Two-layer neural networks with values in a Banach space
arxiv(2022)
摘要
We study two-layer neural networks whose domain and range are Banach spaces with separable preduals. In addition, we assume that the image space is equipped with a partial order, i.e. it is a Riesz space. As the nonlinearity we choose the lattice operation of taking the positive part; in case of $\mathbb R^d$-valued neural networks this corresponds to the ReLU activation function. We prove inverse and direct approximation theorems with Monte-Carlo rates for a certain class of functions, extending existing results for the finite-dimensional case. In the second part of the paper, we study, from the regularisation theory viewpoint, the problem of finding optimal representations of such functions via signed measures on a latent space from a finite number of noisy observations. We discuss regularity conditions known as source conditions and obtain convergence rates in a Bregman distance for the representing measure in the regime when both the noise level goes to zero and the number of samples goes to infinity at appropriate rates.
更多查看译文
关键词
 , vector-valued neural networks, ReLU, Barron space, variation norm space, curse of dimensionality, Bregman distance
AI 理解论文
溯源树
样例
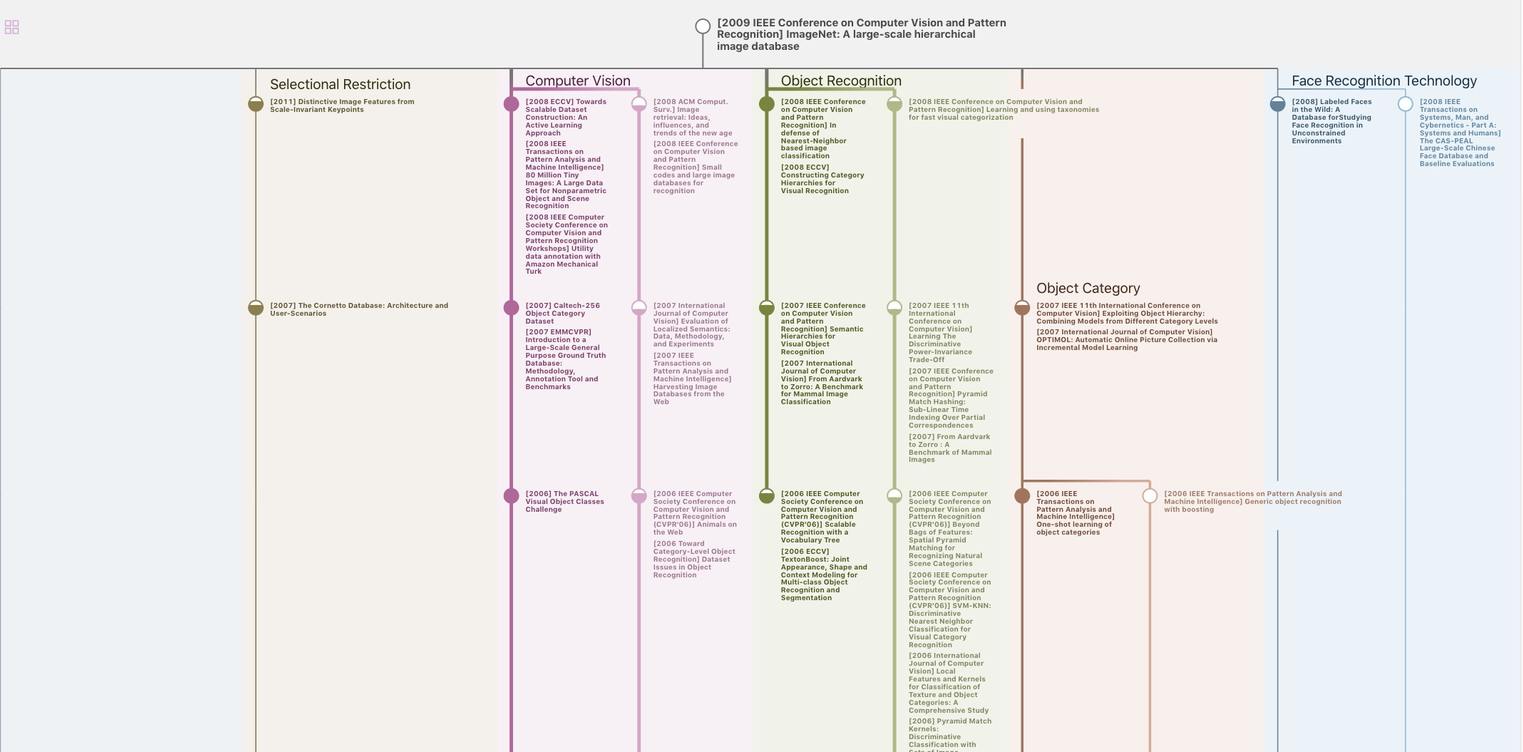
生成溯源树,研究论文发展脉络
Chat Paper
正在生成论文摘要