Promoting Global Stability In Data-Driven Models Of Quadratic Nonlinear Dynamics
PHYSICAL REVIEW FLUIDS(2021)
摘要
Modeling realistic fluid and plasma flows is computationally intensive, motivating the use of reduced-order models for a variety of scientific and engineering tasks. However, it is challenging to characterize, much less guarantee, the global stability (i.e., long-time boundedness) of these models. Previous work provided a theorem outlining necessary and sufficient conditions to ensure global stability in systems with energy-preserving, quadratic nonlinearities, with the goal of evaluating the stability of projection-based models. In this work, we incorporate this theorem into modern data-driven models obtained via machine learning. First, we propose that this theorem should be a standard diagnostic for the stability of projection-based and data-driven models, examining the conditions under which it holds. Second, we illustrate how to modify the objective function in machine learning algorithms to promote globally stable models, with implications for the modeling of fluid and plasma flows. Specifically, we introduce a modified "trapping SINDy" algorithm based on the sparse identification of nonlinear dynamics (SINDy) method. This method enables the identification of models that, by construction, only produce bounded trajectories. The effectiveness and accuracy of this approach are demonstrated on a broad set of examples of varying model complexity and physical origin, including the vortex shedding in the wake of a circular cylinder.
更多查看译文
关键词
quadratic nonlinear dynamics,global stability,models,data-driven
AI 理解论文
溯源树
样例
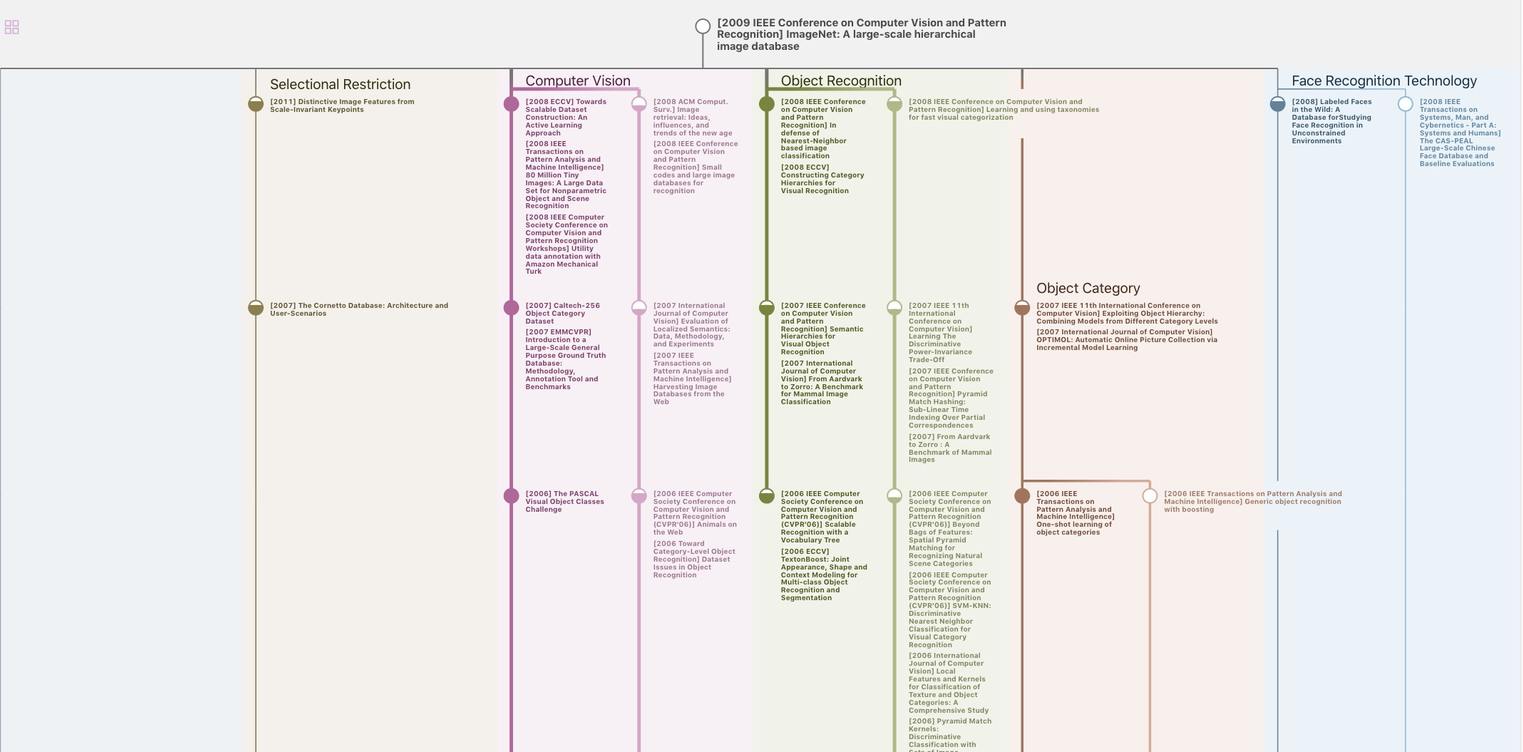
生成溯源树,研究论文发展脉络
Chat Paper
正在生成论文摘要