A Double Channel CNN-LSTM Model for Text Classification.
HPCC/DSS/SmartCity(2020)
摘要
The CNN-LSTM model has the advantages of combining Convolutional Neural Network (CNN) and Long-Short Term Memory (LSTM). It can perform timing analysis while extracting abstract features. It is widely used in Computer Vision and Natural Language Processing (NLP) fields and has achieved satisfactory results. However, for a large number of samples of complex text data, especially for words with ambiguous meanings, the word-level CNN-LSTM model is insufficient. Therefore, in order to solve this issue, this paper presents an improved Double Channel (DC) mechanism as a significant enhancement to CNN-LSTM. In this DC mechanism, two channels are used to receive word-level and char-level embedding respectively, at the same time. Hybrid Attention is proposed to combine the current time output with the current time unit state, and then using Attention to calculate the weight. By calculating the probability distribution of each timestep input data weight, the weight score is obtained, and then weighted summation is performed, and the data input by each timestep is subjected to trade-off learning to improve the generalization ability of the model learning. After experimental comparison, the DC CNN-LSTM model proposed in this paper has significantly superior accuracy and F1-score compared with the basic CNN-LSTM model.
更多查看译文
关键词
CNN,LSTM,Text Classification,Double Channel,Probability Distributions
AI 理解论文
溯源树
样例
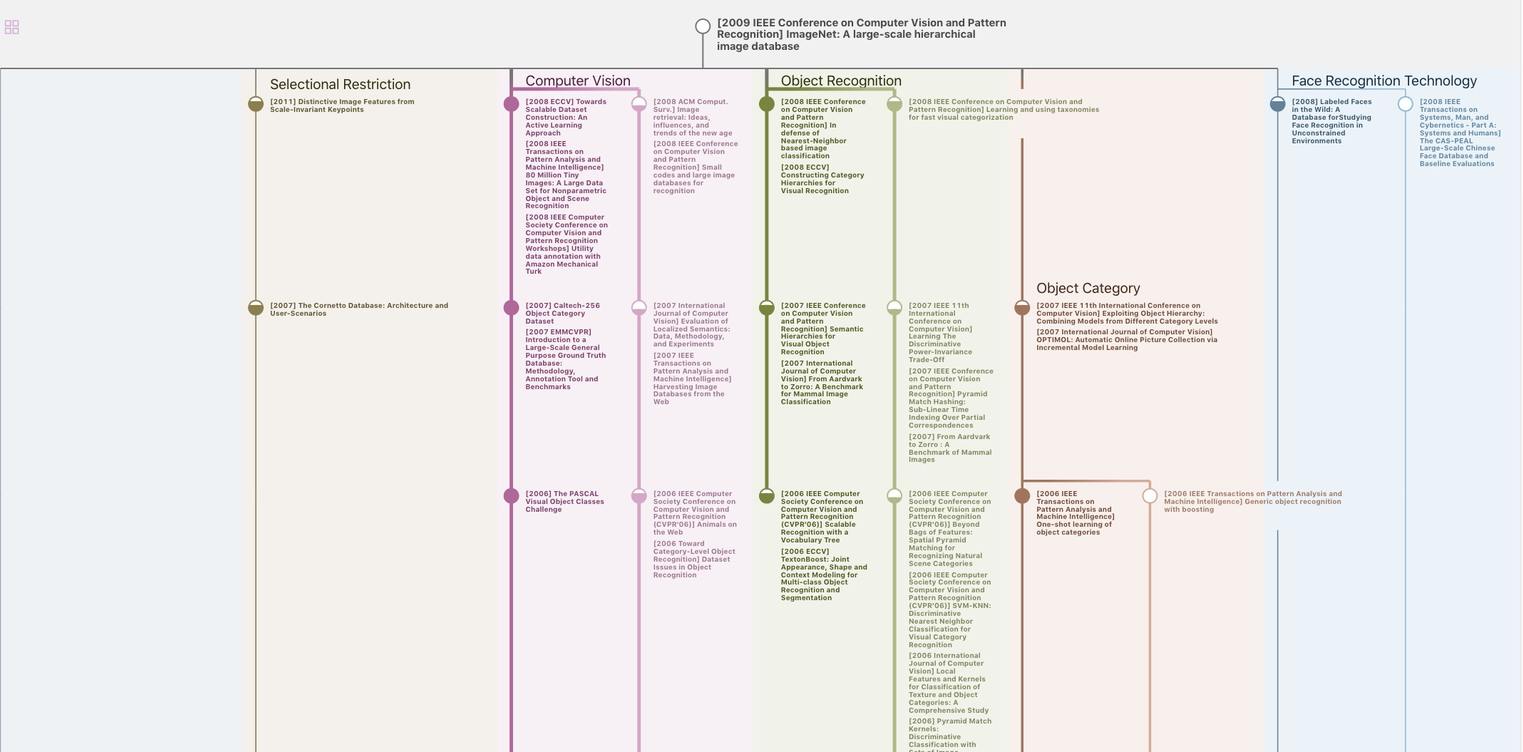
生成溯源树,研究论文发展脉络
Chat Paper
正在生成论文摘要