Towards Semantic Travel Behavior Prediction for Private Car Users.
HPCC/DSS/SmartCity(2020)
摘要
The urban private car as convenient transportation plays an essential role in daily human life, which accordingly produces massive trajectory data by built-in GPS tracking devices. These data offer a new opportunity to mine and explore travel behavior for private car users. Existing works mining travel behavior mainly focus on modeling the sequential contexts while seldom considering the semantic information of the travel behavior, which led to a shallow understanding of users’ travel regularity. To capture valuable information on users’ travel mode, we design a semantic-aware method named as Semantic Long Short-Term Memory(Sem-LSTM). Specifically, we exploit an LSTM network as the foundation of a unified travel behavior prediction framework and introduce two types of semantic information, including area of interest (AOI) properties and user interests. We aim to explore individual travel behavior for a single private car user and conduct extensive experiments on real-life private car datasets. The experimental results demonstrate that Sem-LSTM is very suitable for capturing semantic content and improve prediction performance on private car users. In detail, for the travel behavior prediction, achieve average prediction accuracy of 0.82, recall of 0.80 and F1-score of 0.81.
更多查看译文
关键词
Private car,Location prediction,Semantic information,Sequential contexts,Long Short-Term Memory
AI 理解论文
溯源树
样例
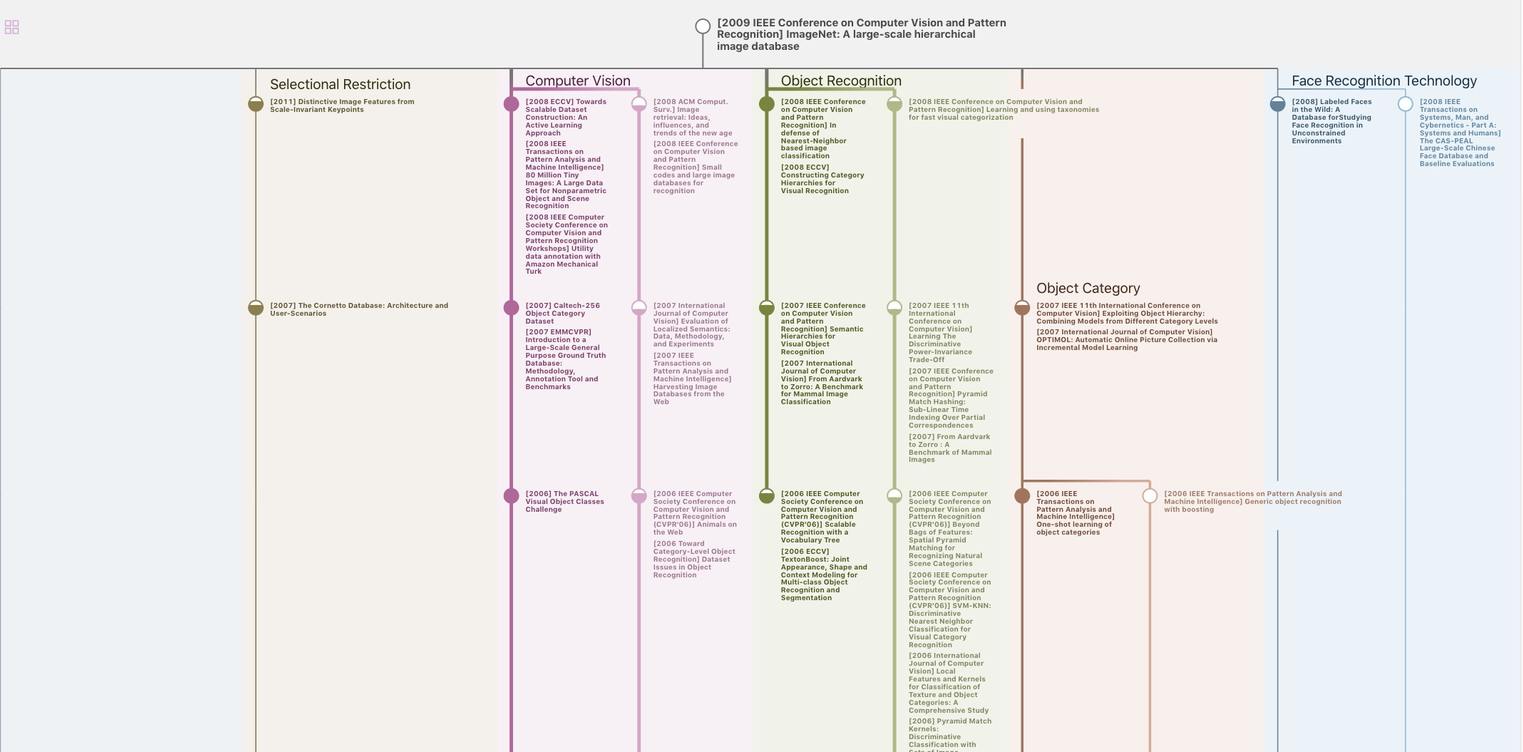
生成溯源树,研究论文发展脉络
Chat Paper
正在生成论文摘要