D2D-Assisted Federated Learning in Mobile Edge Computing Networks
2021 IEEE WIRELESS COMMUNICATIONS AND NETWORKING CONFERENCE (WCNC)(2021)
摘要
With the proliferation of edge intelligence and the breakthroughs in machine learning, Federated Learning (FL) is capable of learning a shared model across several edge devices by preserving their private data from being exposed to external adversaries. However, the distributed architecture of FL naturally introduces communication between the central parameter server and the distributed learning nodes. The huge communication cost poses a challenge to practical FL, especially for FL in mobile edge computing (MEC) networks. Existing communication-efficient FL systems predominantly optimize their intrinsic learning process and are not concerned with the implications on the network. In this paper we propose a FL scheme that leverages Device-to-Device (D2D) communication (hence called D2D-FedAvg) and is suitable for mobile edge networks. D2D-FedAvg creates a two-tier learning model where D2D learning groups communicate their results as a single entity to the MEC server leading to traffic reduction. We propose the schemes for D2D grouping, master UE selection, and also D2D exit in the learning process and then form a complete D2D-assisted federated averaging algorithm. Via extensive simulations on the Federated Extended MNIST dataset, the feasibility and convergence of D2D-FedAvg scheme are evaluated. Our results show that D2D-FedAvg lowers the communication cost relative to the typical Federated Averaging (FedAvg) in cellular networks as the number of users is increased (for 100 cellular users 37% traffic reduction), while keeping the same learning accuracy with FedAvg across the board.
更多查看译文
关键词
Edge Intelligence, Federated Learning, D2D, MEC
AI 理解论文
溯源树
样例
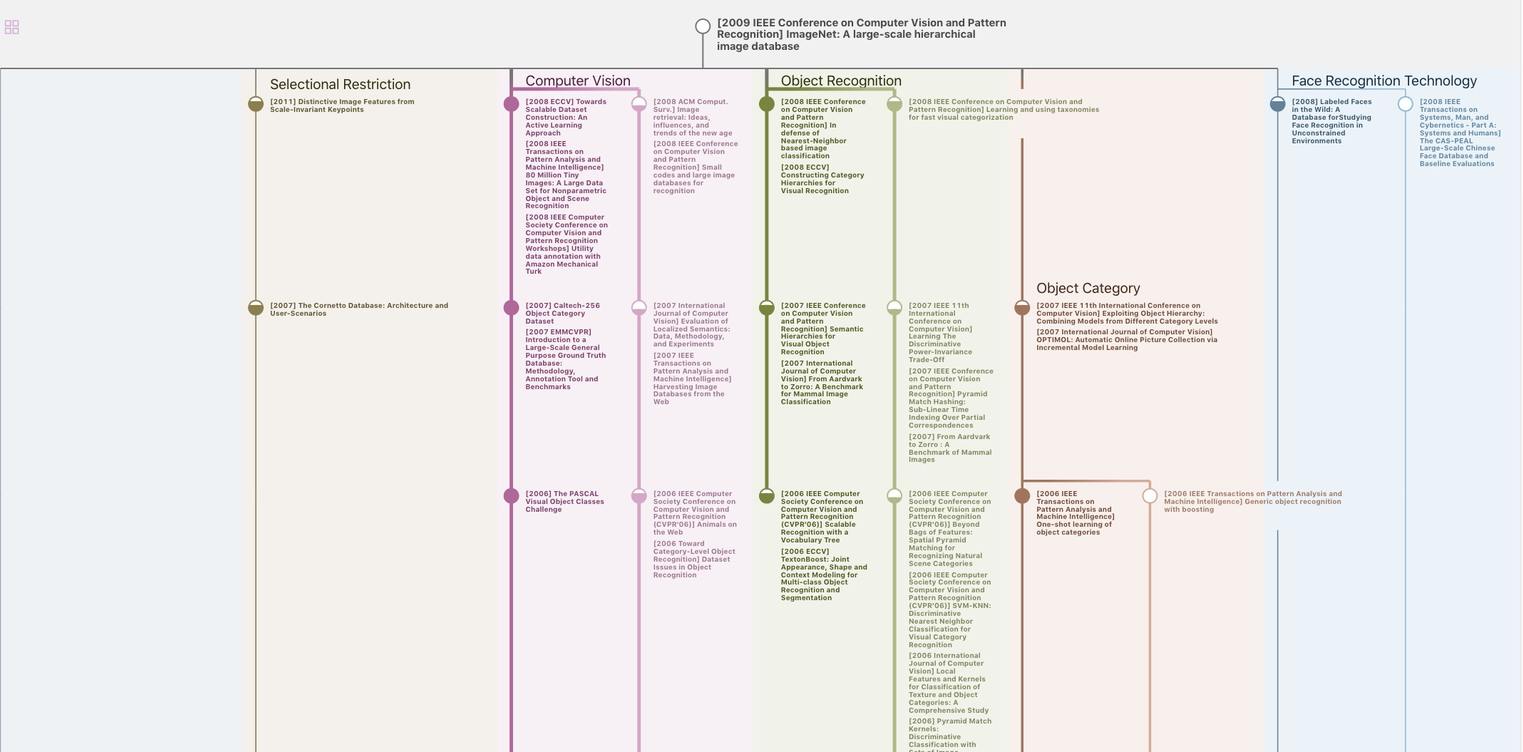
生成溯源树,研究论文发展脉络
Chat Paper
正在生成论文摘要