a base classifier, an initial hypothesis is repeatedly generalized (softened) to a weaker label until a particular confidence measure is achieved. As conditioning the probabilistic model with the full set of terminal logits quickly becomes intractable for large label sets, we propose an alternative approach employing "generalized logits" spanning relevant hypotheses within the label hierarchy. Experimental results are compared with related methods on multiple datasets and base classifiers. The proposed approach provides an efficient and effective hierarchical classification framework with monotonic, non-decreasing inference behavior.
更多查看译文
关键词
generalized logits,output logits,terminal labels,base classifier,initial hypothesis,confidence measure,terminal logits,label sets,label hierarchy,multiple datasets,base classifiers,hierarchical classification framework
AI 理解论文
溯源树
样例
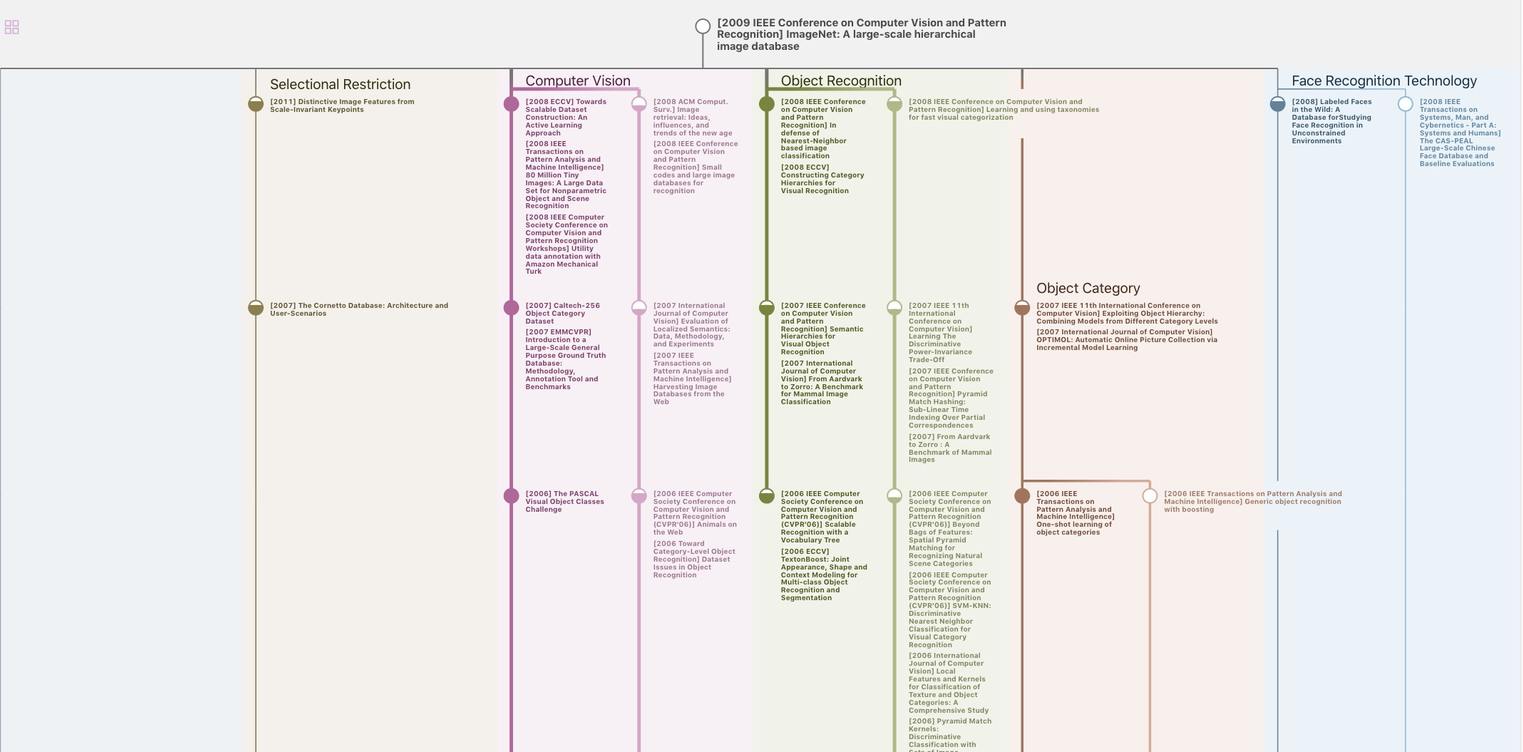
生成溯源树,研究论文发展脉络
Chat Paper
正在生成论文摘要