Improved calibration method for dynamic traffic assignment models: constrained extended Kalman filter
user-6078fb444c775e0497f4d030(2017)
摘要
The calibration of dynamic traffic assignment (DTA) models involves the estimation of model parameters to best replicate real-world measurements. Good calibration is essential to estimate and predict accurately traffic states, which are crucial for traffic management applications to alleviate congestion. A widely used approach to calibrate simulation-based DTA models is the extended Kalman filter (EKF). The EKF assumes that the DTA model parameters are unconstrained, although they are in fact constrained; for instance, origin–destination (O-D) flows are nonnegative. This assumption is typically not problematic for small- and medium-scale networks in which the EKF has been successfully applied. However, in large-scale networks (which typically contain numbers of O-D pairs with small magnitudes of flow), the estimates may severely violate constraints. In consequence, simply truncating the infeasible estimates may result in the divergence of EKF, leading to extremely poor state estimations and predictions. T...
更多查看译文
关键词
Extended Kalman filter,Divergence (statistics),Algorithm,Calibration,Replicate,Flow (mathematics),Computer science,State (functional analysis),Model parameters
AI 理解论文
溯源树
样例
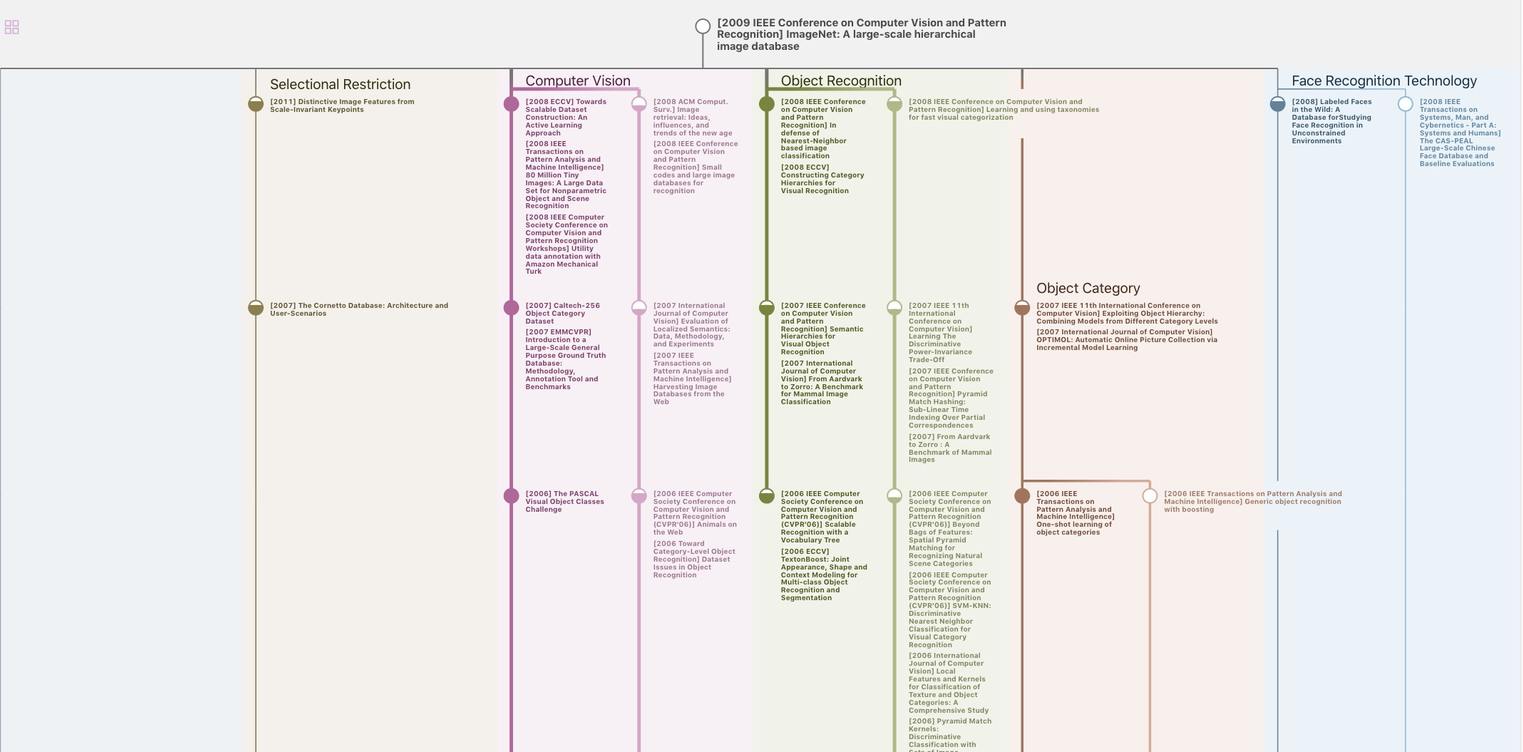
生成溯源树,研究论文发展脉络
Chat Paper
正在生成论文摘要