Dual Part-pooling Attentive Networks for Session-based Recommendation
Neurocomputing(2021)
摘要
Session-based recommendation is proposed to predict user preferences in a short anonymous interaction session. There are two kinds of characteristic in the session: sequential dependencies and collective dependencies. Sequential dependencies mean that user-item interactions in the session are strictly ordered, while collective dependencies mean that multiple interactions with a flexible order jointly determine the user’s next behaviors. However, existing methods only take one of the two characteristics into account, which leads to the fact that they can’t model user behavior well. Furthermore, few methods emphasize the first interaction in the session, although user’s initial intention can be reflected by it to a large extent. To this end, we propose a novel model, Dual Part-pooling Attentive Networks for session-based Recommendation (DPAN4Rec), which is capable of capturing the sequential dependencies and collective dependencies of sessions simultaneously. By proposing a part-pooling attention mechanism, DPAN4Rec explores user’s initial intention from first click and filters out noisy clicks in the session. Extensive experiments have been conducted on two benchmark e-commerce datasets, Yoochoose and Diginetica, and the experimental results show that DPAN4Rec outperforms state-of-the-art methods. Furthermore, our study demonstrates that it is necessary to consider both sequential dependencies and collective dependencies in session-based recommendation.
更多查看译文
关键词
Session-based recommendation,Sequential dependencies,Collective dependencies,Part-pooling attention
AI 理解论文
溯源树
样例
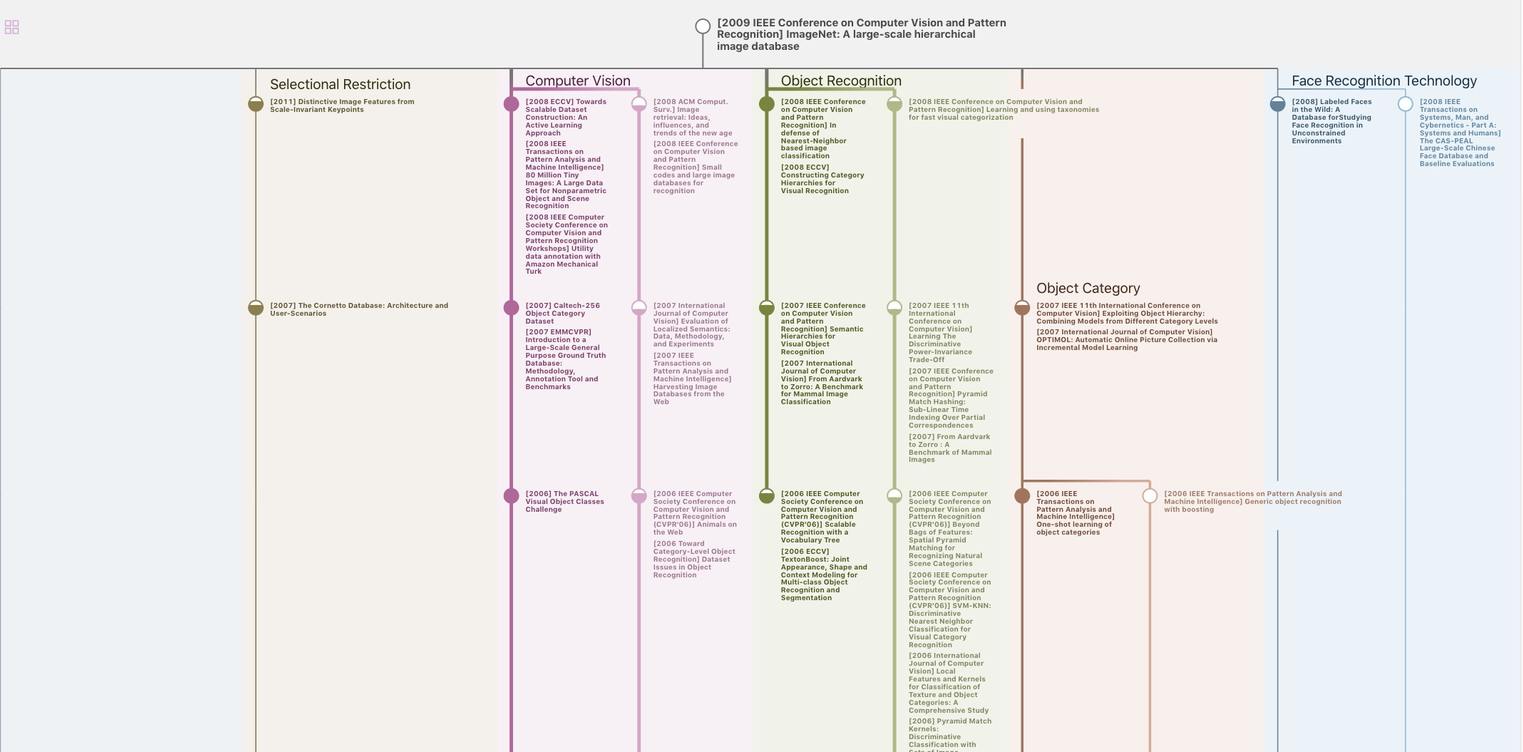
生成溯源树,研究论文发展脉络
Chat Paper
正在生成论文摘要