Enhancing the generalizability of predictive models with synergy of data and physics
MEASUREMENT SCIENCE AND TECHNOLOGY(2022)
摘要
Wind farms require predictive models for predictive maintenance. There is a need to predict values of non-observable parameters beyond ranges reflected in available data. A predictive model developed for one machine many not perform well for another similar machine. This is usually due to a lack of generalizability of data-driven models. To increase the generalizability of predictive models, this research integrates data mining with first-principle knowledge. Physics-based principles are combined with machine learning algorithms through feature engineering, strong rules and divide-and-conquer. The proposed synergy concept is illustrated with a wind turbine blade icing prediction and achieves significant predictive accuracy across different turbines. The proposed process should be widely accepted by wind energy predictive maintenance practitioners because of its simplicity and efficiency. Furthermore, the testing scores of KNN, CART and DNN algorithms are increased by 44.78%, 32.72% and 9.13%, respectively, with our proposed process. We demonstrate the importance of embedding physical principles within the machine learning process, and also highlight an important point that the need for more complex machine learning algorithms in industrial big data mining is often much less than it is in other applications, making it essential to incorporate physics and follow the 'less is more' philosophy.
更多查看译文
关键词
industrial big data, machine learning, process engineering, physical principle, predictive model, wind turbine blade icing
AI 理解论文
溯源树
样例
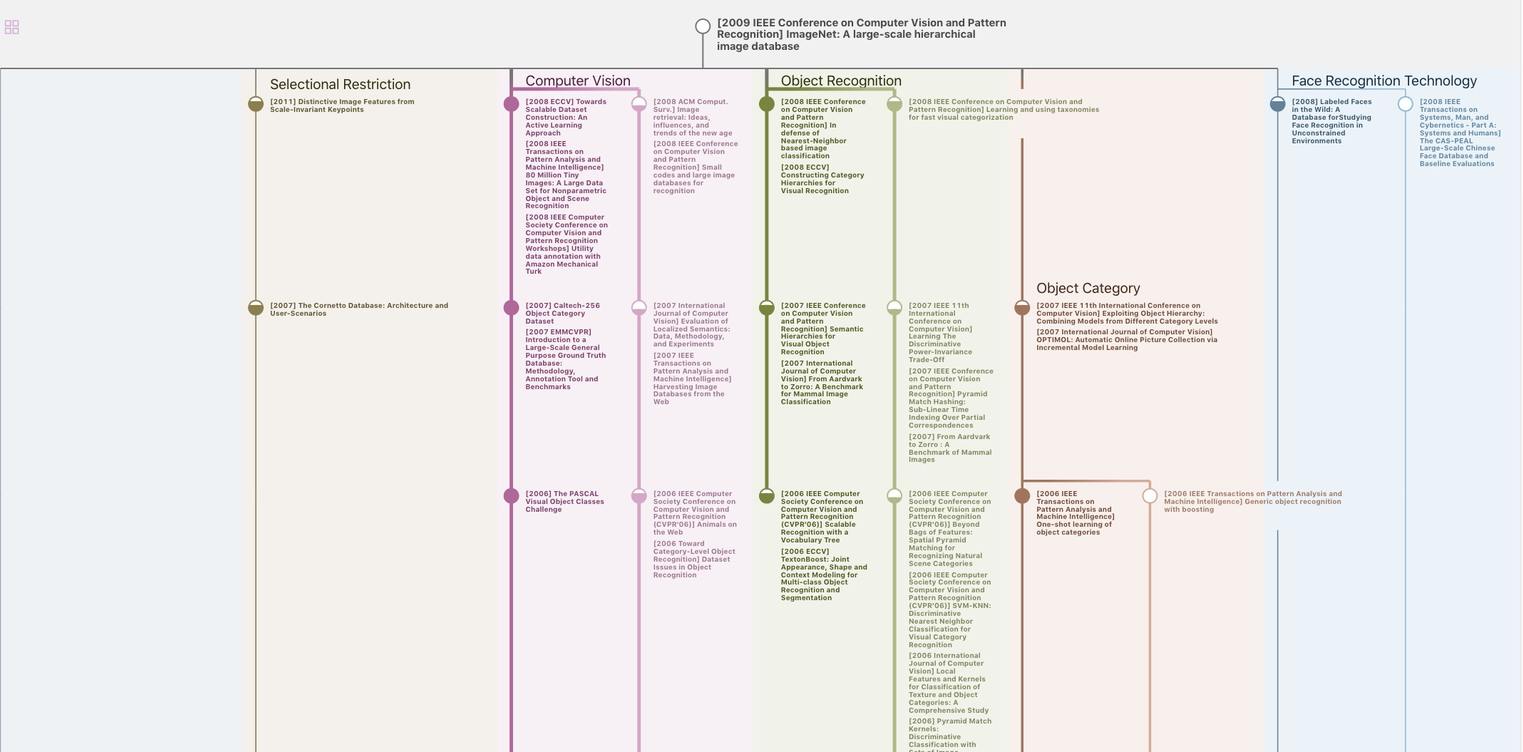
生成溯源树,研究论文发展脉络
Chat Paper
正在生成论文摘要