Class-Incremental Learning With Topological Schemas Of Memory Spaces
2020 25TH INTERNATIONAL CONFERENCE ON PATTERN RECOGNITION (ICPR)(2020)
摘要
Class-incremental learning (CIL) aims to incrementally learn a unified classifier for new classes emerging, which suffers from the catastrophic forgetting problem. To alleviate forgetting and improve the recognition performance, we propose a novel CIL framework, named the topological schemas model (TSM). TSM consists of a Gaussian mixture model arranged on 2D grids (2D-GMM) as the memory of the learned knowledge. To train the 2D-GMM model, we develop a novel competitive expectation-maximization (CEM) method, which contains a global topology embedding step and a local expectation-maximization finetuning step. Meanwhile, we choose the image samples of old classes that have the maximum posterior probability with respect to each Gaussian distribution as the episodic points. When finetuning for new classes, we propose the memory preservation loss ( MPL) term to ensure episodic points still have maximum probabilities with respect to the corresponding Gaussian distribution. MPL preserves the distribution of 2D-GMM for old knowledge during incremental learning and alleviates catastrophic forgetting.Comprehensive experimental evaluations on two popular CIL benchmarks CIFAR100 and subImageNet demonstrate the superiority of our TSM.
更多查看译文
关键词
Continual learning, Class-incremental learning, Gaussian Mixture Model, 2D-GMM, Topological Schemas Model
AI 理解论文
溯源树
样例
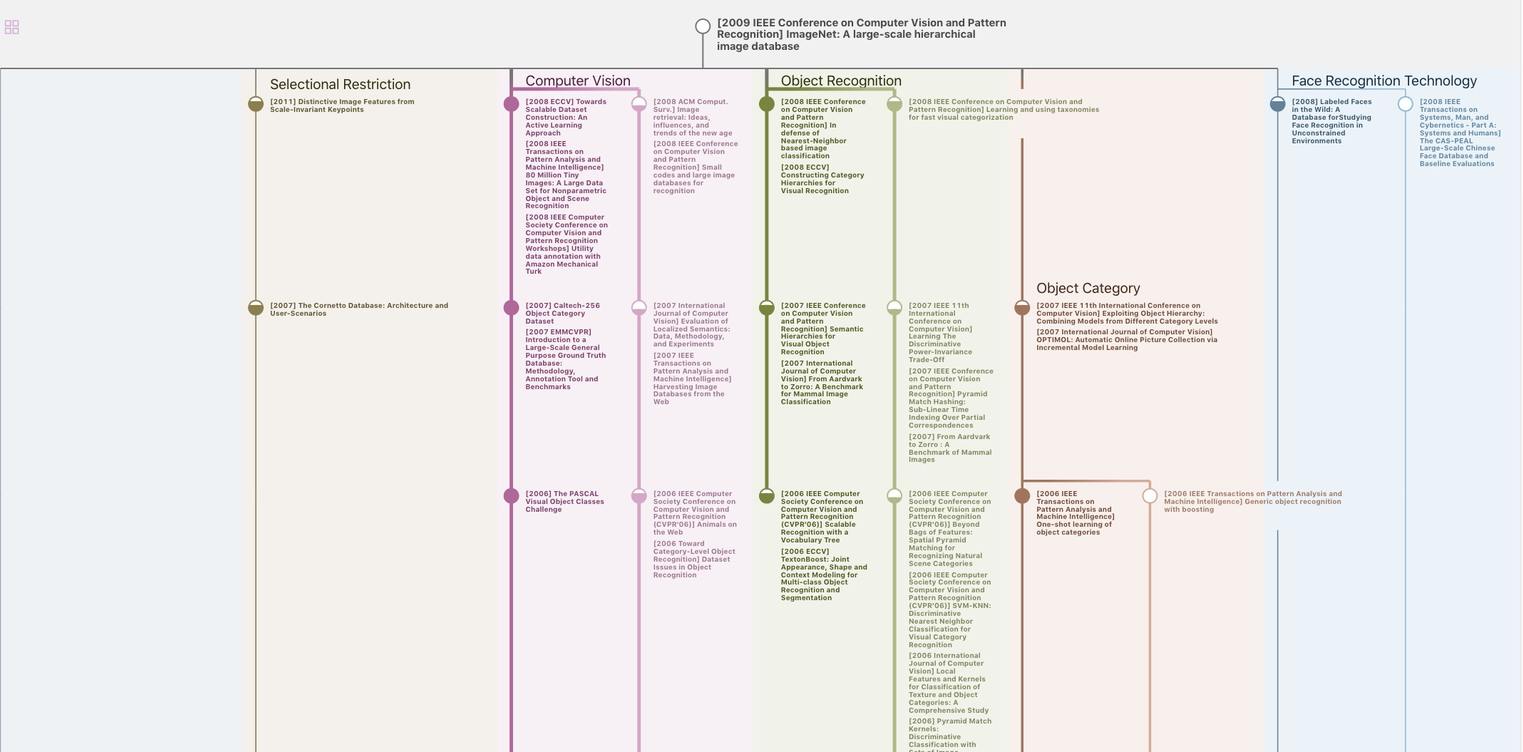
生成溯源树,研究论文发展脉络
Chat Paper
正在生成论文摘要